Discovering Adversarial Driving Maneuvers against Autonomous Vehicles
PROCEEDINGS OF THE 32ND USENIX SECURITY SYMPOSIUM(2023)
摘要
Over 33% of vehicles sold in 2021 had integrated autonomous driving (AD) systems. While many adversarial machine learning attacks have been studied against these systems, they all require an adversary to perform specific (and often unrealistic) actions, such as carefully modifying traffic signs or projecting malicious images, which may arouse suspicion if discovered. In this paper, we present ACERO, a robustness-guided framework to discover adversarial maneuver attacks against autonomous vehicles (AVs). These maneuvers look innocent to the outside observer but force the victim vehicle to violate safety rules for AVs, causing physical consequences, e.g., crashing with pedestrians and other vehicles. To optimally find adversarial driving maneuvers, we formalize seven safety requirements for AD systems and use this formalization to guide our search. We also formalize seven physical constraints that ensure the adversary does not place themselves in danger or violate traffic laws while conducting the attack. ACERO then leverages trajectory-similarity metrics to cluster successful attacks into unique groups, enabling AD developers to analyze the root cause of attacks and mitigate them. We evaluated ACERO on two open-source AD software, openpilot and Autoware, running on the CARLA simulator. ACERO discovered 219 attacks against openpilot and 122 attacks against Autoware. 73:3% of these attacks cause the victim to collide with a third-party vehicle, pedestrian, or static object.
更多查看译文
AI 理解论文
溯源树
样例
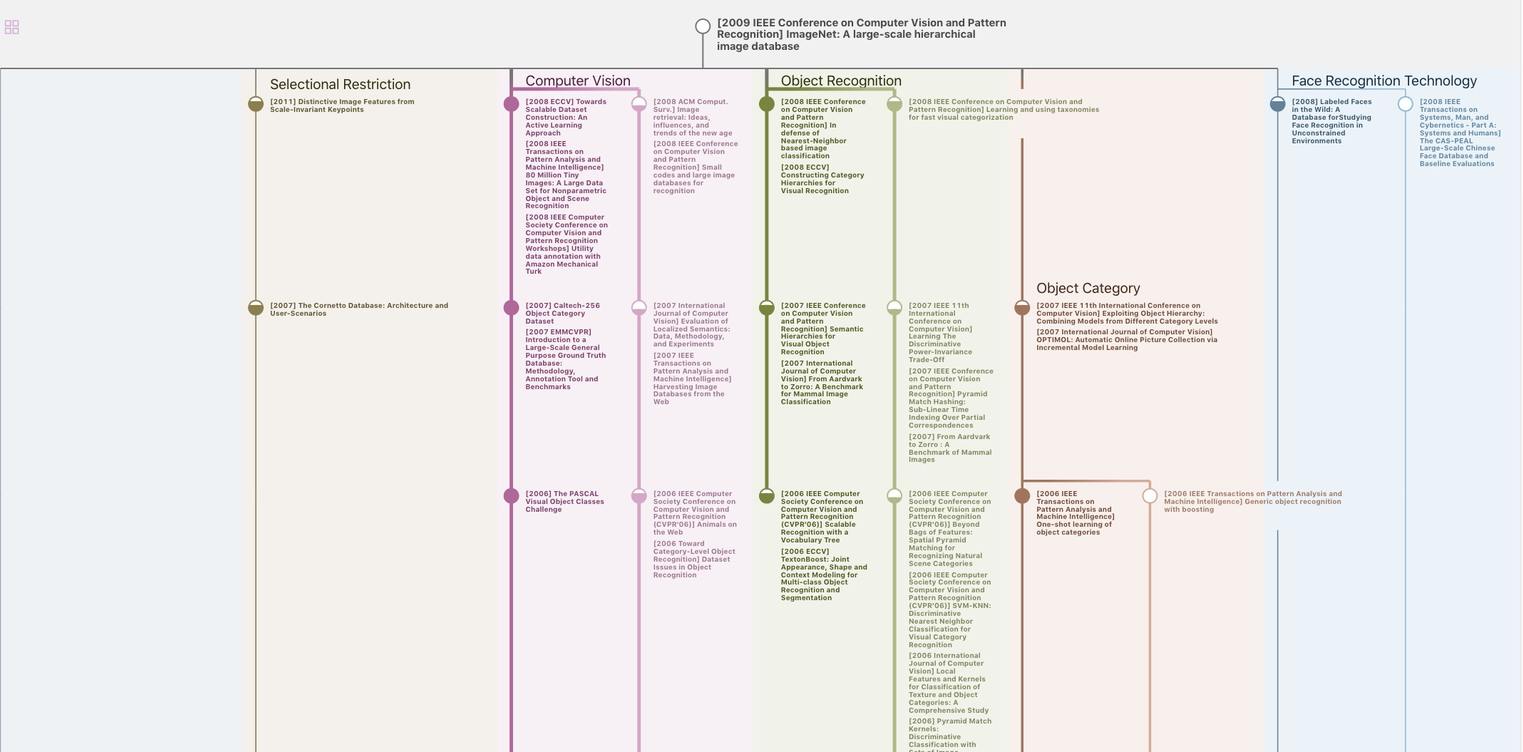
生成溯源树,研究论文发展脉络
Chat Paper
正在生成论文摘要