PASS2EDIT: A Multi-Step Generative Model for Guessing Edited Passwords
PROCEEDINGS OF THE 32ND USENIX SECURITY SYMPOSIUM(2023)
摘要
While password stuffing attacks (that exploit the direct password reuse behavior) have gained considerable attention, only a few studies have examined password tweaking attacks, where an attacker exploits users' indirect reuse behaviors (with edit operations like insertion, deletion, and substitution). For the first time, we model the password tweaking attack as a multi-class classification problem for characterizing users' password edit/modification processes, and propose a generative model coupled with the multi-step decision-making mechanism, called PASS2EDIT, to accurately characterize users' password reuse/modification behaviors. We demonstrate the effectiveness of PASS2EDIT through extensive experiments, which consist of 12 practical attack scenarios and employ 4.8 billion real-world passwords. The experimental results show that PASS2EDIT and its variant significantly improve over the prior art. More specifically, when the victim's password at site A (namely pwA) is known, within 100 guesses, the cracking success rate of PASS2EDIT in guessing her password at site B ( pw(B) not equal pw(A)) is 24.2% (for common users) and 11.7% (for security-savvy users), respectively, which is 18.2%-33.0% higher than its foremost counterparts. Our results highlight that password tweaking is a much more damaging threat to password security than expected.
更多查看译文
AI 理解论文
溯源树
样例
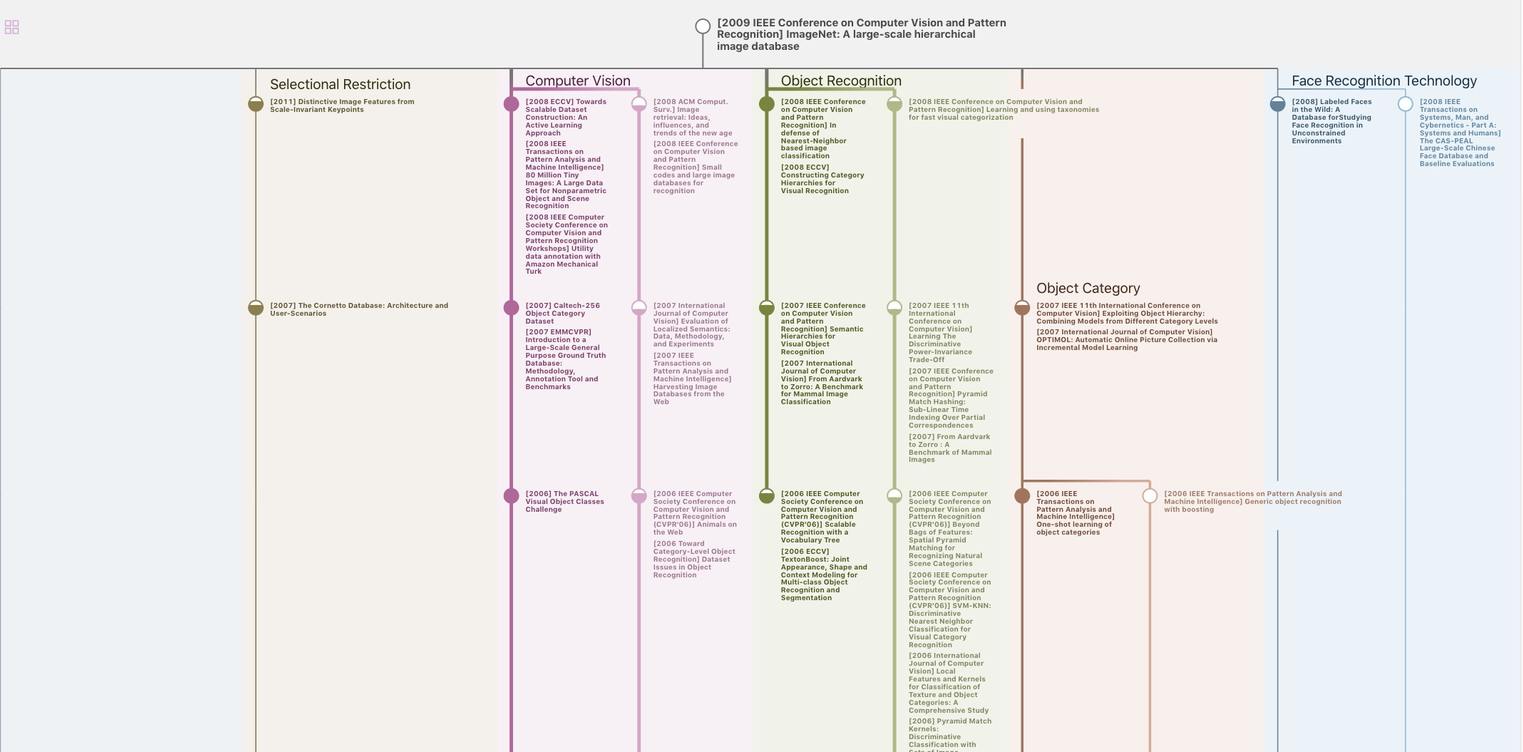
生成溯源树,研究论文发展脉络
Chat Paper
正在生成论文摘要