VILLAIN: Backdoor Attacks Against Vertical Split Learning
PROCEEDINGS OF THE 32ND USENIX SECURITY SYMPOSIUM(2023)
摘要
Vertical split learning is a new paradigm of federated learning for participants with vertically partitioned data. In this paper, we make the first attempt to explore the possibility of backdoor attacks by a malicious participant in vertical split learning. Different from conventional federated learning, vertical split learning poses new challenges for backdoor attacks, the most looming ones being a lack of access to the training data labels and the server model. To tackle these challenges, we propose VILLAIN, a backdoor attack framework that features effective label inference and data poisoning strategies. VILLAIN realizes high inference accuracy of the target label samples for the attacker. Furthermore, VILLAIN intensifies the backdoor attack power by designing a stealthy additive trigger and introducing backdoor augmentation strategies to impose a larger influence on the server model. Our extensive evaluations on 6 datasets with comprehensive vertical split learning models and aggregation methods confirm the effectiveness of VILLAIN. It is also demonstrated that VILLAIN can resist the popular privacy inference defenses, backdoor detection or removal defenses, and adaptive defenses.
更多查看译文
AI 理解论文
溯源树
样例
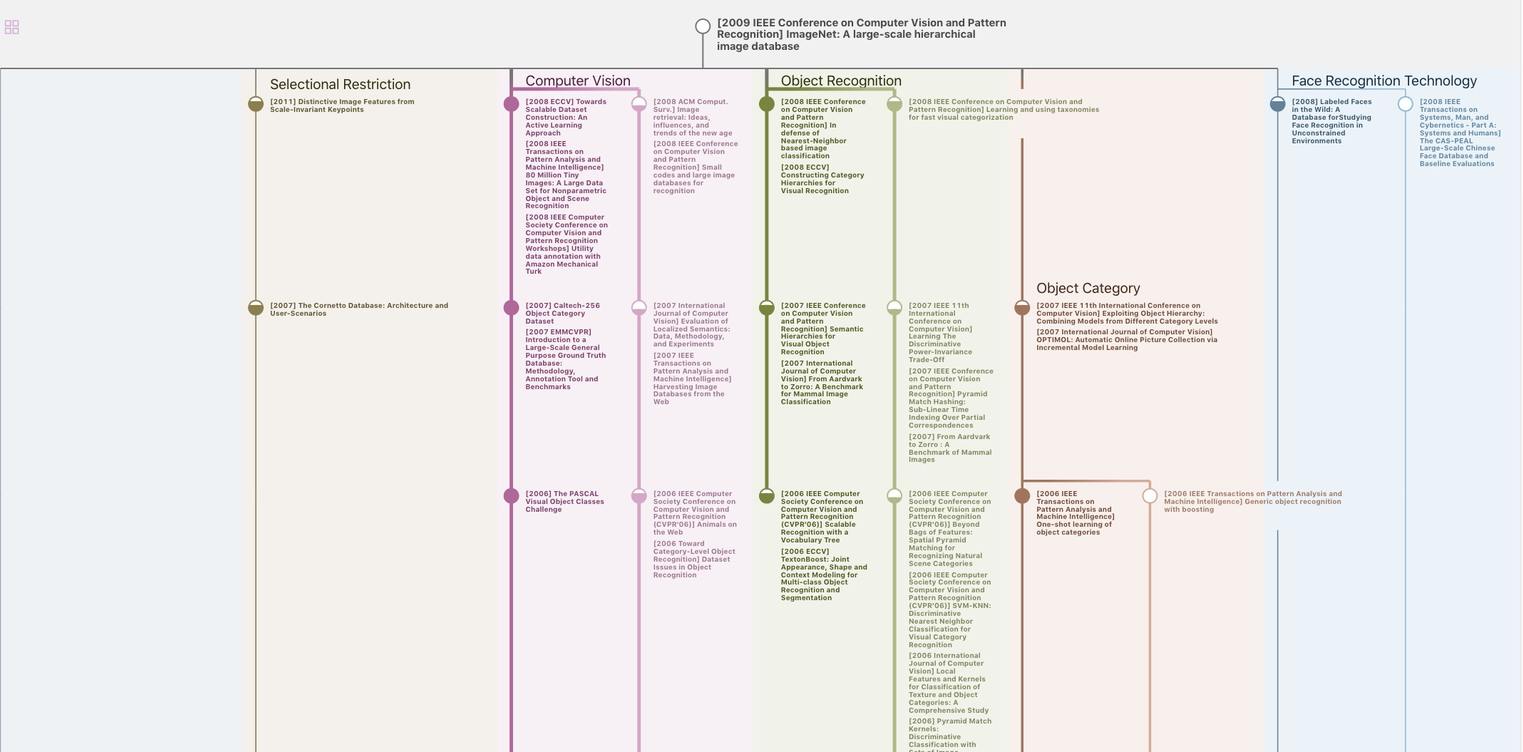
生成溯源树,研究论文发展脉络
Chat Paper
正在生成论文摘要