TBM-GAN: Synthetic Document Generation with Degraded Background.
ICDAR (2)(2023)
摘要
Deep document enhancement models often suffer in real world applications due to limited annotation and bias in training data. Moreover, generative models are often prone to spectral bias towards certain frequencies. The background (noisy) texture is usually harder to learn as it is composed from different frequency regions. In this work, we propose TBM-GAN, a generative adversarial network based framework to synthesise realistic handwritten documents with degraded background. In addition to the spatial information, TBM-GAN also incorporates the frequency information in its loss function to focus on complex noisy texture. Overall, we develop an automated pipeline for TBM-GAN and train it with artificially annotated data from publicly available resources. The pipeline provides both text-label and corresponding pixel-level annotation. We evaluate the quality of synthetic images in the downstream task of OCR. In text images with historical noisy background, we observe an 11 % reduction in the character error rate when the OCR is trained with synthetic data from TBM-GAN.
更多查看译文
关键词
synthetic document generation,degraded background,tbm-gan
AI 理解论文
溯源树
样例
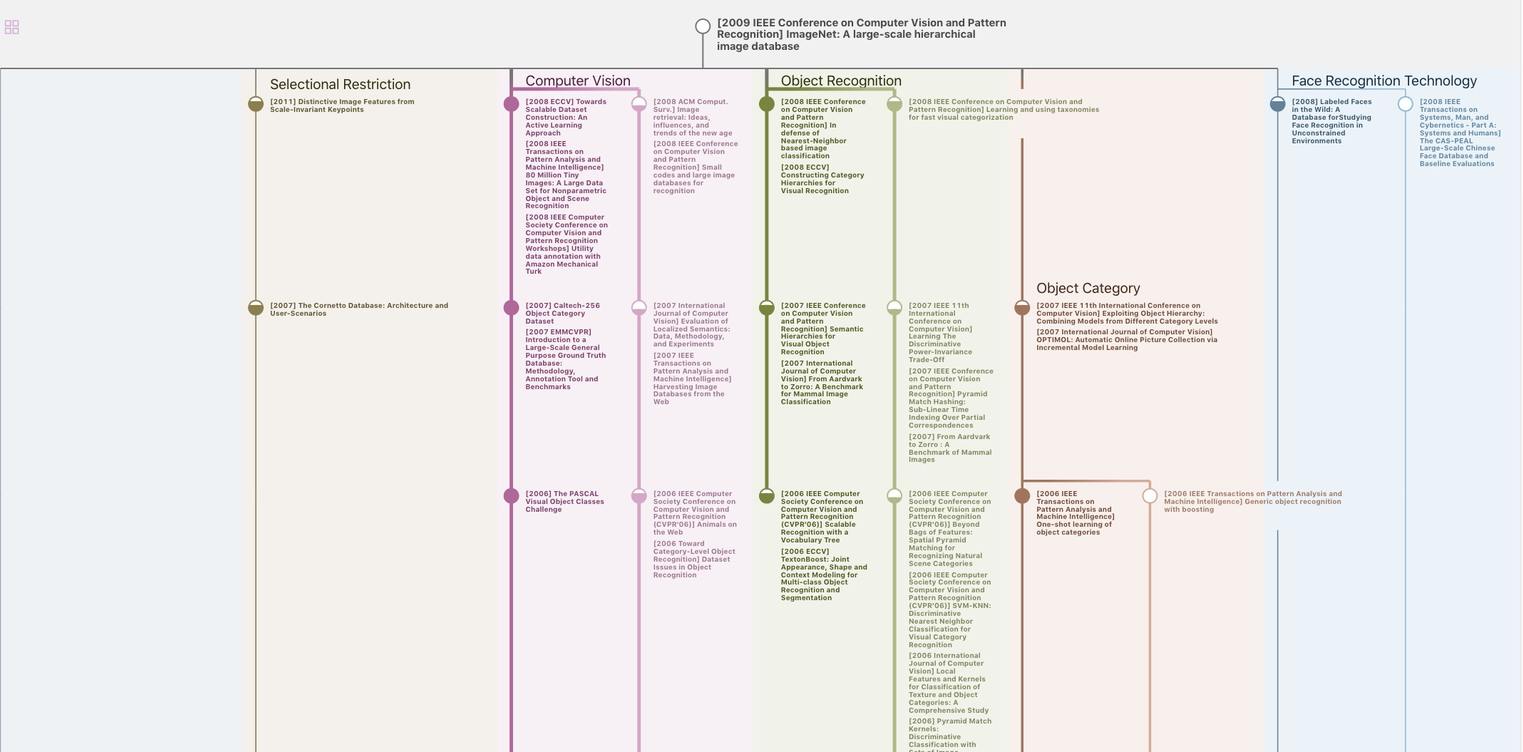
生成溯源树,研究论文发展脉络
Chat Paper
正在生成论文摘要