Predicting Adverse Childhood Experiences via Machine Learning Ensembles
PROCEEDINGS OF THE 16TH ACM INTERNATIONAL CONFERENCE ON PERVASIVE TECHNOLOGIES RELATED TO ASSISTIVE ENVIRONMENTS, PETRA 2023(2023)
摘要
Adverse Childhood Experiences (ACEs) have been linked to negative health outcomes later in life, including depression, anxiety, insomnia, and suicidal behavior. Recent studies have explored machine learning methods to classify individuals based on their ACE scores and predict their mental health outcomes. However, an extensive prediction of ACE via novel machine-learning ensembles based on several measures is yet to be undertaken. In this study, we used machine learning algorithms to classify individuals into high and low ACE groups and predict their mental health outcomes using various measures, including the Major Depressive Inventory, Generalized Anxiety Disorder, Insomnia Severity Index, World Health Organization Well-Being Index (WHO-5), suicide behavior, irrational decisions, self-harm, ability to focus, and suicidal thoughts. The study results showed that novel machine learning ensemble algorithms like a support-vector-decision tree ensemble and a support-vector-decision tree-random forest ensemble could accurately classify individuals into high and low ACE groups and predict their mental health outcomes. The study highlights the potential of using machine learning methods to identify individuals at high risk for mental health issues and provide targeted interventions to prevent the long-term negative consequences of ACEs.
更多查看译文
关键词
Adverse Childhood Experiences,Childhood trauma,Depression,Insomnia,Suicidal Behavior,Machine learning,Random Forest
AI 理解论文
溯源树
样例
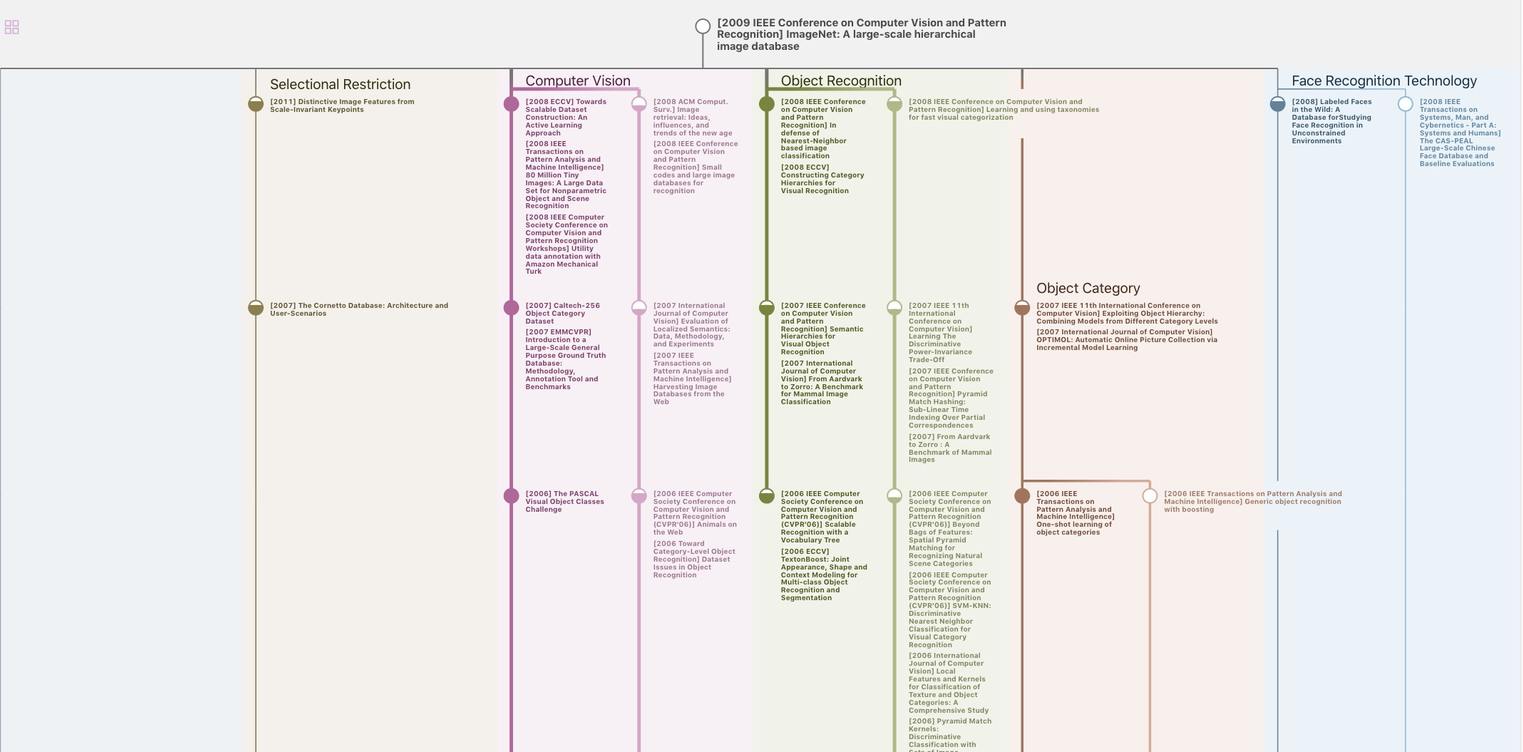
生成溯源树,研究论文发展脉络
Chat Paper
正在生成论文摘要