Optimizing the Performance of Text Classification Models by Improving the Isotropy of the Embeddings Using a Joint Loss Function.
ICDAR (5)(2023)
摘要
Recent studies show that the spatial distribution of the sentence representations generated from pre-trained language models is highly anisotropic. This results in a degradation in the performance of the models on the downstream task. Most methods improve the isotropy of the sentence embeddings by refining the corresponding contextual word representations, then deriving the sentence embeddings from these refined representations. In this study, we propose to improve the quality of the sentence embeddings extracted from the [CLS] token of the pre-trained language models by improving the isotropy of the embeddings. We add one feed-forward layer between the model and the downstream task layers, and we train it using a novel joint loss function. The proposed approach results in embeddings with better isotropy, that generalize better on the downstream task. Experimental results on 3 GLUE datasets with classification as the downstream task show that our proposed method is on par with the state-of-the-art, as it achieves performance gains of around 2–3% on the downstream tasks compared to the baseline.
更多查看译文
关键词
text classification models,text classification,embeddings,joint loss function
AI 理解论文
溯源树
样例
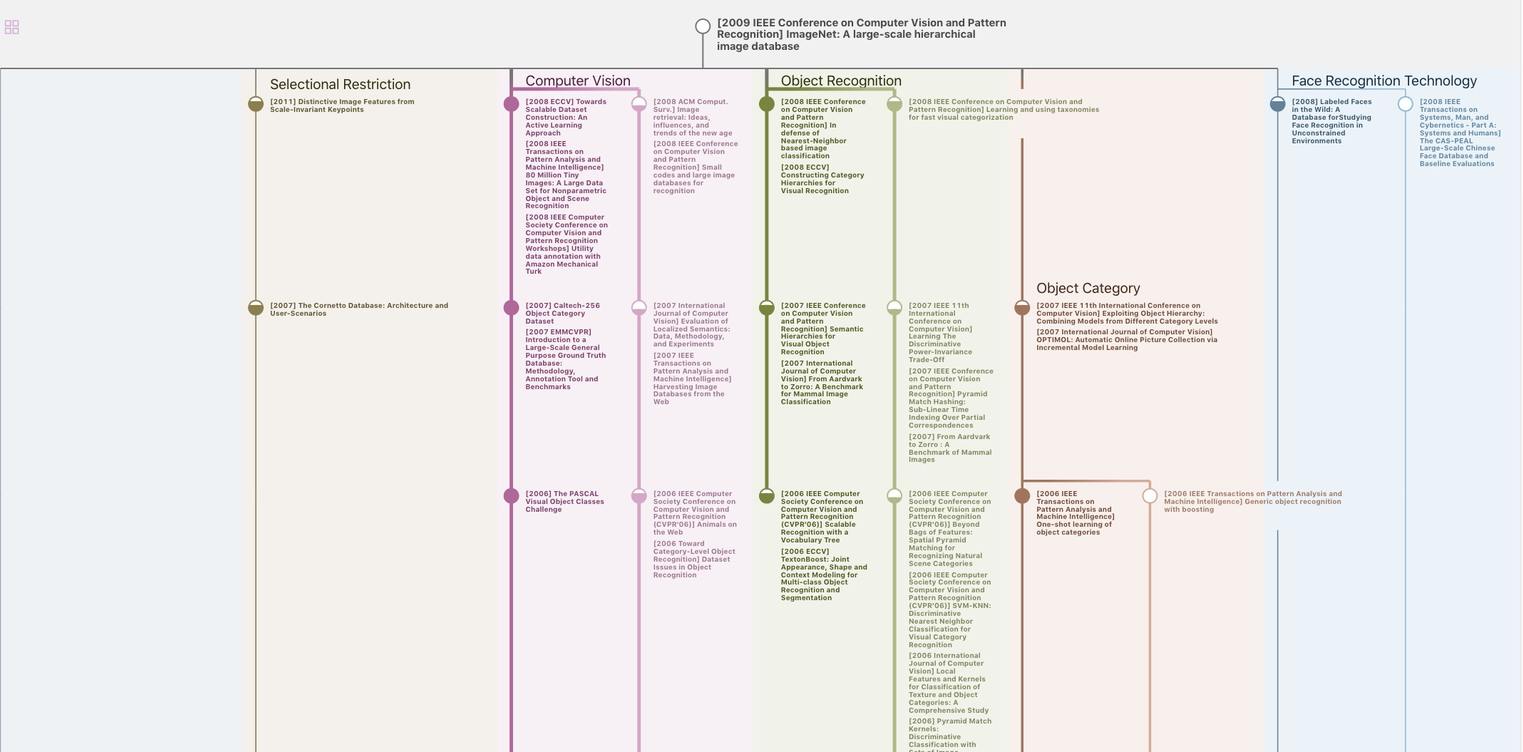
生成溯源树,研究论文发展脉络
Chat Paper
正在生成论文摘要