Data-Driven Similarity-based Worker Recruitment Towards Multi-task Data Inference for Sparse Mobile Crowdsensing.
IWQoS(2023)
摘要
Sparse Mobile Crowdsensing is an emerging paradigm for data collection with budgets and workers' limitations' which recruits workers to sense a part of spatio-temporal data and infer what is unsensed. In order to achieve high inferring accuracy in all spatio-temporal areas, we need to measure the importance level of each area and sense some important ones. Existing works usually use the average distance or the difficulty level inferred by historical data to measure the area's importance. However, we argue that neither distance nor difficulty level is suitable for measuring the importance. A better approach is inspired by the data itself, i.e., data similarity among different areas. Furthermore, there usually exist multiple data types in sparse mobile crowdsensing, which leads to a more complex inference from two-dimensional data (spatial and temporal) to three-dimensional data (spatial, temporal, and data type). In this paper, we study worker recruitment in a multi-task scenario, which aims to recruit workers to sense important data for a three-dimensional inference. Specifically, we first present the SWDTW method to calculate data similarity, which is used to evaluate data importance. Based on this, we further propose an evaluation model for three-dimensional data similarity and measure the importance of each area. Finally, inspired by generalized greedy and simulated annealing, we propose a worker recruitment method named WRGSA, the target of which is selecting workers to sense important areas to enhance the inference accuracy. Extensive experiments have been conducted over three real-world datasets with multiple data types, which can verify the effectiveness of our proposed methods.
更多查看译文
关键词
Sparse MCS,Similarity,Multiple data type,Worker recruitment
AI 理解论文
溯源树
样例
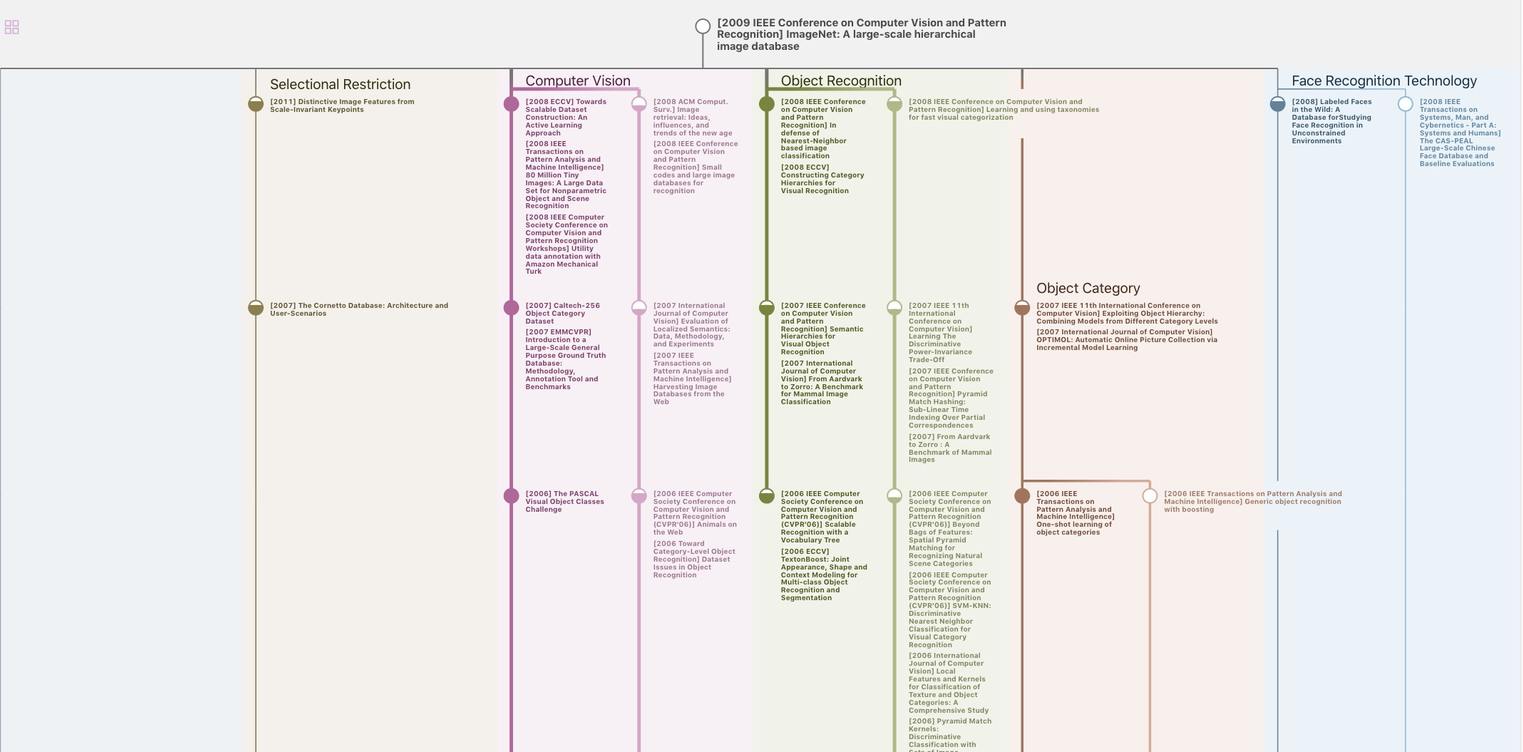
生成溯源树,研究论文发展脉络
Chat Paper
正在生成论文摘要