Automatic Image Compositing and Snow Segmentation for Alpine Snow Cover Monitoring
SDS(2023)
摘要
Accurate snow cover maps are an important tool in a large variety of applications including avalanche research. The WSL Institute for Snow and Avalanche Research (SLF) has developed a snow cover mapping system for the Dischma valley in Davos, based on ground-based cameras. Their goal has been to validate snow-cover maps derived from satellite data. In the currently implemented system, several steps require manual work. The goal of this paper is to automate SLF’s approach by applying a deep learning framework. For the training of our models, we have access to data from multiple cameras mounted in the Dischma valley. In our approach, we first apply deep learning to perform fog classification and then to do pixel-wise snow segmentation. Unlike the current procedure our method is independent of image- and camera-specific thresholds. In our experiments, we compare a model that is trained on images from all cameras to camera-specific models for both tasks. For the fog classification, we aim for a higher recall to be able to detect most of the foggy images. We show that, when tuned to achieve the same precision as the baseline, our model improves recall by 17%. For the snow segmentation, we evaluate our models based on the Fl-score. When using our fully automated machine learning model without any manually selected thresholds, we achieve a higher Fl-score only for a part of the cameras in the valley, and on average, this comes at a cost of 2.3%. We also show that for the case of a new camera being mounted in the Dischma valley, our models for both tasks can still be used for that new camera and will achieve similar results compared to the currently mounted cameras.
更多查看译文
关键词
Avalanche Research, Computer Vision, Classification, Deep Learning, Remote Sensing, Segmentation
AI 理解论文
溯源树
样例
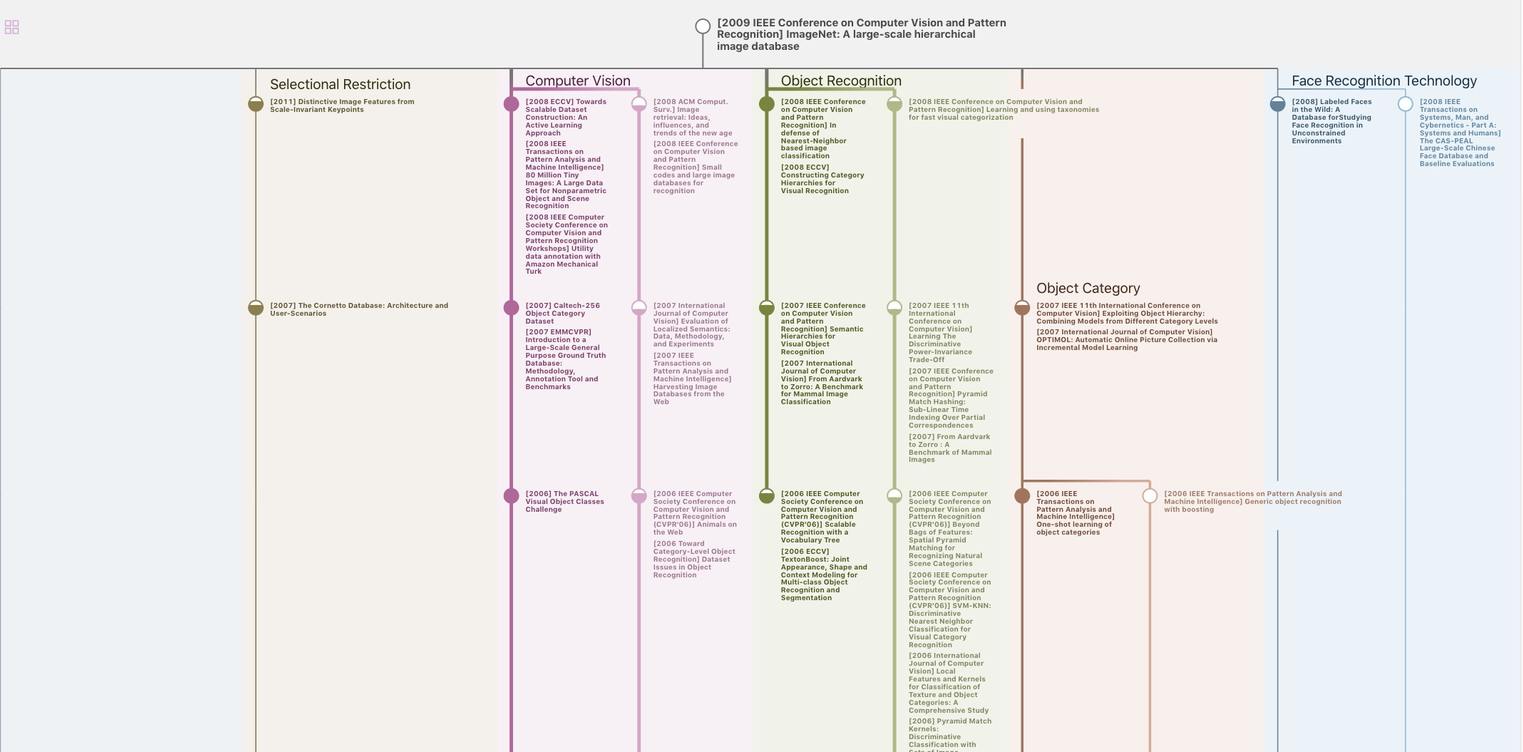
生成溯源树,研究论文发展脉络
Chat Paper
正在生成论文摘要