State-Space Modeling and Tuning of Memristors for Neuromorphic Computing Applications.
ICONS(2023)
摘要
Analog memristive devices have the potential to merge computing and memory, support local learning, reach high densities, enable 3D stacking, and low energy consumption for neuromorphic computing applications. Yet, integration is challenged by the variability and complex nonlinear dynamics involved in the tuning of memristors, which is required in computing and memory applications. In this paper, we model the dynamic analog switching of memristive devices with an evolution-measurement state-space model. A physics-based compact model is extended to capture statistical distributions of the variability observed in memristors. Based on metal-oxide memristors and electronic measurement data, we applied Sequential-Monte Carlo (Particle Filter) techniques to infer underlying memristor model parameters. The result is validated by experimental data. Applying the calibrated statistical model, we propose an efficient adaptive pulse programming scheme, and performed a comparative analysis across widely applied write-and-verify techniques. We show improved programming control in the metrics of error, energy, and time in reaching target states.
更多查看译文
AI 理解论文
溯源树
样例
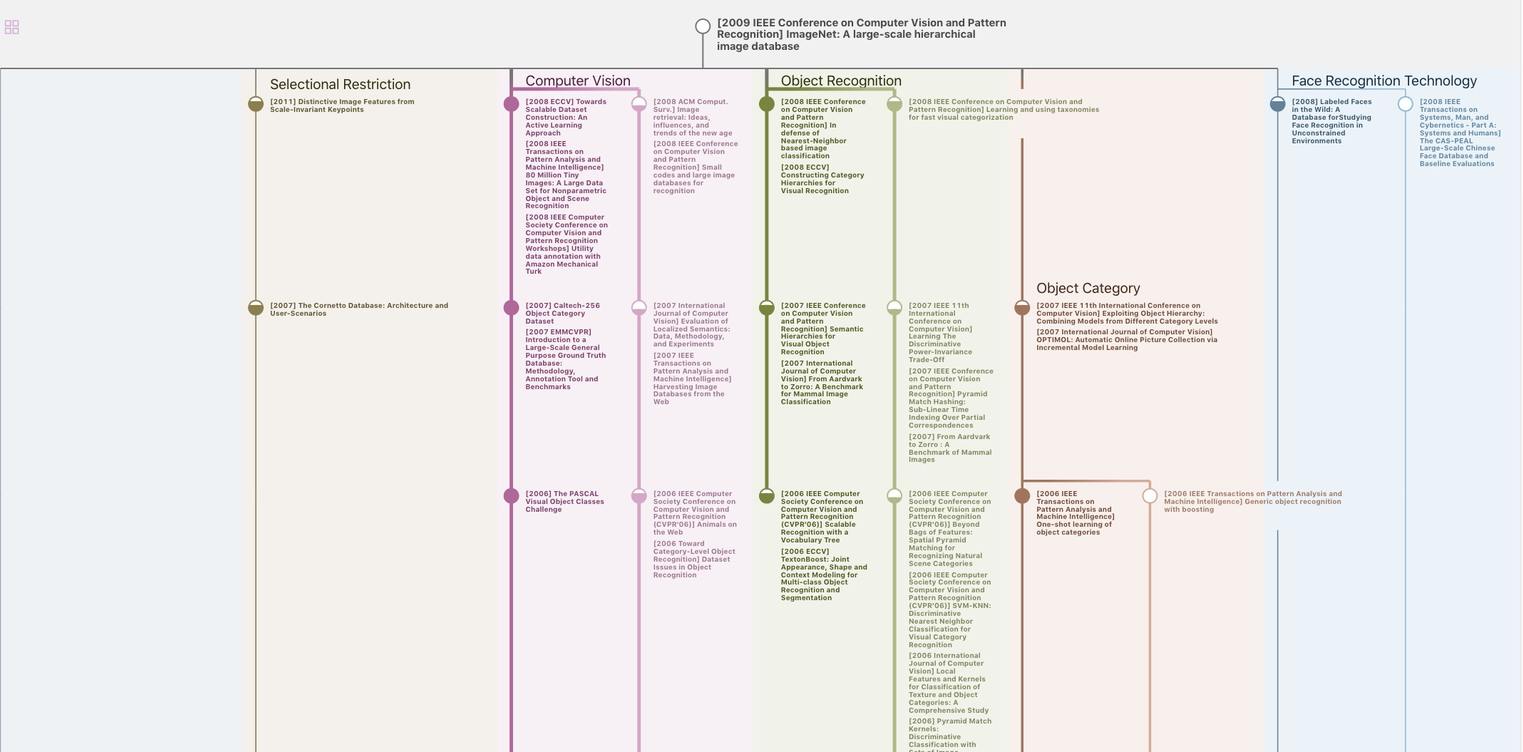
生成溯源树,研究论文发展脉络
Chat Paper
正在生成论文摘要