Deduplication of Textual Data by NLP Approaches
VTC2023-Spring(2023)
摘要
With the increasing amount of digital data, data deduplication has become an increasingly popular method for reducing data in large-scale storage systems. Generalized deduplication is an alternative technique for reducing the cost of data storage by identifying similar data chunks. This paper proposes TL-GD, a method for improving cloud storage efficiency using generalized deduplication focusing on textual datasets. The core concept of this study is to develop an efficient deduplication system that combines an alternative technique for splitting data into smaller pieces and a new approach for transforming data pieces into bases and deviations. The performance of the system has been validated using two real-world datasets. We also compare the results to state-of-the-art deduplication methods. Our evaluation results show that TL-GD achieves nearly 67% lossless compression for textual navigation instructions datasets, which is a 25% improvement on average compared to existing deduplication techniques.
更多查看译文
关键词
CSP,Storage,Generalized Deduplication
AI 理解论文
溯源树
样例
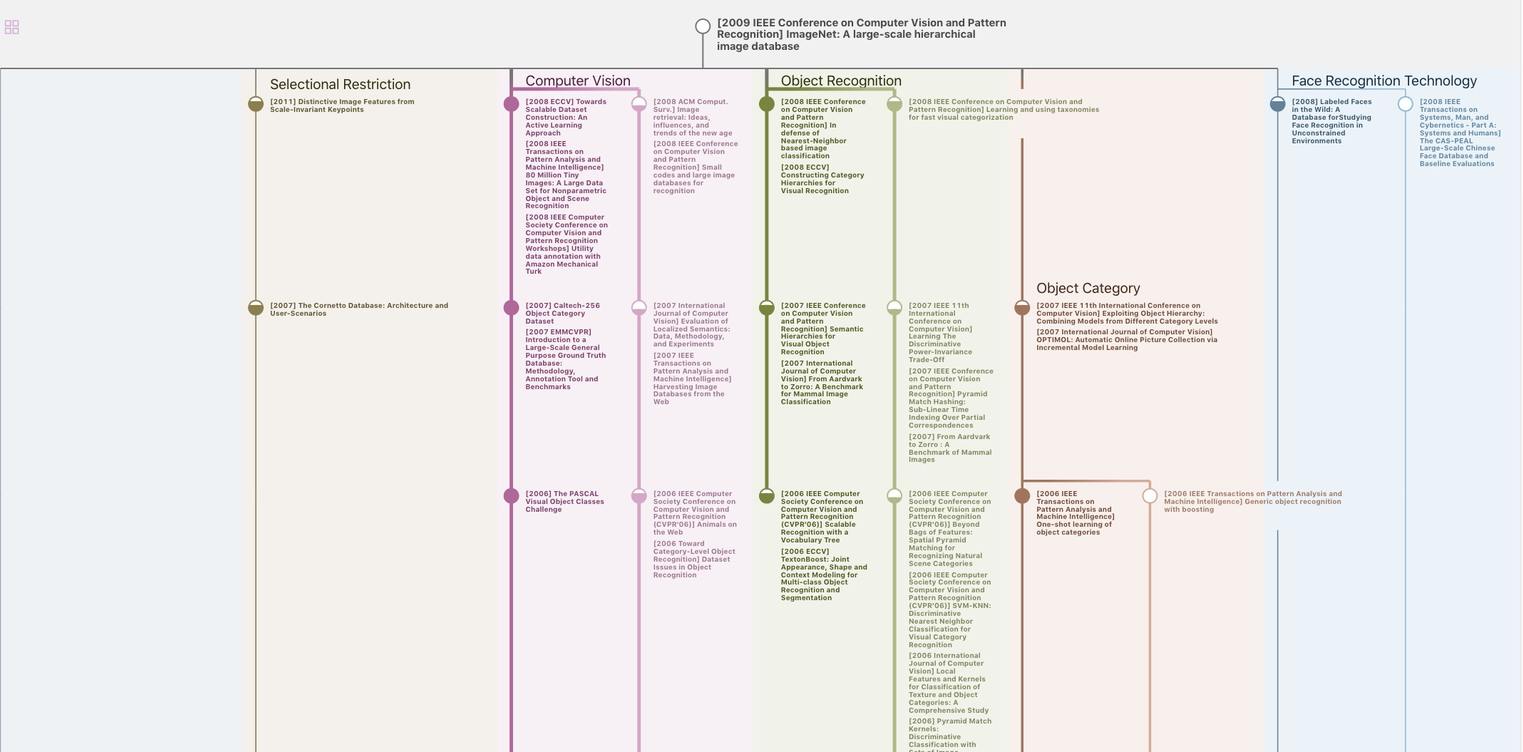
生成溯源树,研究论文发展脉络
Chat Paper
正在生成论文摘要