Adversarial Machine Learning Attacks on Multiclass Classification of IoT Network Traffic
18TH INTERNATIONAL CONFERENCE ON AVAILABILITY, RELIABILITY & SECURITY, ARES 2023(2023)
摘要
Machine Learning-based Intrusion Detection Systems have been proven to be very effective in the protection of IoT Networks. How-ever, the expansion of Adversarial Machine Learning attacks threat-ens their effcacy affecting also the security of IoT networks. Thus, this paper proposes a Machine Learning-driven methodology for multiclass classification of cyber-attacks in IoT networks and inves-tigates the robustness of the Machine and Deep Learning classifiers against several well-known Adversarial Machine Learning attacks (JSMA, FGSM, DeepFool). Moreover, the effectiveness of the Ad-versarial Training defense method has been studied in tackling Adversarial Machine Learning attacks. The proposed methodol-ogy was evaluated using a new and large IoT dataset (IoTID20) and the experimental results concluded that the Random Forest classifier can classify the cyber-attacks with high classification accu-racy (99.9%) as well as the JSMA, FGSM, and DeepFool attacks can significantly reduce the performance of all the classifiers. Finally, based on the evaluation adversarial training can overall enhance the classifiers' robustness against all the utilized Adversarial Ma-chine Learning attacks without affecting the performance when only normal samples are present.
更多查看译文
关键词
IoT Cyber-Attacks Detection,Machine Learning,Adversarial Ma-chine Learning,Adversarial Training
AI 理解论文
溯源树
样例
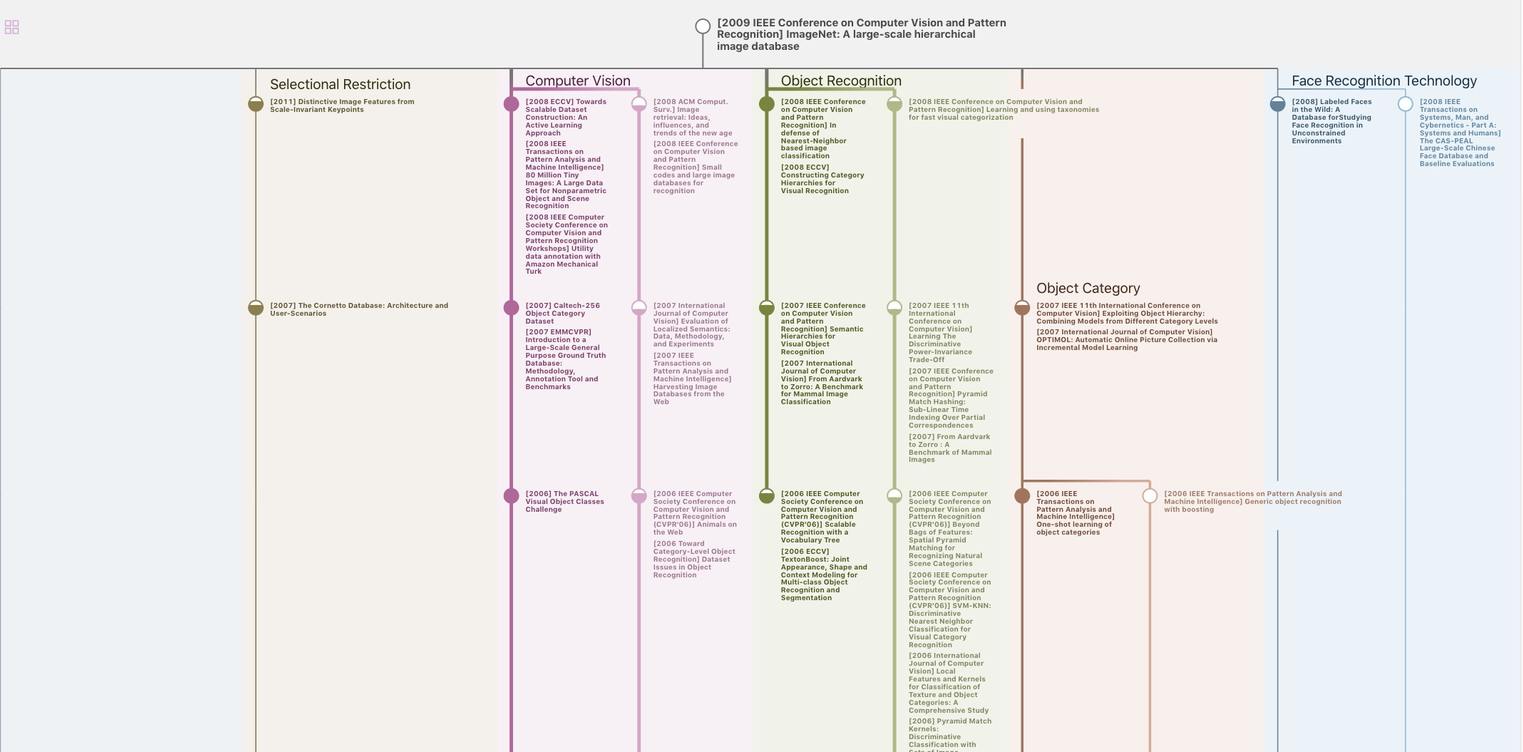
生成溯源树,研究论文发展脉络
Chat Paper
正在生成论文摘要