Dealing with Over-Reliance on Background Graph for Few-Shot Knowledge Graph Completion.
KSEM (1)(2023)
摘要
Few-shot knowledge graph completion (FKGC) has drawn growing research attention recently, aiming at inferring new triples using only a small number of related references. Most existing FKGC methods encode few-shot relations by capturing signals from local neighbors of each entity. However, this process dramatically relies on a thorough background knowledge graph (background KG) that is space-consuming to storage and often inaccessible in real-life cases. Moreover, they tend to overlook the underlying correlational information coming with different relations. In this paper, we attempt to address FKGC task in a practical scenario where background KG is not provided and propose a novel framework called ICOM ( I Can C ount O n M yself) which aims at making full use of few-shot instances within and across relations. Specifically, we go deep into the interaction between entity pairs within specific relation and devise a 3D convolutional meta-relation learner to extract relation-specific features straightly from triple. To accumulate knowledge across relations, an analogical enhancer is then built to leverage the semantic relational correlations attentively so that correlative relations can complement each other on representation learning for better link prediction. Finally, we introduce meta-learning technique for faster adaption. Empirical studies on three real-world FKGC datasets demonstrate that ICOM shows superiority over competitive baseline methods and achieves new state-of-the-art results with different few-shot sizes.
更多查看译文
关键词
background graph,knowledge,over-reliance,few-shot
AI 理解论文
溯源树
样例
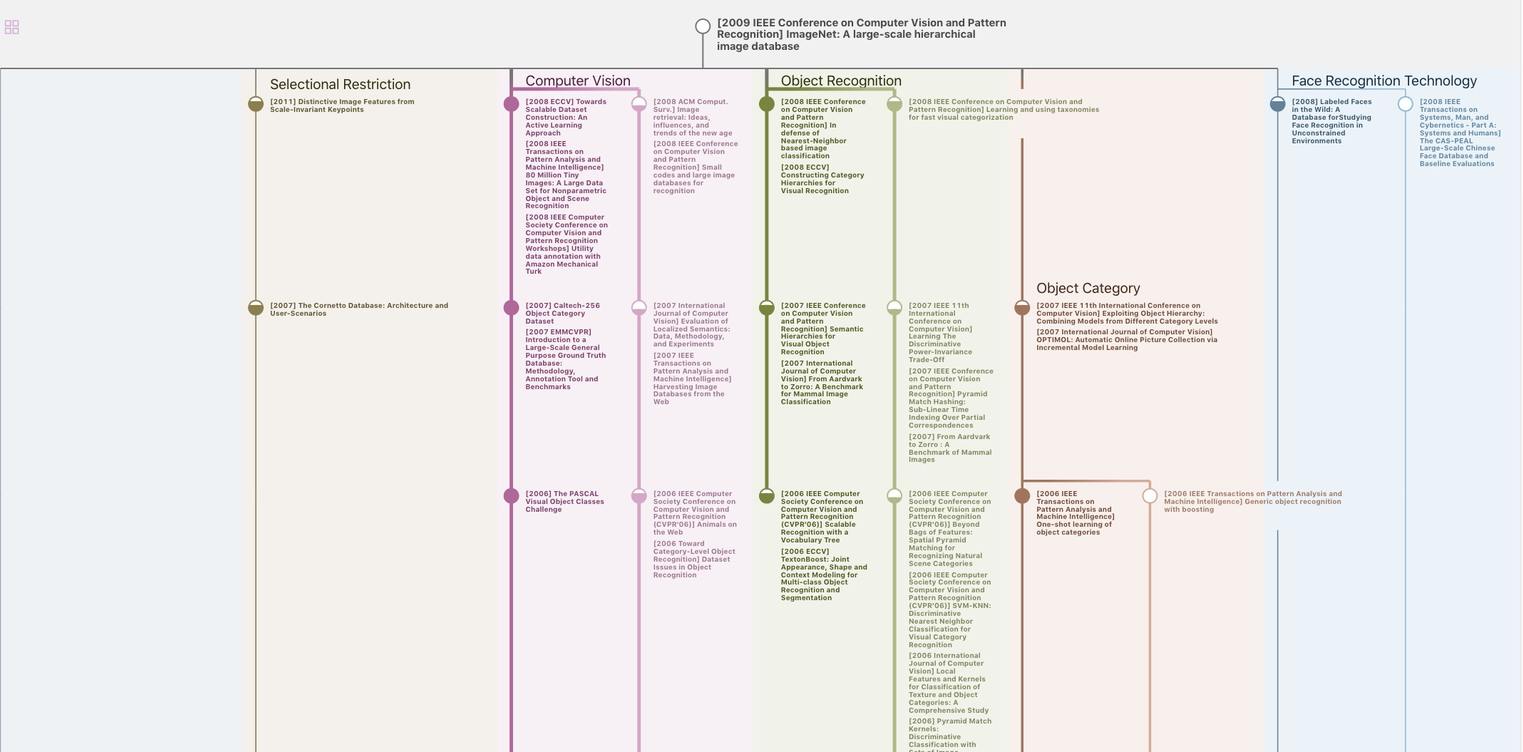
生成溯源树,研究论文发展脉络
Chat Paper
正在生成论文摘要