Robust Few-Shot Graph Anomaly Detection via Graph Coarsening.
KSEM (1)(2023)
摘要
Graph anomaly detection is an important aspect of anomaly detection, especially the graph-level anomaly detection, which can be used into biomolecular research or drug molecular detection and so on. It is necessary to identify anomalies within a limited sample size as the real-world scenarios always lack of anomalous graph labels. Graph-level anomaly detection with few samples mainly faces the following problems: 1) There are not enough samples for the model to effectively learn anomalies; 2) There is noise or irrelevant information in the graph, which makes it difficult to quickly learn the key structural information of the graph. To address these issues, we propose a Robust Meta-learning-based Graph Anomaly Detection Framework via Graph Coarsening (RCM-GAD). Specifically, we employ meta-learning to effectively extract and integrate abnormal information from similar networks. Then, we use the Graph Coarsening module to obtain the key structural information of the graph for anomaly detection. We apply this framework to detect anomalies at both the graph-level and subgraph-level. We conduct experiments on four datasets, demonstrating the superiority of our proposed framework, RCM-GAD, over state-of-the-art baselines in graph-level and subgraph-level anomaly detection tasks.
更多查看译文
关键词
detection,few-shot
AI 理解论文
溯源树
样例
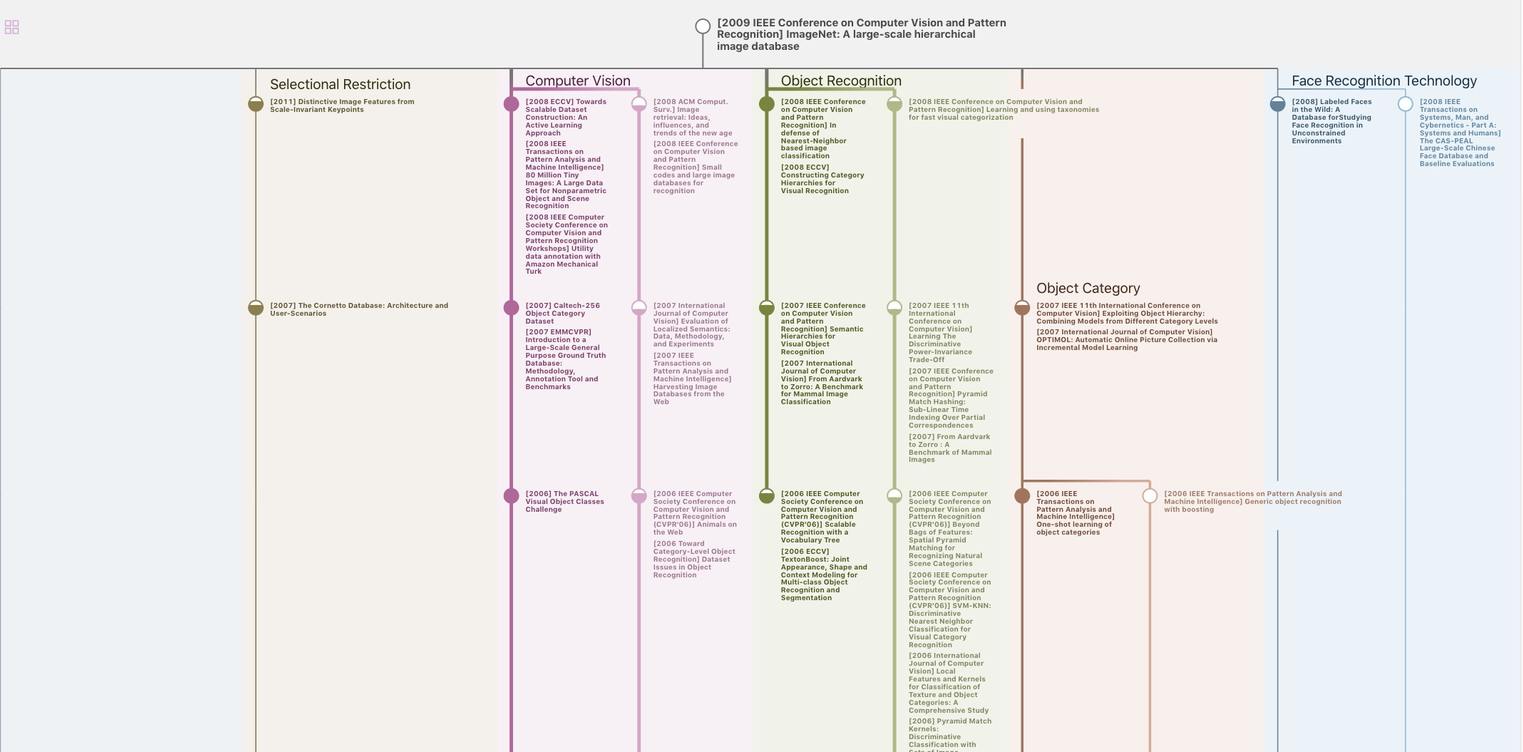
生成溯源树,研究论文发展脉络
Chat Paper
正在生成论文摘要