MA-TGNN: Multiple Aggregators Graph-Based Model for Text Classification.
KSEM (3)(2023)
摘要
In recent years, graph neural network (GNN) has performed well in processing non-Euclidean structural data and saving global co-occurrence information. Researchers are exploring the application of GNN in the field of text classification. However, some existing GNN-based methods employ corpus-level graph structures, which can result in high memory consumption. Additionally, a single-node aggregation method may only partially extract semantic features. We propose a graph-based text classification model called the Multi-Aggregator GNN model to address these limitations. Specifically, we utilize multiple aggregation methods to obtain the distributional characteristics of the text comprehensively. And we incorporate dimensionality reduction pooling to preserve crucial information in the text representation. Finally, we use the updated node representations as document embeddings. Experimental results on seven benchmark datasets demonstrate that our proposed model significantly improves the performance of text classification tasks.
更多查看译文
关键词
classification,ma-tgnn,graph-based
AI 理解论文
溯源树
样例
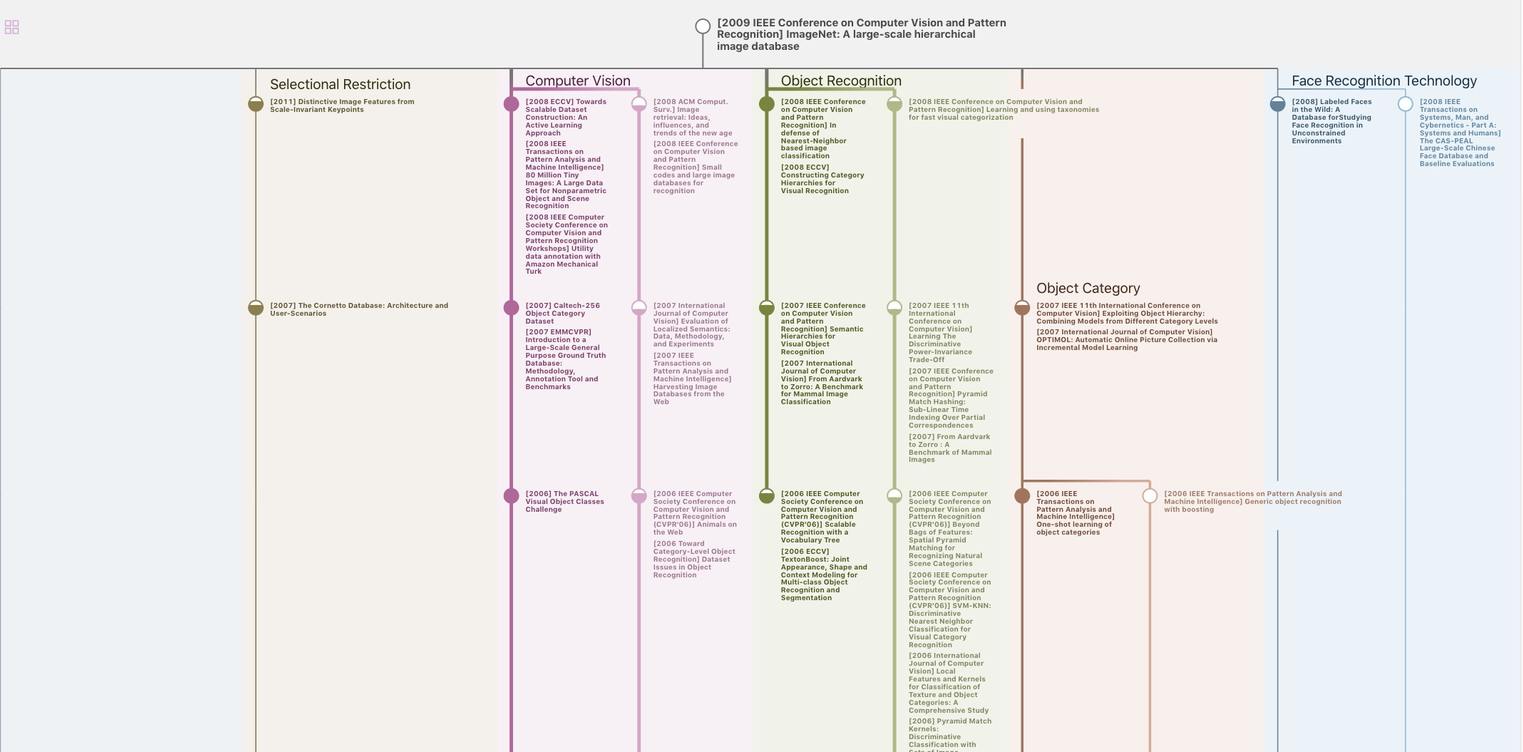
生成溯源树,研究论文发展脉络
Chat Paper
正在生成论文摘要