Hyperplane Knowledge Graph Embedding with Path Neighborhoods and Mapping Properties.
KSEM (1)(2023)
摘要
Knowledge representation learning(KRL) is significant for the knowledge graph completion(or link prediction) task, it aims to project entities and relations to a low-dimensional vector space. There are two topics have been widely studied in KRL: one is the ability of the model to handle complex relations(i.e., N-to-1, 1-to-N and N-to-N), and the other is whether the model integrates multi-source information. However, the existing methods rarely consider both topics. To mitigate this problem, this paper proposes TransPMH, a hyperplane knowledge graph embedding model with path neighborhoods and mapping properties, which models the relation as a hyperplane. Besides, this paper introduces path neighborhoods as the multi-source information to improve the model’s knowledge representation ability, and also introduces the relational mapping properties to enhance the model’s ability to handle complex relations. We conducted extensive experiments for link prediction and triplet classification on benchmark datasets like WordNet and Freebase. Experimental results show that the proposed method achieves significant improvements in multiple evaluation metrics.
更多查看译文
关键词
path neighborhoods,knowledge,mapping properties
AI 理解论文
溯源树
样例
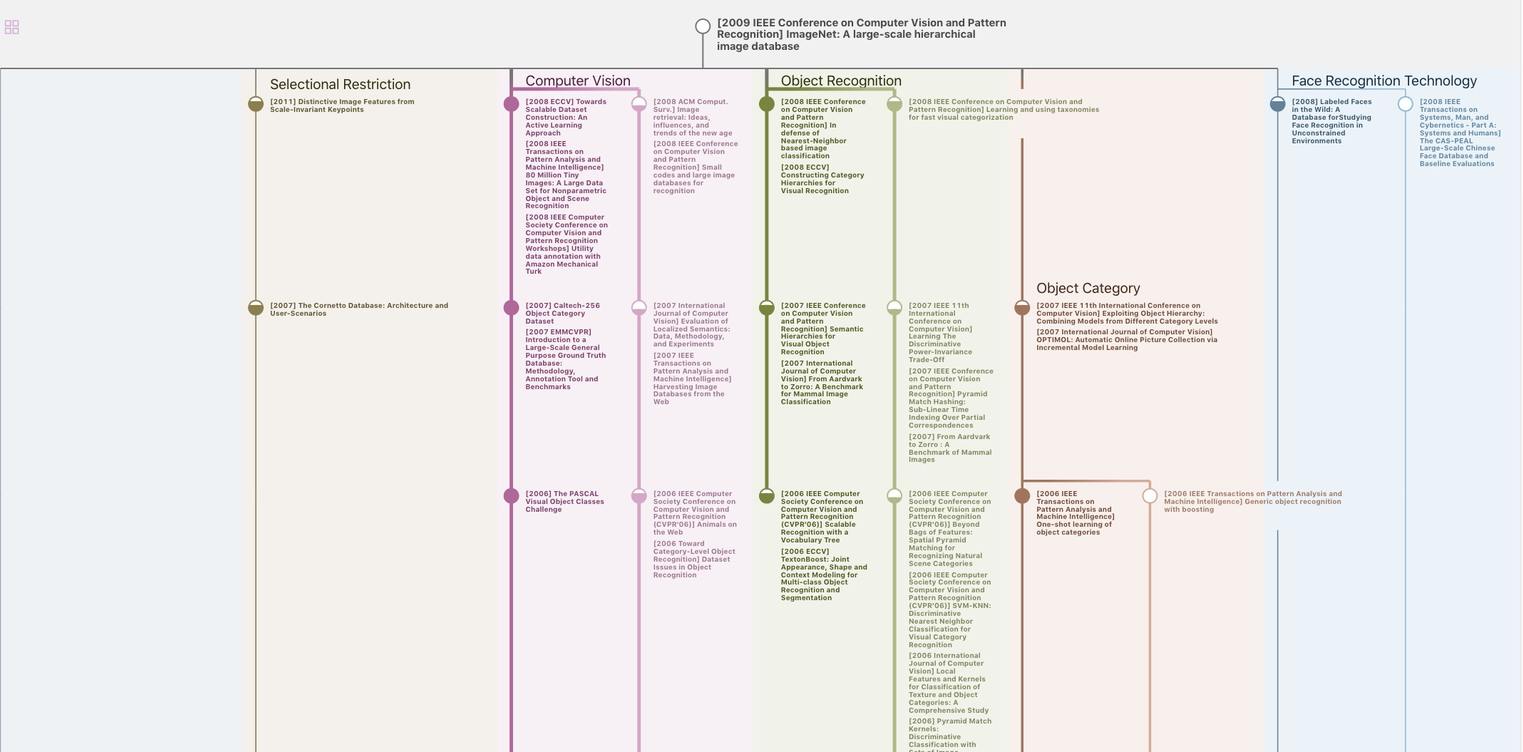
生成溯源树,研究论文发展脉络
Chat Paper
正在生成论文摘要