Kernel-Based Feature Extraction for Time Series Clustering.
KSEM (1)(2023)
摘要
Time series clustering is a key unsupervised data mining technique that has been widely applied in various domains for discovering patterns, insights and applications. Extracting meaningful features from time series is crucial for clustering. However, most existing feature extraction algorithms fail to capture the complex and dynamic patterns in time series data. In this paper, we propose a novel kernel-based feature extraction algorithm that utilizes a data-dependent kernel function with an efficient dimensionality reduction method. Our algorithm can adapt to the local data distribution and represent high-frequency subsequences of time series effectively. We demonstrate that, with a bag-of-words model, our feature extraction algorithm outperforms other existing methods for time series clustering on many real-world datasets from various domains.
更多查看译文
关键词
time series clustering,feature extraction,kernel-based
AI 理解论文
溯源树
样例
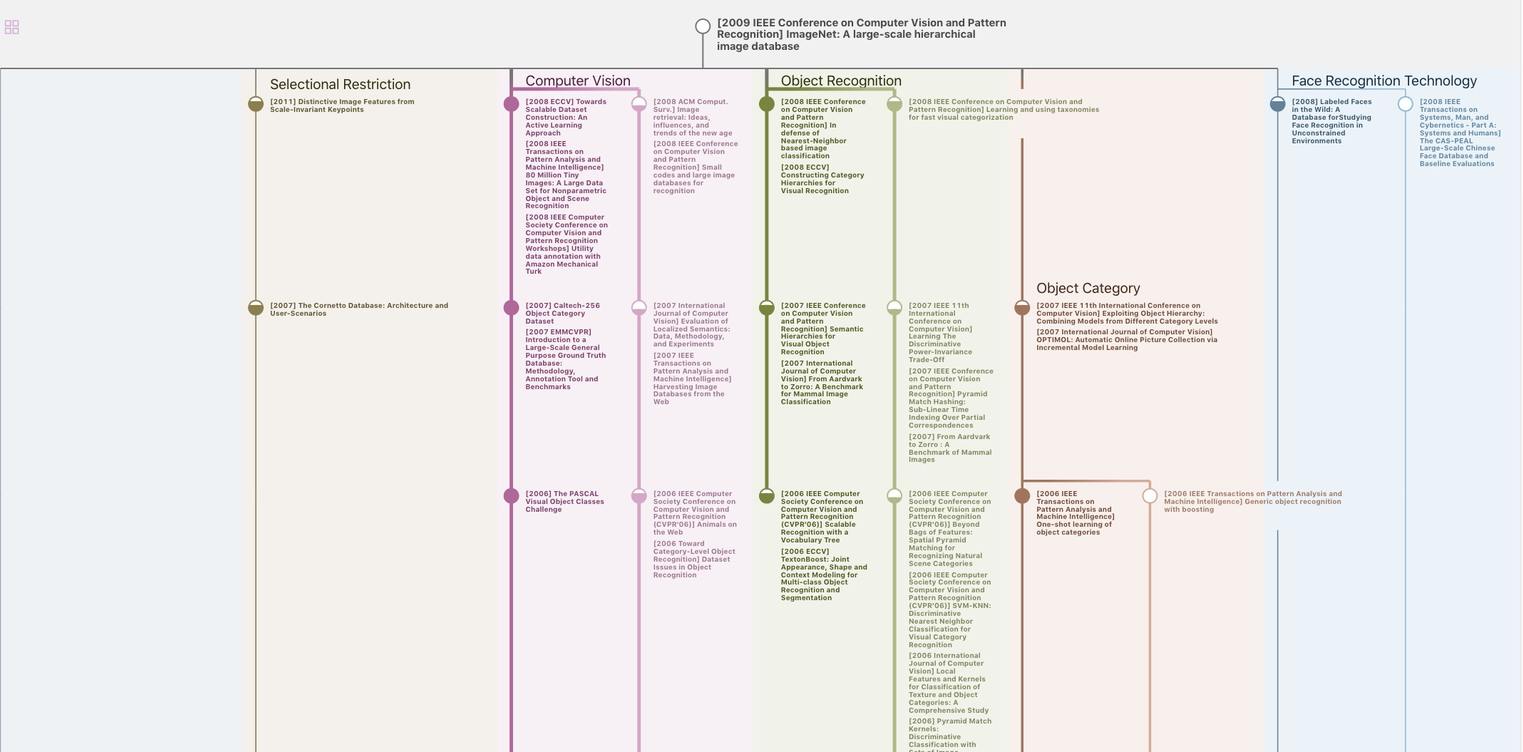
生成溯源树,研究论文发展脉络
Chat Paper
正在生成论文摘要