Industrial defect detection on the edge with deep learning over scarcely labeled and extremely imbalanced data.
COINS(2023)
摘要
Reliable automated defect detection is an integral part of modern manufacturing and improved performance can provide a competitive advantage. Despite the proven capabilities of convolutional neural networks (CNNs) for image classification, application on real world tasks remains challenging due to the high demand for labeled and well balanced data of the common supervised learning scheme. Semi-supervised learning (SSL) promises to achieve comparable accuracy while only requiring a small fraction of the training samples to be labeled. However, SSL methods struggle with data imbalance and existing benchmarks do not reflect the challenges of real world applications. In this work we present a CNN-based defect detection unit for thermal sensors. We describe how to collect data from a running process and release our dataset of 1k labeled and 293k unlabeled samples. Furthermore, we investigate the use of SSL under this challenging real world task.
更多查看译文
关键词
quality assurance,edge computation,imbalanced data,semi-supervised learning
AI 理解论文
溯源树
样例
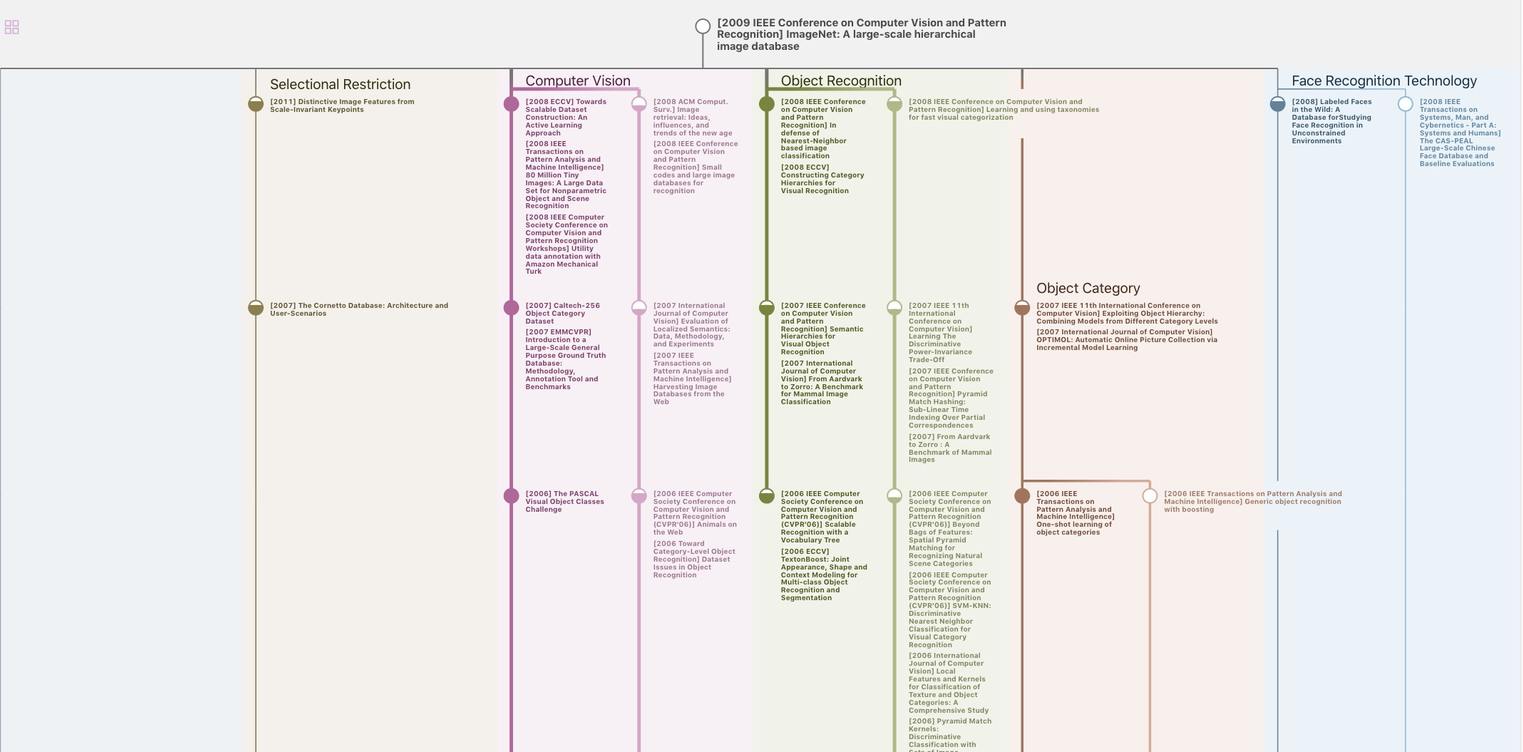
生成溯源树,研究论文发展脉络
Chat Paper
正在生成论文摘要