Online Consistent Video Depth with Gaussian Mixture Representation
2023 IEEE INTERNATIONAL CONFERENCE ON ROBOTICS AND AUTOMATION, ICRA(2023)
摘要
We demonstrate how off-the-shelf single-image depth estimation methods can be augmented with guidance from optical flow to achieve consistent and accurate online depth estimation using video sequences of static scenes. While previous work has successfully leveraged the complementary nature of optical flow and depth estimation, these techniques use computationally expensive test time optimization strategies that do not generalize beyond a single video sequence and also require knowledge of the future. In contrast, we present a computationally efficient feed-forward design that runs in an online fashion by utilizing learned data priors from previously seen video sequences. To accomplish this, we propose a continuous geometric scene representation that parametrically and compositionally represents the scene as a Gaussian Mixture Model (GMM). Based on this representation, our pipeline learns to estimate consistent depths and associated camera poses from video sequences of static scenes without direct supervision. Our online method achieves state-of-the-art results compared against offline methods that require all sequence frames.
更多查看译文
AI 理解论文
溯源树
样例
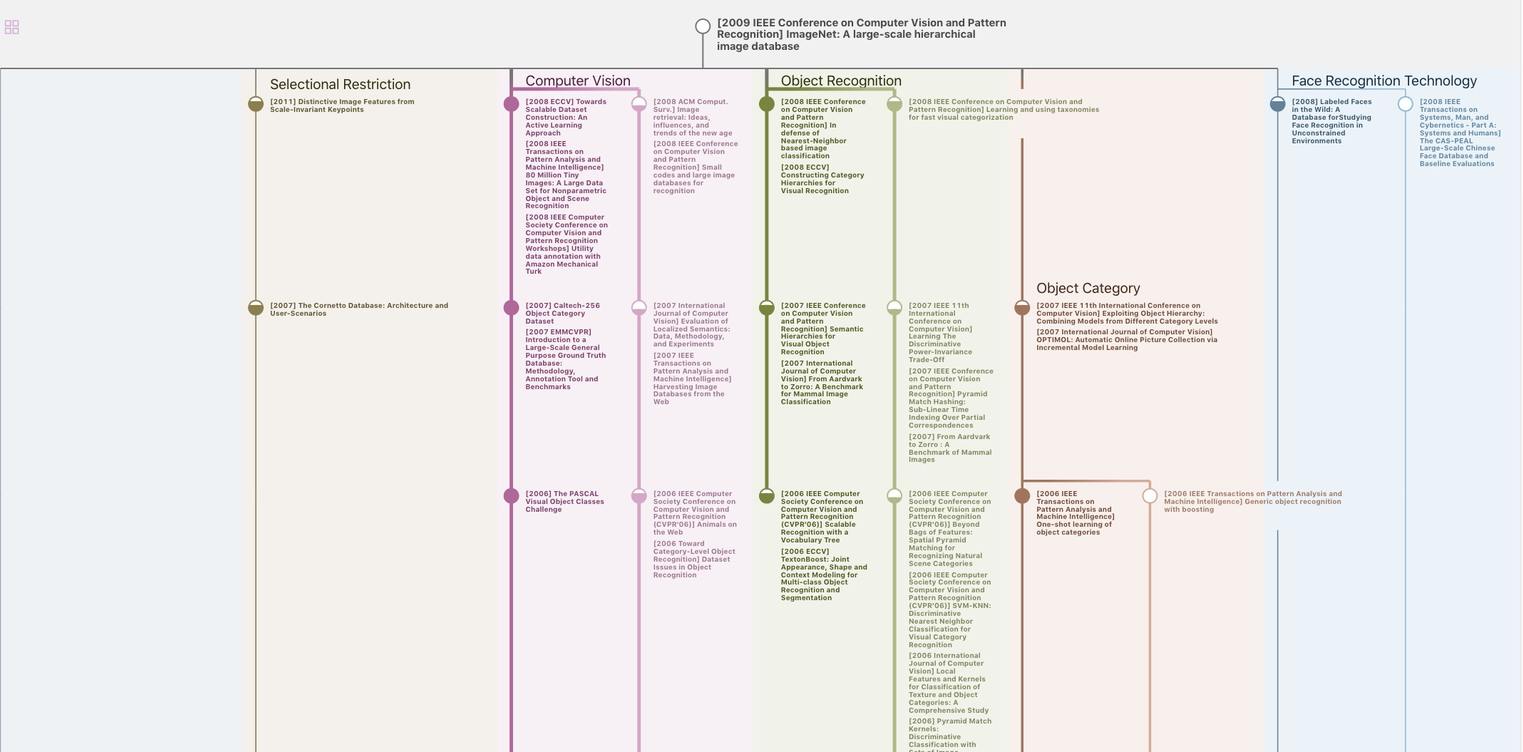
生成溯源树,研究论文发展脉络
Chat Paper
正在生成论文摘要