Multi-Head Attention Machine Learning for Fault Classification in Mixed Autonomous and Human-Driven Vehicle Platoons.
ICRA(2023)
摘要
Connected Autonomous Vehicle (CAV) platoons have been extensively studied to protect against cyber and physical vulnerabilities. Faults can occur in all layers of the platoon system or could be introduced by impaired human drivers. Since different types of faults may require different fault resolution methods, identifying the fault class facilitates the selection of the best mitigation strategy. This paper introduces a Multi-Head Attention Machine Learning (MHA-ML) approach to classify a set of five different faults and abnormalities in mixed autonomous and human-driven vehicle platoons. Autonomous vehicles can face actuator faults, False Data Injection (FDI) attacks, and Denial-of-Service (DoS) attacks, while abnormalities such as drunk or distracted human drivers could occur. MHA-ML is developed to identify faulty vehicle behavior over long sequences of sensor measurements. MHA-ML is trained on a mixed platoon simulation model and then tested on mobile laboratory robots. The experiment classifies the five fault categories with 90% accuracy and outperforms a baseline recurrent neural network approach.
更多查看译文
关键词
actuator faults,Autonomous Vehicle platoons,Autonomous vehicles,different fault resolution methods,False Data Injection attacks,fault categories,fault class,fault classification,faulty vehicle behavior,human-driven vehicle platoons,impaired human drivers,MHA-ML,mixed Autonomous,mixed platoon simulation model,MultiHead Attention Machine,platoon system
AI 理解论文
溯源树
样例
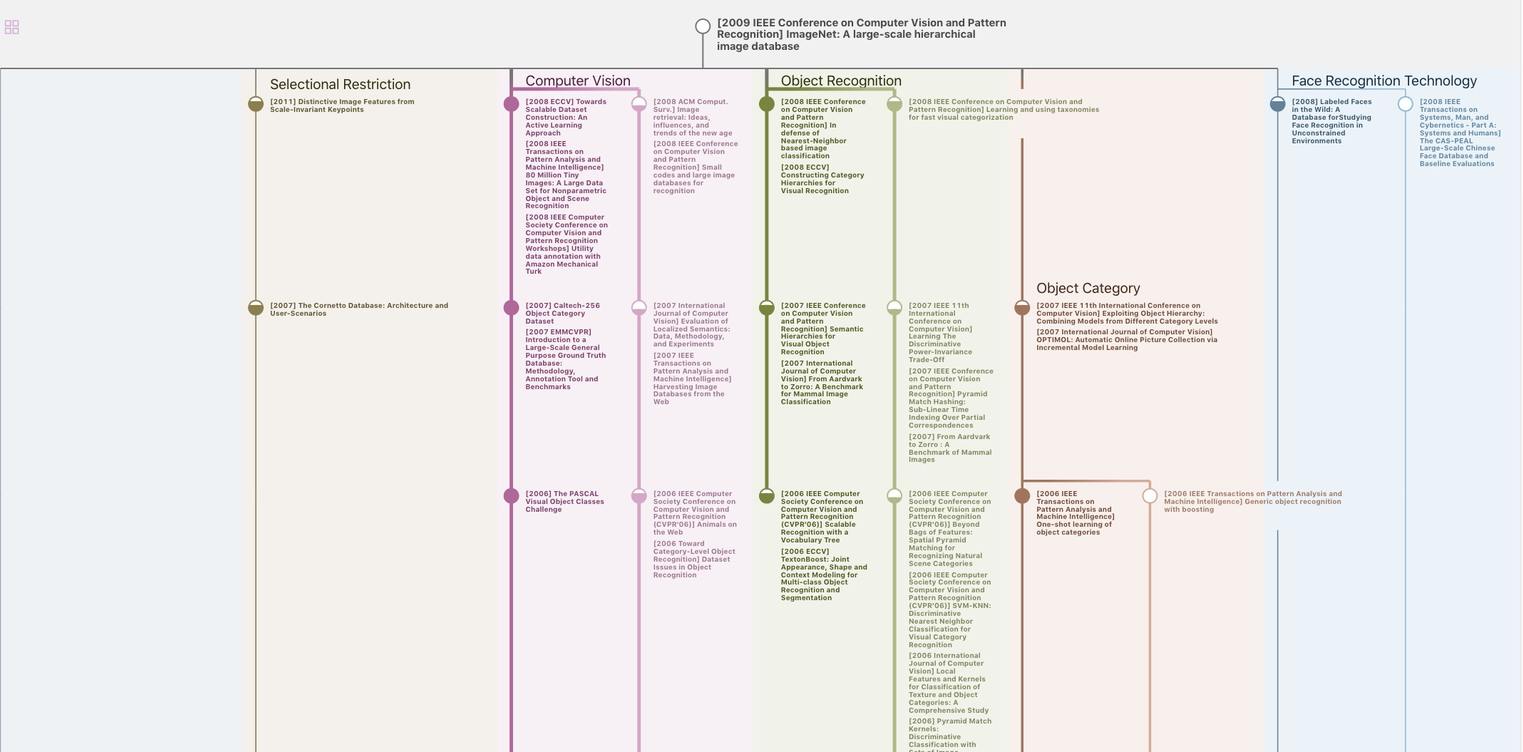
生成溯源树,研究论文发展脉络
Chat Paper
正在生成论文摘要