UGTransformer: Unsupervised Graph Transformer Representation Learning.
IJCNN(2023)
摘要
This paper mainly studies graph representation learning in unsupervised scenarios combined with Transformer models. Transformer network models have been widely used in many fields of machine learning and deep learning, and the application of transformer architectures to graph data has been very popular recently. For graph data, the field of graph representation learning has recently attracted a lot of attention. Graph-level representation is widely used in the real world, such as drug molecule design and disease classification in biochemistry. Traditional graph kernel methods, which design different graph kernels for different substructures, are simple but have poor generalization performance. Recently methods based on language models, such as graph2vec, use a particular substructure as the graph representation, which is also similar to the hand-crafted approach and also leads to poor generalization ability. In this paper, we propose the UGTransformer model, which builds on the standard Transformer architecture. We introduce several simple and effective structural encoding methods in order to encode the structural information of the graph into the model efficiently. The unsupervised representation of graphs is learned through a multi-headed attention mechanism and by using powerful aggregation functions. We conducted experiments on a benchmark date set for graph classification, and the experimental results validate the effectiveness of our proposed model.
更多查看译文
关键词
Transformer,unsupervised graph representation learning,structural encoding methods,graph classification
AI 理解论文
溯源树
样例
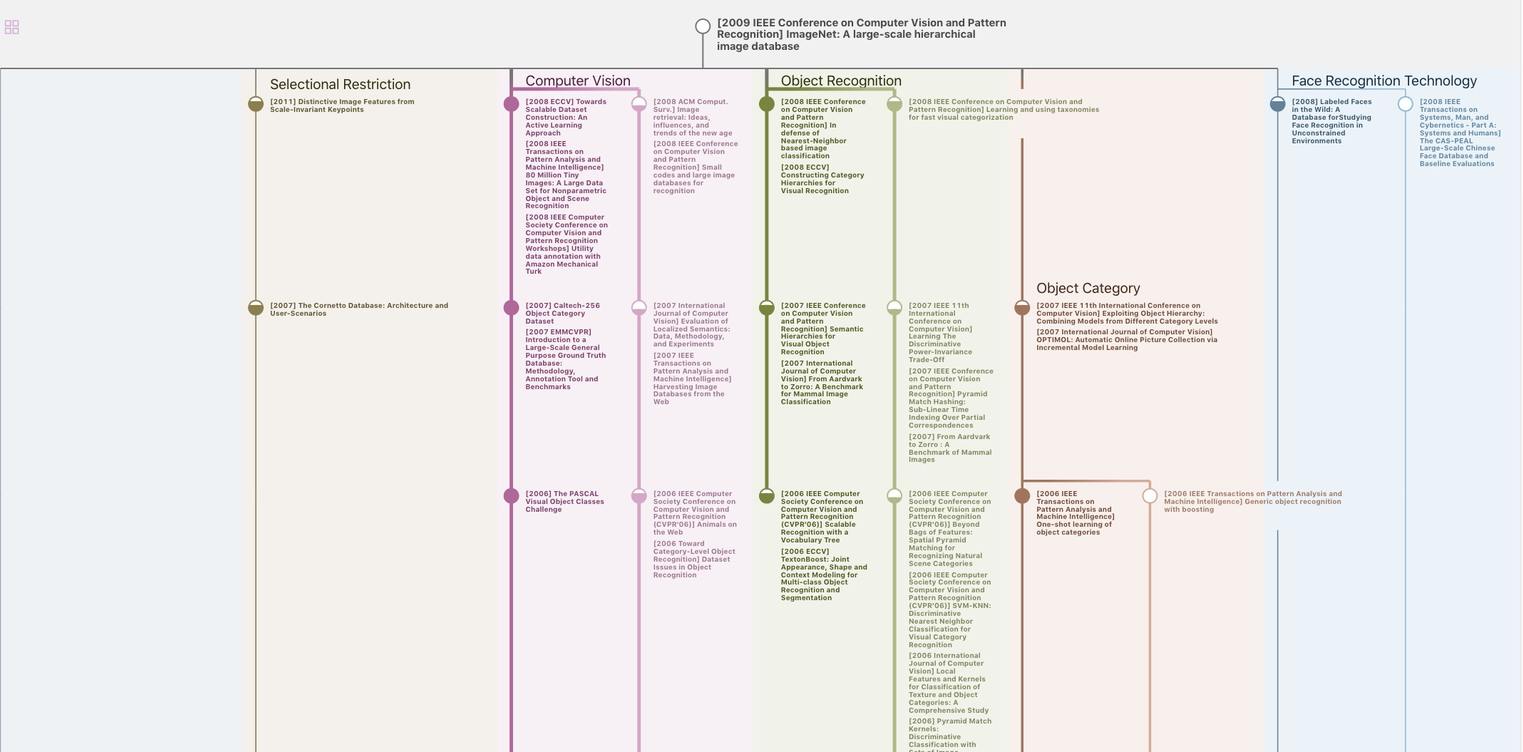
生成溯源树,研究论文发展脉络
Chat Paper
正在生成论文摘要