Multi-Truth Discovery While Being Aware of Unbalanced Data Distribution.
IJCNN(2023)
摘要
Due to information explosion, conflicting data on the same object among multiple sources is ubiquitous on the Web. To solve those conflicts while estimating source reliability, truth discovery has become a hot topic. However, when considering multi-value objects, the inevitable unbalanced data distribution is overlooked by the existing approaches. In particular, only a few sources make lots of claims while most sources only provide a few claims, which renders the source reliability estimated for "small" sources totally random; Some objects are covered by plenty of sources while some objects are claimed by only a few sources, which causes the value correctness calculated for "cold" objects unreasonable. To tackle the unbalanced data where multi-value objects exist, we propose a confidence interval based approach (CIMTD). We estimate source reliability from two aspects, i.e., the ability to claim the correct number of value(s) and specific value(s) on an object. To reflect the real reliability for both "big" and "small" sources, confidence intervals of enriched estimation are considered. While estimating source reliability, uncertainty degrees are introduced to model object differences. Confidence intervals are also considered to reflect the real uncertainty for both "hot" and "cold" objects. Experimental results on two realworld datasets demonstrate the effectiveness of our approach.
更多查看译文
关键词
truth discovery,multi-value objects,unbalanced data,confidence interval,object uncertainty
AI 理解论文
溯源树
样例
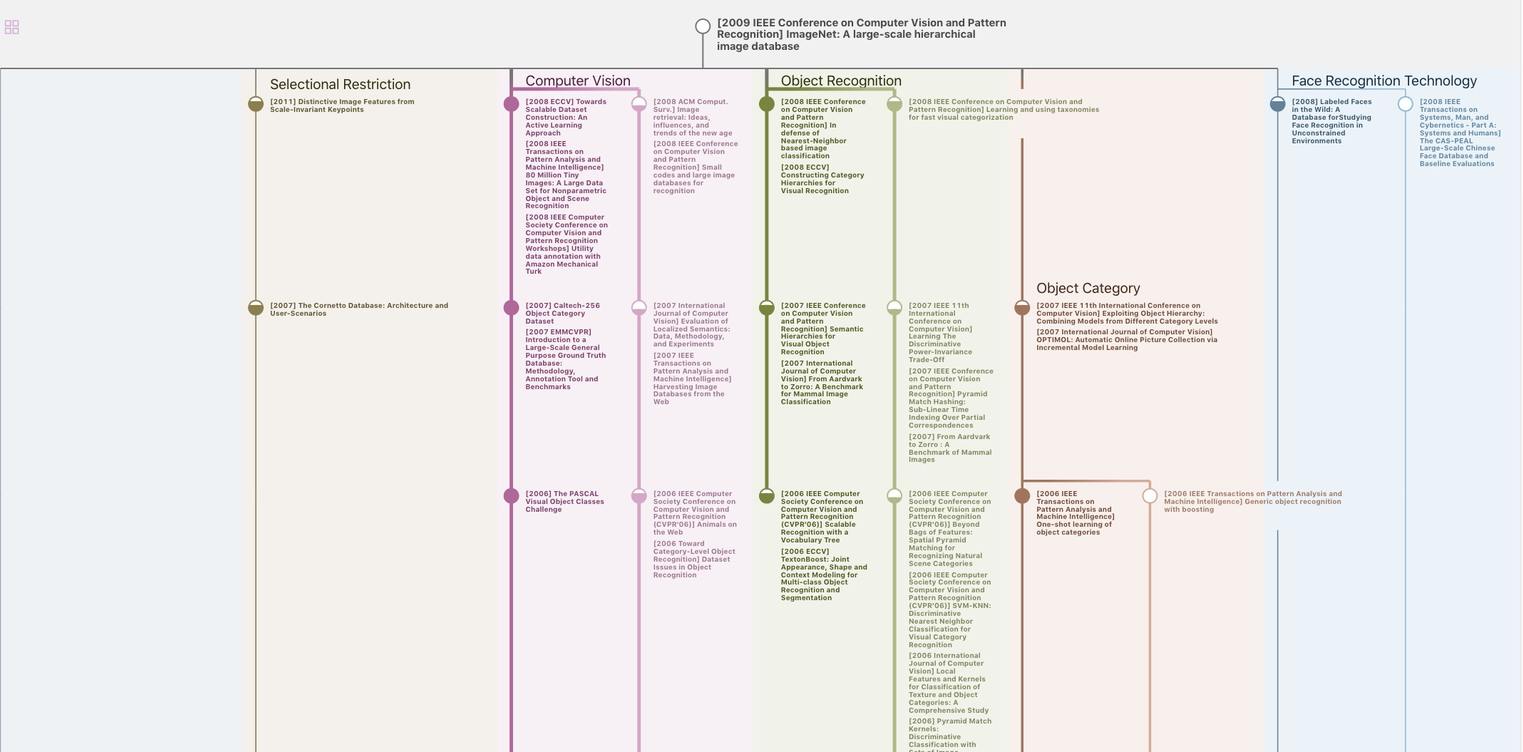
生成溯源树,研究论文发展脉络
Chat Paper
正在生成论文摘要