Improving Siamese Neural Networks with Border Extraction Sampling for the use in Real-Time Network Intrusion Detection.
IJCNN(2023)
摘要
Society reaps the benefits of networking technologies, with the number of connected citizens and devices constantly on the rise. The convenience and efficiency brought by connected technologies are adopted in normal households and industrial plants alike. As the proliferation of the technology expands, the incentives for malicious users to cause mischief are also getting stronger. This causes an influx in cyber incidents. To deal with the rising cyberthreats, a suite of defensive methods has been proposed. One prominent example is network intrusion detection systems. The Machine-Learning-based network intrusion detection systems utilised in critical infrastructure or soft target protection offer many benefits, but still, need improvements in numerous areas. This paper contains a proposition of an improved network intrusion detection system featuring a Siamese network as a few-shot learner. The used NetFlow features allow the system to perform real-time intrusion detection, the Siamese network allows spotting attacks from classes that were not used during the training of the network, and the used sampling method allows circumventing the over-counting problem when formulating sample pairs to train the Siamese networks. The results of the research are presented and show promise.
更多查看译文
关键词
Network Intrusion Detection, Few-Shot Learners, Siamese Neural Networks, Artificial Neural Networks
AI 理解论文
溯源树
样例
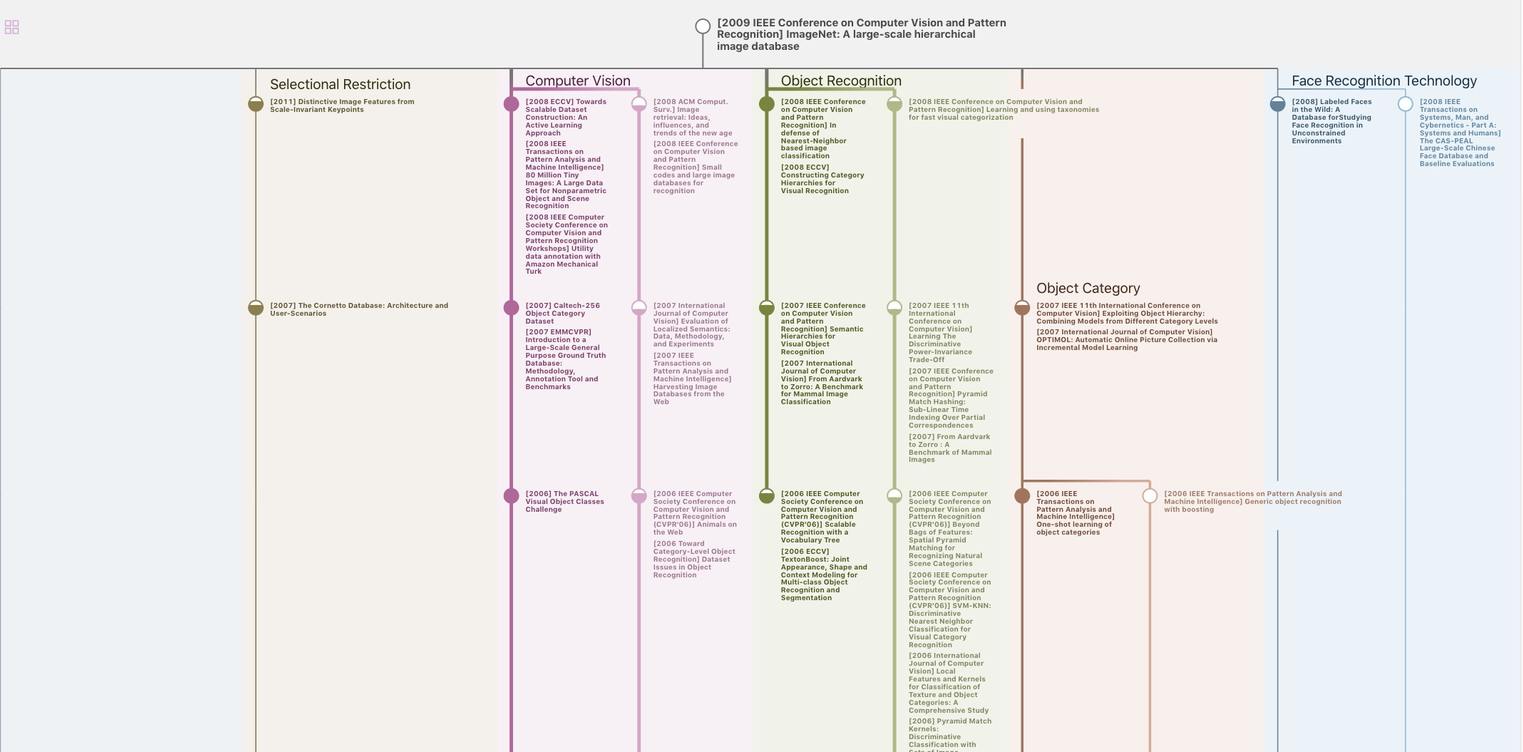
生成溯源树,研究论文发展脉络
Chat Paper
正在生成论文摘要