An homeostatic activity-dependent structural plasticity algorithm for richer input combination.
IJCNN(2023)
摘要
This paper introduces a novel rate-based variant of homeostatic activity-dependent structural plasticity (HADSP) for echo state networks. Despite its importance in brain development, structural plasticity has been largely overlooked in artificial neural networks. Our algorithm, although using only homeostatic plasticity, let emerge principles of Hebbian learning. Our analysis sheds light on the information processing capabilities of HADSP-powered echo state networks and suggests that HADSP effectively leverages the inter-relationships of the network's inputs. The study highlights the potential for rate-based HADSP to contribute to the field of computational neuroscience and plasticity in echo state networks. Furthermore, our findings highlight the crucial role of structural plasticity in influencing network function and organization and contribute significantly to the ongoing research on leveraging plasticity for the advancement of reservoir computing techniques.
更多查看译文
关键词
artificial neural networks,brain development,computational neuroscience,HADSP-powered echo state networks,homeostatic activity-dependent,homeostatic plasticity,information processing capabilities,leveraging plasticity,network function,novel rate-based,rate-based HADSP,richer input combination,structural plasticity
AI 理解论文
溯源树
样例
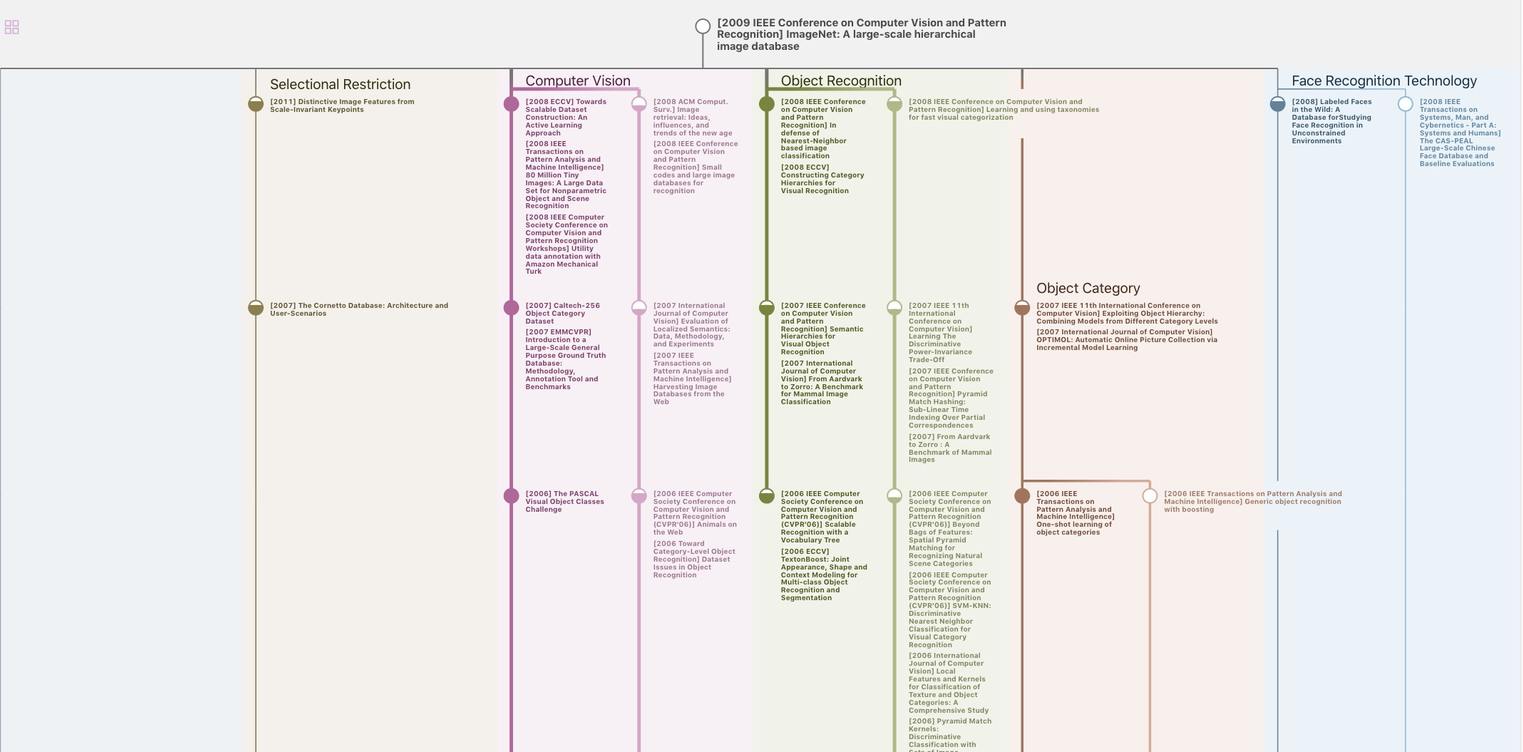
生成溯源树,研究论文发展脉络
Chat Paper
正在生成论文摘要