Transformer-Based Multi-Source Domain Adaptation Without Source Data.
IJCNN(2023)
摘要
Source-free domain adaptation (SFDA) aims at target adaptation without access to source data, but with only pretrained source model. A recent line of work proposed to automatically combine the source models with suitable weights so that it performs at least as good as the best source model when there are multiple pre-trained source models. In addition, some works proposed to preserve consistent inter-class relationships across domains to promote more shared transferable knowledge from source domains towards target adaptation. However, these works ignore the generalization ability of the pre-trained source models, which profoundly affects the initial target predictions that are crucial to the target adaptation stage. To this end, we develop a generic and effective framework based on Transformer, called TransMDA, for multi-source-free domain adaptation (MSFDA). Specifically, we inject the Transformer as the attention module into the convolutional network of each source model since it has the ability to encourage the model to turn attention towards the object regions, which can dramatically improve the model's generalization on the target domain. Furthermore, a novel pseudo-label smoothing strategy is proposed to avoid overfitting to the target domain. Experiments on several challenging datasets demonstrate the superiority of our proposed TransMDA method.
更多查看译文
关键词
Domain adaptation,Self-training,Transformer,Image classification
AI 理解论文
溯源树
样例
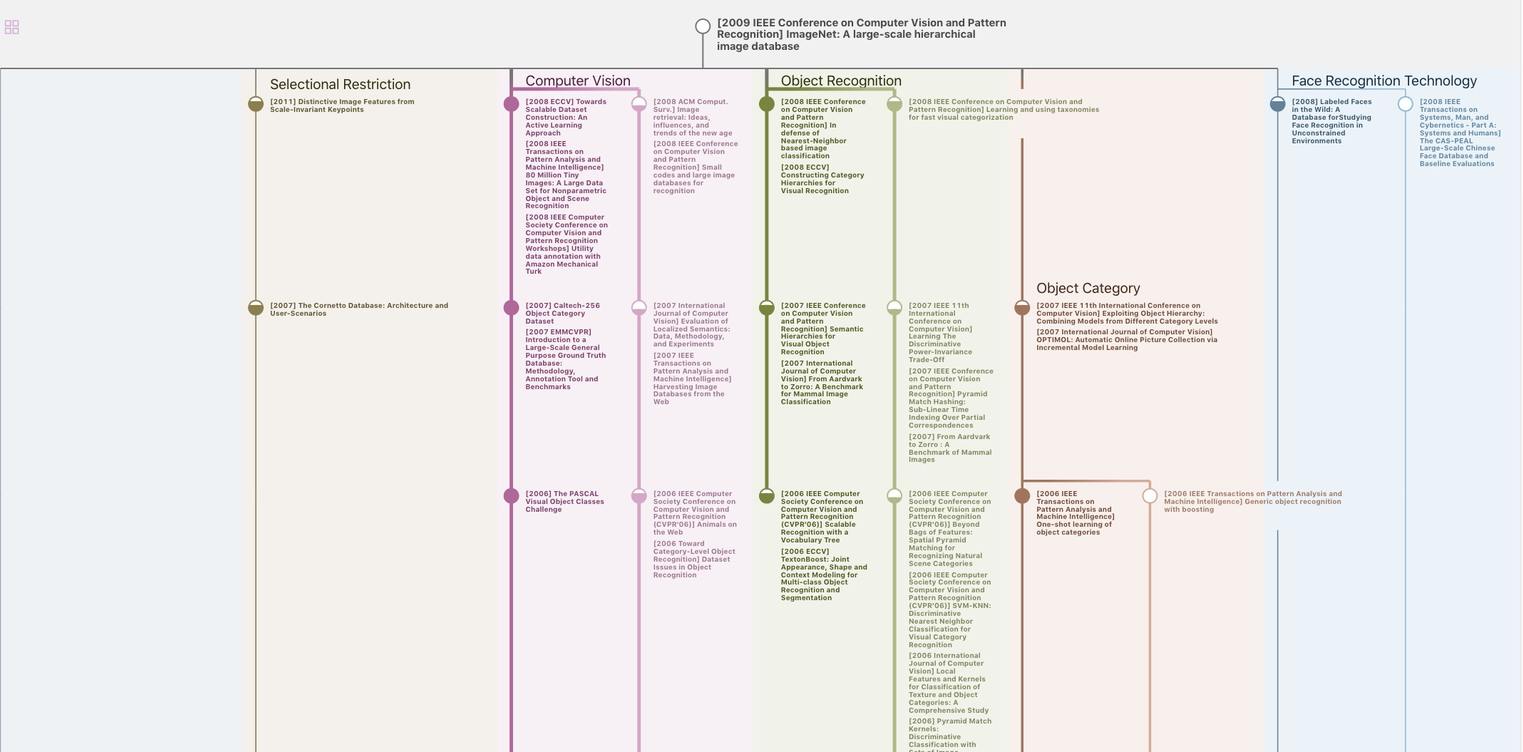
生成溯源树,研究论文发展脉络
Chat Paper
正在生成论文摘要