Improving the Transferability of Adversarial Examples with Diverse Gradients.
IJCNN(2023)
摘要
Previous works have proven the superior performance of ensemble-based black-box attacks on transferability. However, existing methods require significant difference in architecture among the source models to ensure gradient diversity. In this paper, we propose a Diverse Gradient Method (DGM), verifying that knowledge distillation is able to generate diverse gradients from unchangeable model architecture for boosting transferability. The core idea behind our DGM is to obtain transferable adversarial perturbations by fusing diverse gradients provided by a single source model and its distilled versions through an ensemble strategy. Experimental results show that DGM successfully crafts adversarial examples with higher transferability, only requiring extremely low training cost. Furthermore, our proposed method could be used as a flexible module to improve transferability of most of existing black-box attacks.
更多查看译文
关键词
Adversarial examples, Gradient diversity, Black-box attack, Transferability
AI 理解论文
溯源树
样例
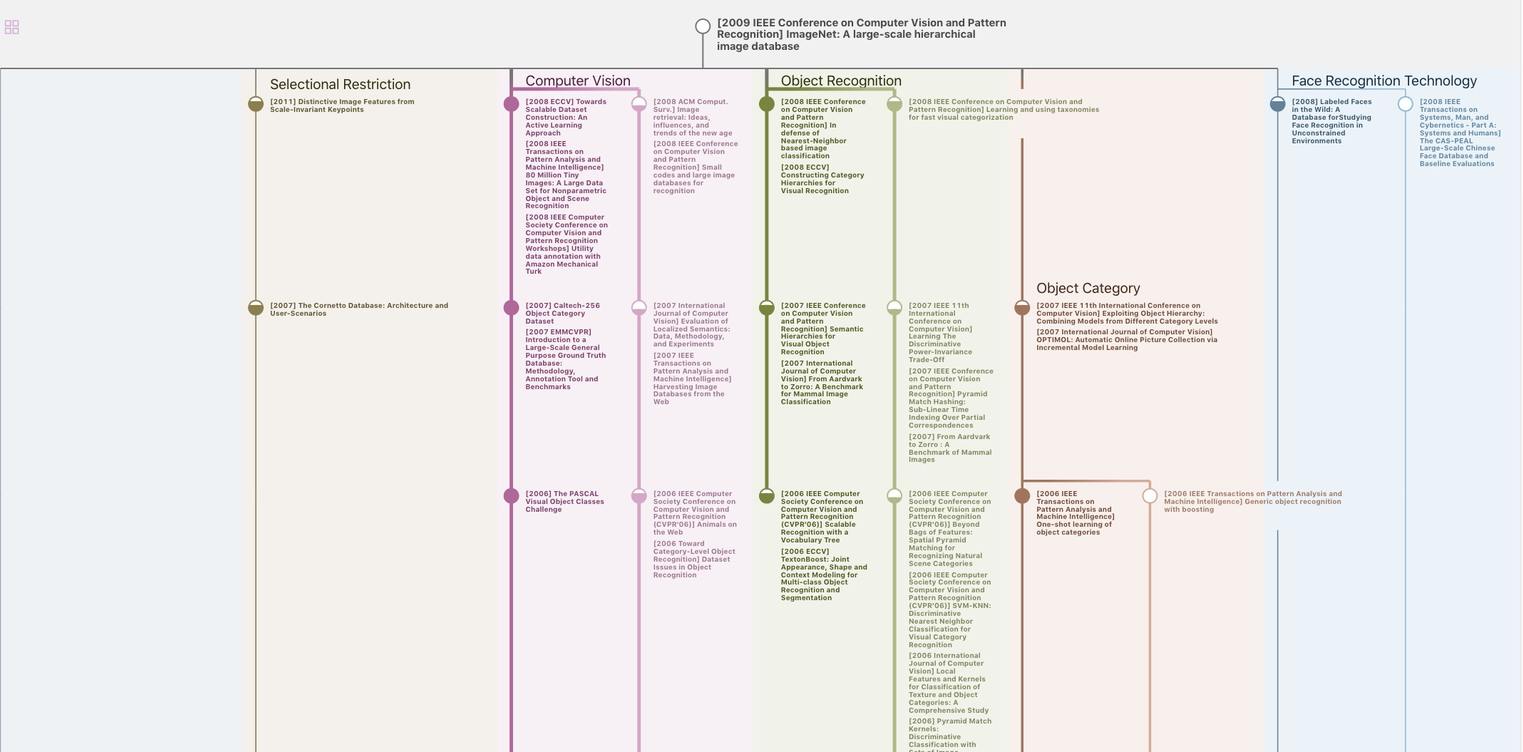
生成溯源树,研究论文发展脉络
Chat Paper
正在生成论文摘要