Weighted Spatial Pooling Preprocessing for Rank- ($L, L, 1,1$) BTD with Orthonormality: Application to Multi-Subject fMRI Data.
IJCNN(2023)
摘要
The rank- (
$L, L, 1,1$
) block term decomposition (BTD) with spatial orthonormality (BTD-O) applied to 4-way multi-subject fMRI data achieves good performance due to preserving higher spatial structure and reducing crosstalk between components. However, the high rank
$L$
value (e.g., 35) of BTD-O for fMRI data leads to high computation complexity. Moreover, multi-subject fMRI data contains high noise nature. Although an accelerated BTD-O (accBTD-O) was proposed, it showed similar performance to BTD-O. Inspired by the compression, smoothing, and spatial structure invariance features of the pooling scheme, we respectively propose weighted spatial 3D and 2D pooling preprocessing for BTD-O of fMRI data. These two methods give higher weight to meaningful in-brain voxels and reduce the size and noise of fMRI data. Specifically, weighted spatial 3D pooling compresses weighted 3D brain images of a 5-way fMRI tensor, then transforms pooled 5-way fMRI tensor into a 4-way fMRI tensor. In contrast, for weighted spatial 2D pooling, the 5-way weighted fMRI data is first transformed into a 4-way tensor, and then 2D brain images of 4-way fMRI tensor are compressed by 2D pooling. The pooled 4-way fMRI tensor is separated by BTD-O to extract shared spatial maps, shared time courses, and subject intensities. Results of simulated and experimental fMRI data analyses both demonstrate that these two proposed methods achieve obviously better task-related component than compared methods. Moreover, the proposed 3D pooling is about 2.243 times faster than accBTD-O.
更多查看译文
关键词
Block term decomposition (BTD),weighted spatial pooling,orthonormality,fMRI data
AI 理解论文
溯源树
样例
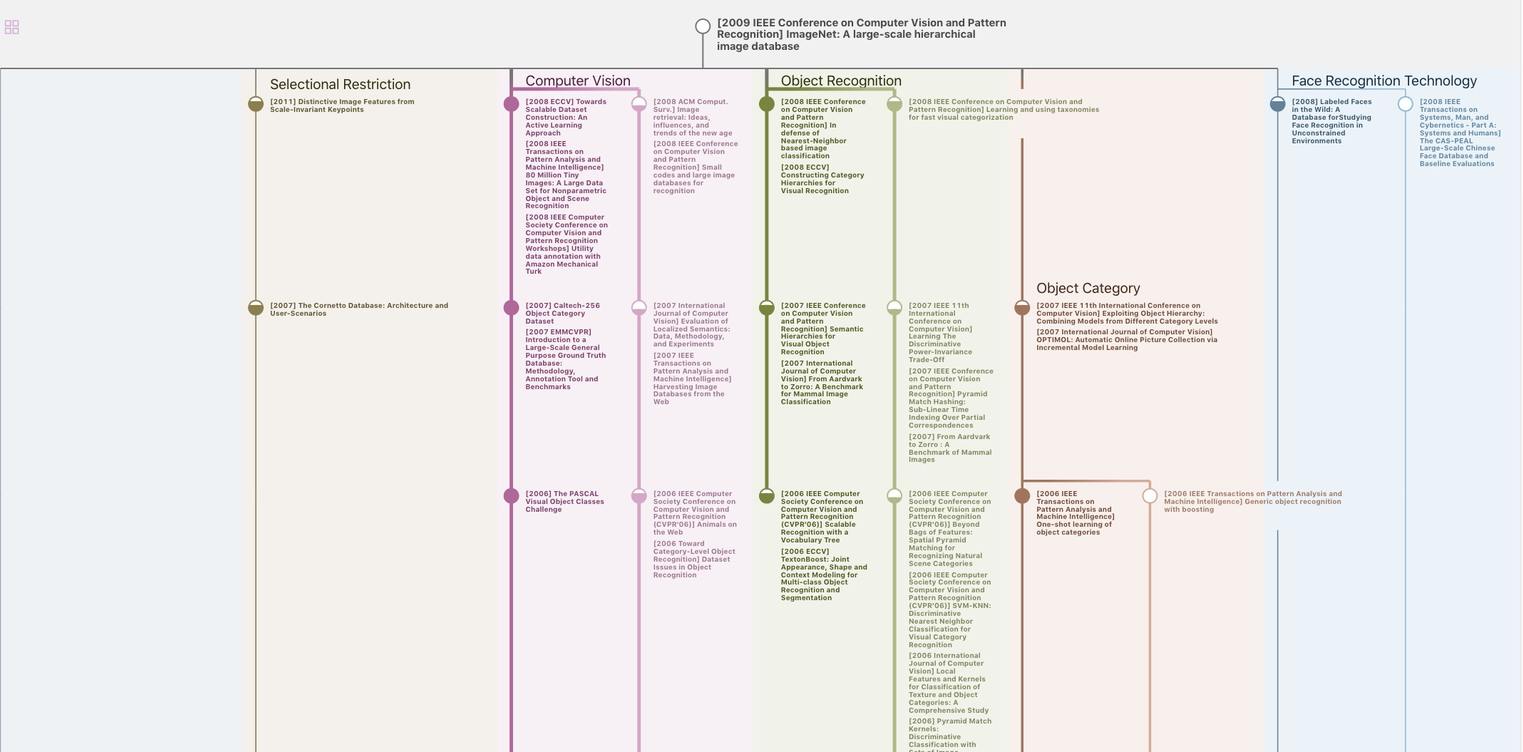
生成溯源树,研究论文发展脉络
Chat Paper
正在生成论文摘要