FLCAP: Federated Learning with Clustered Adaptive Pruning for Heterogeneous and Scalable Systems
2023 INTERNATIONAL JOINT CONFERENCE ON NEURAL NETWORKS, IJCNN(2023)
摘要
In this paper, we address the challenge of efficient local training on heterogeneous data in Federated Learning, where devices have limited computation and communication resources. The proposed algorithm generates personalized masks for groups of nodes by applying structured or unstructured pruning methods. We find a trade-off between global generalization and per-device personalization by optimizing the personalized masks to local data distributions through clustering methods and by aggregating the parameters in a unique model. Our results demonstrate effectiveness of the method for training personalized and sparsified models on non-IID distributions. The maximal accuracy achieved by our Clustered Adaptive Pruning method outperforms the Federated Average algorithm, while using a small percentage of the original set of coefficients per dataset. In particular, for MNIST, CIFAR10, and FMNIST datasets, our method uses approximately two-thirds, one-third, and less than one-third of the total number of parameters required for Federated Average training, respectively.
更多查看译文
关键词
federated learning,personalization,pruning,data heterogeneity,scalable systems
AI 理解论文
溯源树
样例
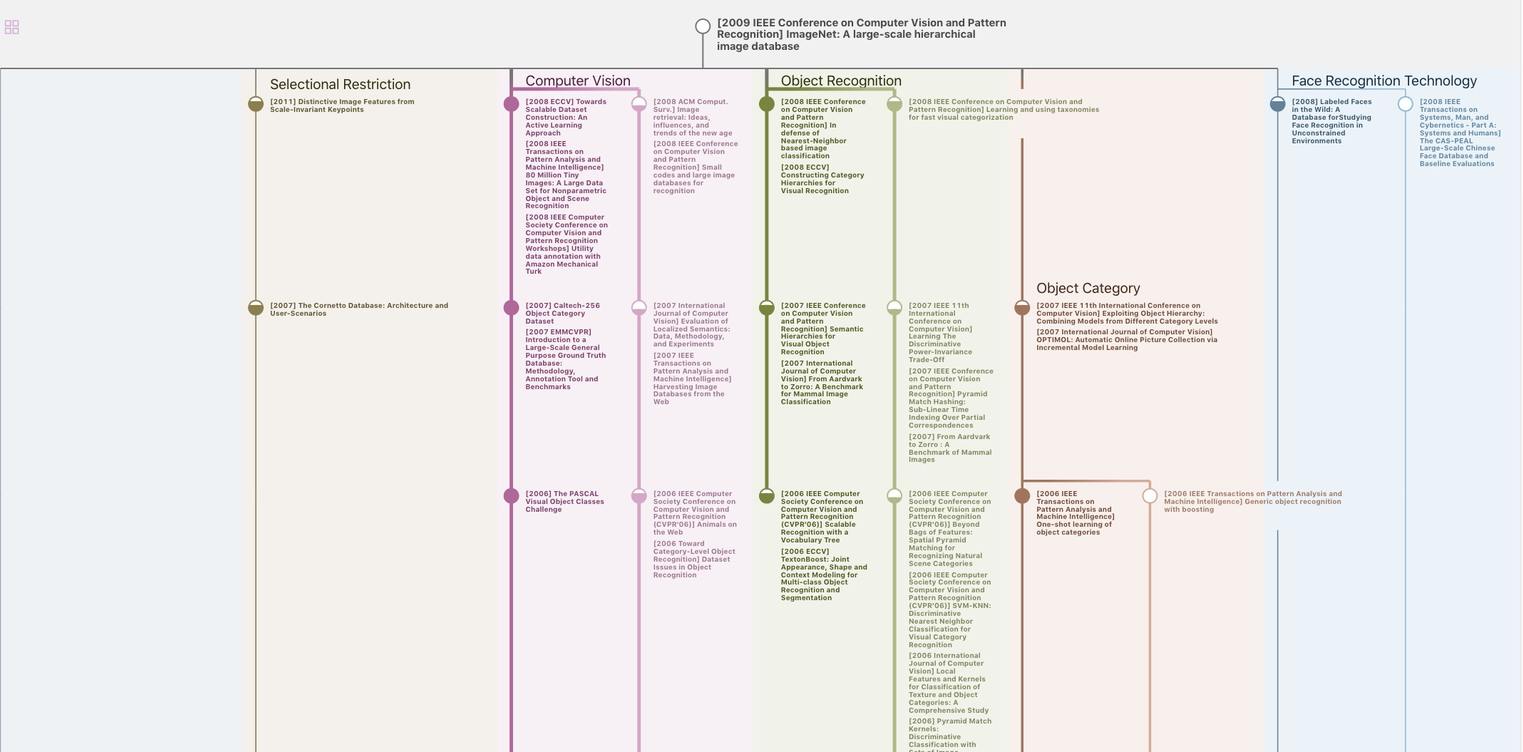
生成溯源树,研究论文发展脉络
Chat Paper
正在生成论文摘要