Learn to Encode Heterogeneous Data: A Heterogeneous Aware Network for Multi-Future Trajectory Prediction.
IJCNN(2023)
摘要
With the development of intelligent transportation and human-robot interaction systems in recent years, the demand for multi-future trajectory prediction is increasing rapidly. A key challenge is to model the stochasticity of future agents motion, which affects the prediction for Multi-Future Trajectory directly. Numerous trajectory prediction methods have been proposed by using heterogeneous data composed of High Definition (HD) maps and agents' history trajectories to model the future agents motion. As we know, regions where there is an object will enter contain more valuable features than regions where there is an object has already passed through. But existing methods are usually influenced by the passed regions in HD maps, which leads to unreasonable predictions. To mitigate this problem, we present a conditional variational autoencoder based model, called Heterogeneous Aware Network (HANet), to forecast trajectories of agents in real-world scenes. The HANet is embedded with a carefully designed Discriminative Region Exploring module (DRE) to encode heterogeneous data. We also tailor a Predictive Sampling Strategy (PSS) to help the DRE focus on regions that agents will enter. The proposed HANet achieves competitive results on a real-world public dataset nuScenes compared with SOTA methods.
更多查看译文
关键词
multi-future trajectory prediction,heteroge-neous data,real-world
AI 理解论文
溯源树
样例
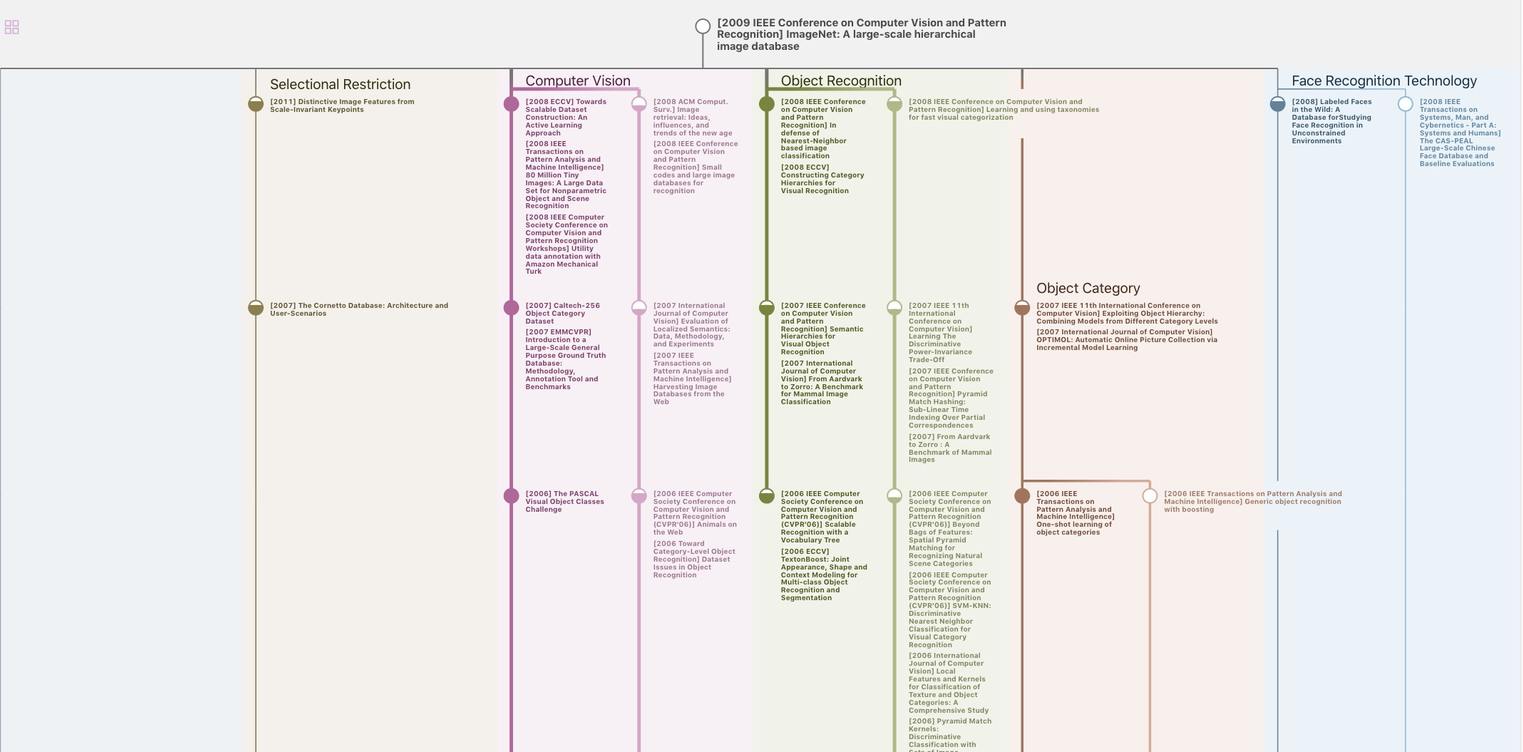
生成溯源树,研究论文发展脉络
Chat Paper
正在生成论文摘要