GENet: Guidance Enhancement Network for 3D Shape Recognition.
IJCNN(2023)
摘要
Both point cloud-based and view-based deep learning methods for 3D shape recognition have achieved relatively remarkable results in recent years. However, there are few methods to jointly represent 3D shapes from both point cloud and multi-view modal data. Therefore, we propose a guidance enhancement network (GENet) for 3D shape recognition based on multimodal data. On the one hand, the point cloud is encoded with features from both explicit and implicit aspects, and on the other hand, all views are encoded and constructed as a graph. In the multilayer guidance enhancement module, graph convolutional neural network (GCN) enhances each view feature, and then temporary high-level features (initially point cloud global feature) guide multiple low-level view features to obtain correlation coefficients, through which the views with higher importance are filtered as inputs for the next layer of the structure and the view features in the current layer are weighted and aggregated. The aggregated view features are then connected to the high-level features with residuals to form the enhanced high-level features. The 3D shape descriptor is finally obtained after several guidance and enhancements. The proposed GENet achieves state-of-the-art results on the 3D benchmark dataset ModelNet.
更多查看译文
关键词
3D benchmark dataset ModelNet,3D shape descriptor,3D shape recognition,aggregated view features,deep learning methods,GENet,graph convolutional neural network,guidance enhancement network,high-level features guide multiple low-level view features,initially point cloud global feature,multilayer guidance enhancement module,multiview modal data,point cloud-based
AI 理解论文
溯源树
样例
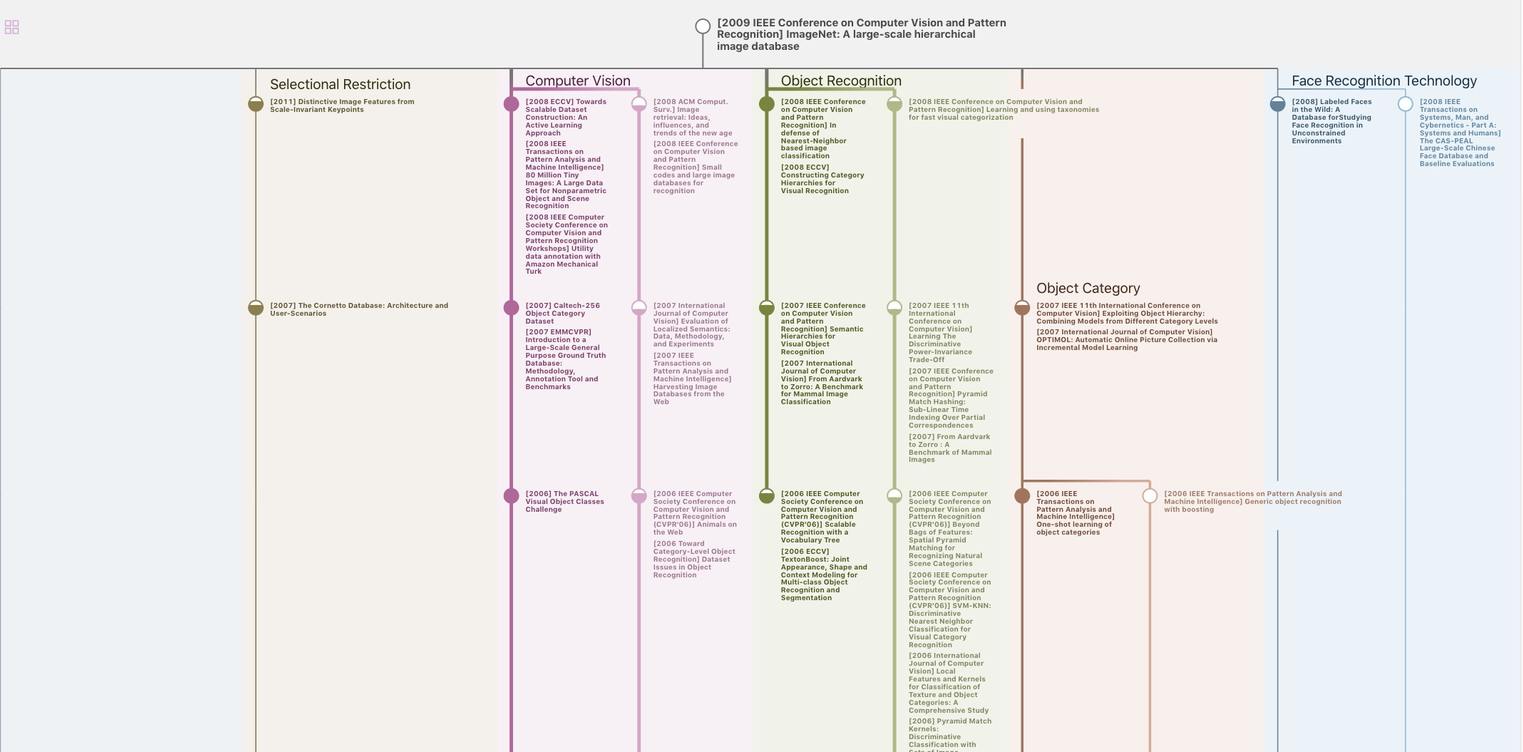
生成溯源树,研究论文发展脉络
Chat Paper
正在生成论文摘要