Machinery Anomaly Detection using artificial neural networks and signature feature extraction.
IJCNN(2023)
摘要
Machine learning models are increasingly being used in predictive maintenance. However, due to the complexity of vibration and audio signals used in fault diagnosis, some preprocessing is required before feeding them into the machine learning algorithm. Fast Fourier Transform (FFT) and the Hilbert transform (HT) envelope spectrum are mostly used in the literature for pre-processing. However, these frequency domain transforms are not very effective when applied to rotating systems (e.g. bearings) fault detection. In this paper we propose to use signature coefficients to feed machine learning models for fault detection. Our experimental results show that this method outperforms most state-of-the-art methods on fault diagnosis data sets. Moreover, the results show that this method is particularly well suited for high dimensional time series. The results also show that compared to Fast Fourier Transform (FFT), the signature method requires fewer data points to detect failure. This means that in a situation where the two methods have similar performances, the signature method detects failure faster than FFT.
更多查看译文
关键词
Fault diagnosis, Anomaly detection, Predictive maintenance, Concept drift detection, Data streams, Signature, Machine learning
AI 理解论文
溯源树
样例
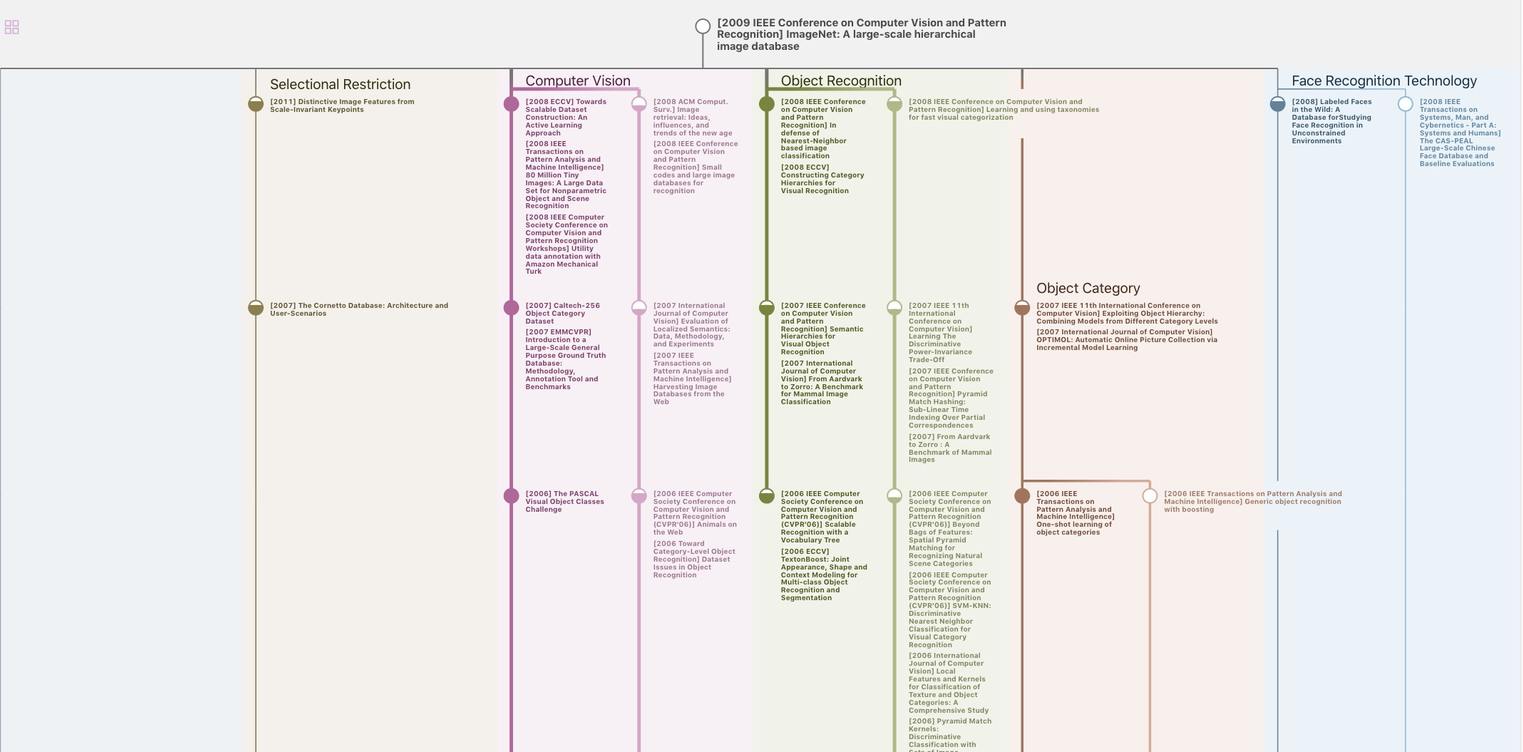
生成溯源树,研究论文发展脉络
Chat Paper
正在生成论文摘要