Mass-Based Short Term Selection of Classifiers in Data Streams.
IJCNN(2023)
摘要
Dynamic classifier selection (DCS) regards well-known machine learning techniques in the batch setting that leverage ensemble performance. Most of the methods use similarity-based methods as a proxy, culminating in high computation costs and becoming unfeasible in many streaming scenarios. In this paper, we propose a DCS method able to cope with the high-speed streaming setting, which is based on the performance of base learners in the most recent instances. The impact of our method is evaluated with different ensembles for data streams. We also propose modifications to an Online Boosting method, which has its performance improved with DCS. Our method increases the accuracy and kappa statistic of state-of-the-art ensembles with low overhead of time processing and memory.
更多查看译文
AI 理解论文
溯源树
样例
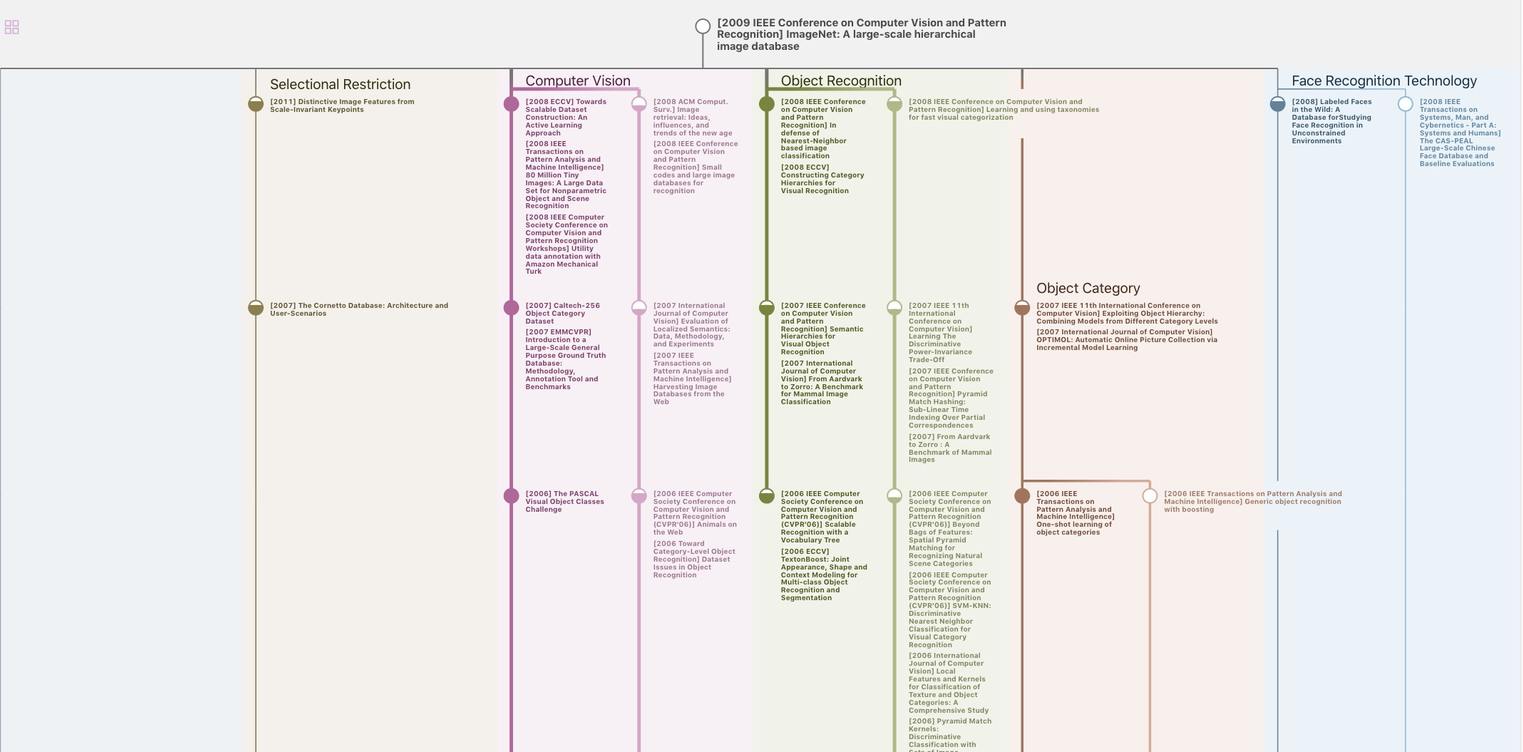
生成溯源树,研究论文发展脉络
Chat Paper
正在生成论文摘要