Model Driven Deep Unfolding Network for Extreme Low-Light Image Enhancement and Denoising.
IJCNN(2023)
摘要
Low visibility and severe noise are two main degradations in extreme low-light images. Nevertheless, existing lowlight image enhancement methods often fail to handle real lowlight images with strong noise. To address this issue, We propose a deep unfolding network based on the robust Retinex model with an additional noise term. In particular, we design an optimization model with implicit priors and employ the proximal gradient descent (PGD) technique to alternately solve three iterative sub-problems of the optimization model in a data-driven manner. The proposed method combines the interpretability of model-based methods with the speed and strong fitting ability of learning-based methods. In addition, we collect an extreme low-light sRGB image dataset (E-LOL) containing noisy low/normal-light image pairs. Extensive experimental results demonstrate that our method outperforms state-of-the-art methods in enhancing noisy low-light images and obtains better-exposed illumination, richer colors and textures.
更多查看译文
关键词
Low-light image enhancement, Deep unfolding network, Retinex model, Noise suppression
AI 理解论文
溯源树
样例
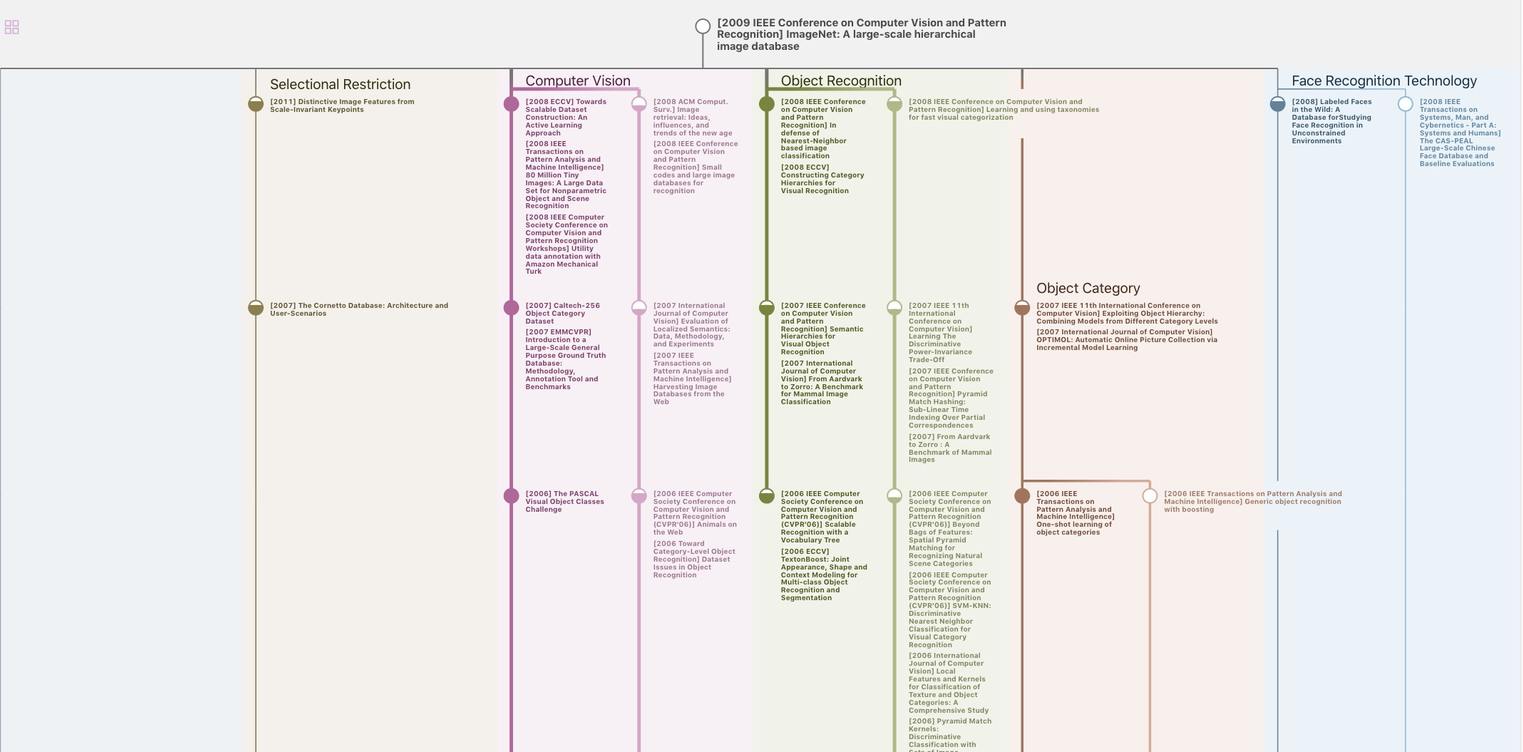
生成溯源树,研究论文发展脉络
Chat Paper
正在生成论文摘要