Novel Automatic Deep Learning Feature Extractor with Target Class Specific Feature Explanations.
IJCNN(2023)
摘要
have gained their popularity in various fields of computer vision, natural language processing etc, due to their excellent predictive accuracy and ability to automatically extract good features from raw input data. Though these models have these significant advantages, most deep learning models are notoriously black-box models. The features learned by these models are not explainable. The need to explain these predictions is important in many high stakes fields. In this work, we propose a method that uses convolutional layers like in Convolutional Neural Networks (CNNs) that automatically learns features from raw data. In our proposed method we extract feature representation that is unique to each target class in the given data. Visualizations of this unique representation explain the feature learned by the model for a specific target class. To classify an input data we use two approaches. The first approach uses the similarity scores to match the features learned for a particular instance with the extracted target class-specific features to make prediction. The second approach uses an intrinsically explainable model like logistic regression to make predictions. Thus our proposed method in addition to having good predictive accuracy, identifies features that are class-specific. The proposed method achieved an accuracy of 99.31% on MNIST data set, 90.45% on CIFAR-10 data set and 96.45% accuracy on Assira data set.
更多查看译文
关键词
black-box models,deep learning models,deep-learning models,excellent predictive accuracy,extracted target class-specific features,feature representation,good features,good predictive accuracy,high stakes fields,intrinsically explainable model,novel automatic deep learning feature extractor,popular machine learning models,raw data,raw input data,specific target class,target class specific feature explanations
AI 理解论文
溯源树
样例
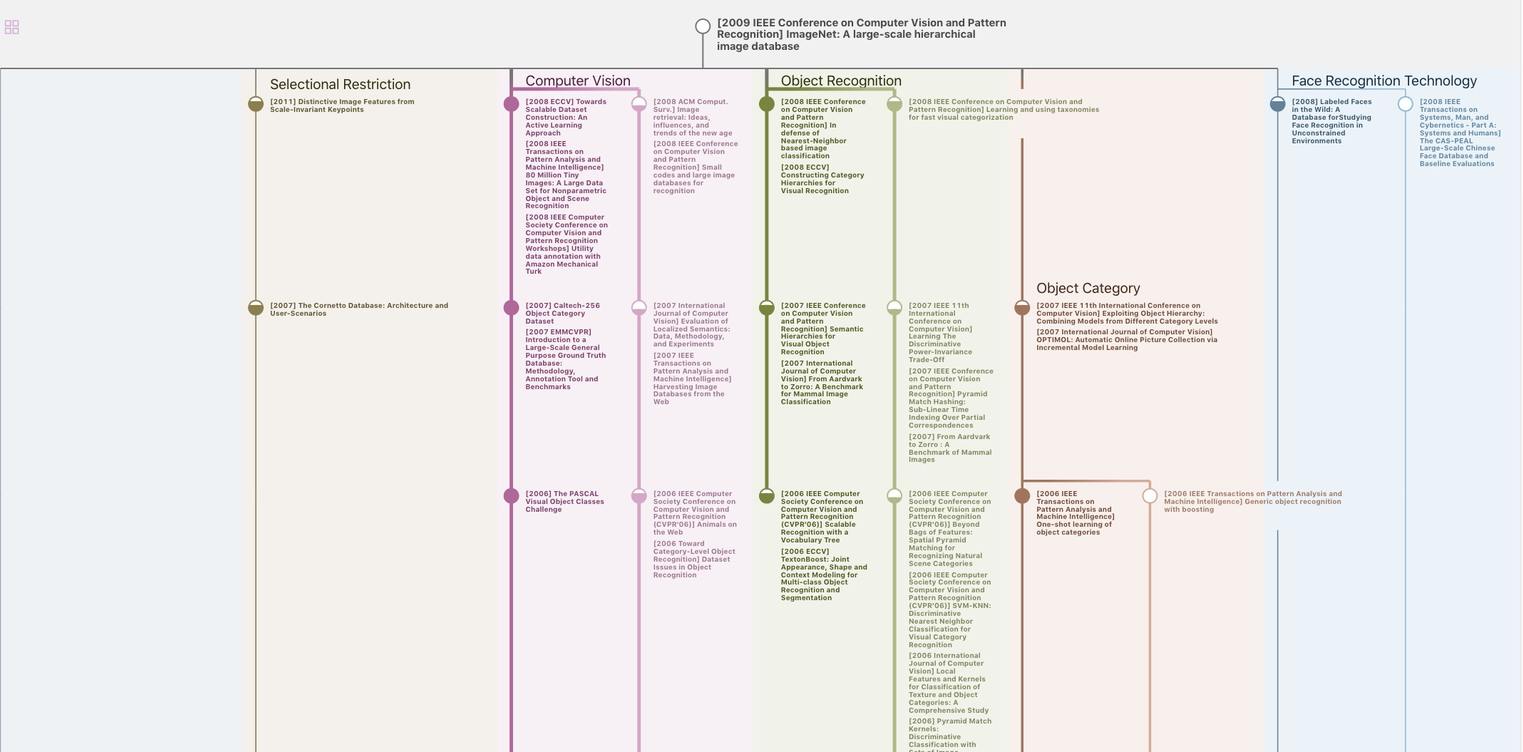
生成溯源树,研究论文发展脉络
Chat Paper
正在生成论文摘要