Visualization Tool for Extraction of Various Attributes and Corresponding Data for Dataset Quality Assessment
2023 IEEE International Conference On Artificial Intelligence Testing (AITest)(2023)
摘要
Generally, the quality of artificial intelligence (AI) models depends on the training dataset. Therefore, evaluating dataset quality is crucial. Datasets contain considerable attribute information, and the presence of noise attributes in the dataset can result in inferior performance in tasks such as classification and object detection. Thus, the numerous attributes contained in the dataset have to be extracted, and attributes that affect the performance of an AI model, as well as the presence and quantity of data with those attributes, have to be identified to assess dataset quality. In this study, we proposed a visualization tool for the extraction of various attributes and corresponding data for dataset quality assessment (VisTEx) by using an existing unsupervised learning method, multi-facet clustering variational autoencoders (MFCVAE). The proposed visualization tool displays the features extracted by MFCVAE on a two-dimensional (2D) scatter plot. When the user selects a region on this scatterplot, the corresponding data are displayed. This enables users to efficiently extract data attributes. A set of data corresponding to the attributes can then be extracted. Because handwritten character datasets were used in the study, a group of data with common attributes, such as the line width of the characters and presence of ruled lines as well as the character type, were extracted. Data containing ruled line noise could be extracted with 99.40% precision and 99.96% recall.
更多查看译文
关键词
attribute extraction,data quality evaluation,data visualization tool,latent variable,multi-facet clustering variational autoencoders (MFCVAE)
AI 理解论文
溯源树
样例
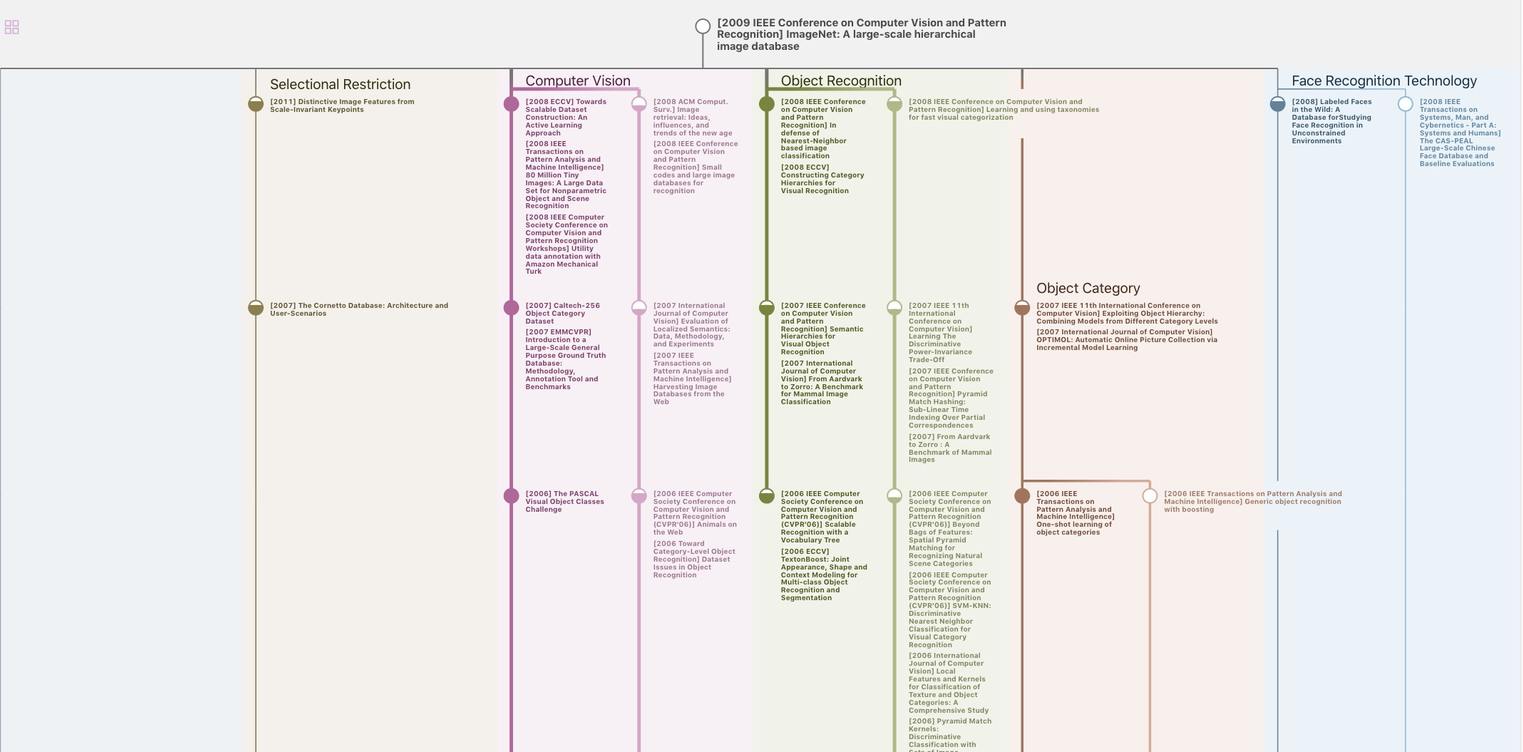
生成溯源树,研究论文发展脉络
Chat Paper
正在生成论文摘要