A Multi-Modal Hypergraph Neural Network via Parametric Filtering and Feature Sampling
IEEE Transactions on Big Data(2023)
摘要
In the real world, relationships between objects are often complex, involving multiple variables and modes. Hypergraph neural networks possess the capability to capture and represent such intricate relationships by deriving and inheriting their graph-based counterparts. Nevertheless, both graph and hypergraph neural networks suffer from the problem of over-smoothing when multiple graph convolution layers are stacked. To address this issue, this article introduces the Multi-modal Hypergraph Neural Network with Parametric Filtering and Feature Sampling (MHNet) to encode complex hypergraph features and mitigate over-smoothing. The proposed approach uses hypergraph structures to model high-order and multi-modal data correlations, a polynomial hypergraph filter to dynamically extract multi-scale node features through parametric polynomial fitting, and a feature sampling strategy to learn from sparse and labeled samples while avoiding overfitting. Experimental results on four hypergraph datasets and two multi-modal visual datasets demonstrate that the proposed MHNet outperforms state-of-the-art algorithms.
更多查看译文
关键词
Graph representation learning, hypergraph neural networks, multi-modality, over-smoothing
AI 理解论文
溯源树
样例
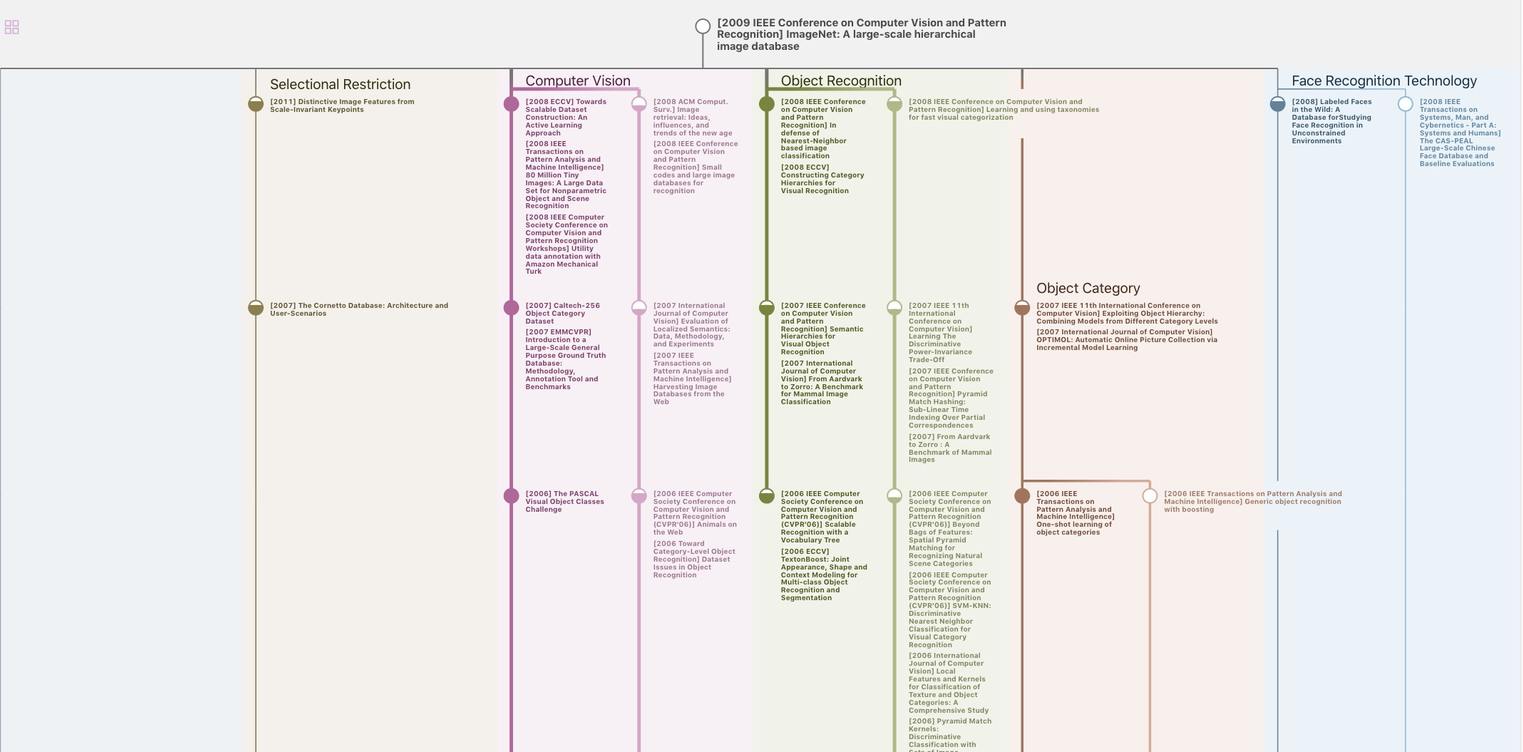
生成溯源树,研究论文发展脉络
Chat Paper
正在生成论文摘要