EdgeAdaptor: Online Configuration Adaption, Model Selection and Resource Provisioning for Edge DNN Inference Serving at Scale
IEEE Transactions on Mobile Computing(2023)
摘要
The accelerating convergence of artificial intelligence and edge computing has sparked a recent wave of interest in edge intelligence. While pilot efforts focused on edge DNN inference serving for a single user or DNN application, scaling edge DNN inference serving to multiple users and applications is however nontrivial. In this paper, we propose an online optimization framework EdgeAdaptor for multi-user and multi-application edge DNN inference serving at scale, which aims to navigate the three-way trade-off between inference accuracy, latency, and resource cost via jointly optimizing the application configuration adaption, DNN model selection and edge resource provisioning on-the-fly. The underlying long-term optimization problem is difficult since it is NP-hard and involves future uncertain information. To address these dual challenges, we fuse the power of online optimization and approximate optimization into a joint optimization framework, via i) decomposing the long-term problem into a series of single-shot fractional problems with a regularization technique, and ii) rounding the fractional solution to a near-optimal integral solution with a randomized dependent scheme. Rigorous theoretical analysis derives a parameterized competition ratio of our online algorithms, and extensive trace-driven simulations verify that its empirical value is no larger than 1.4 in typical scenarios.
更多查看译文
关键词
Edge intelligence,edge computing,DNN inference serving,online optimization
AI 理解论文
溯源树
样例
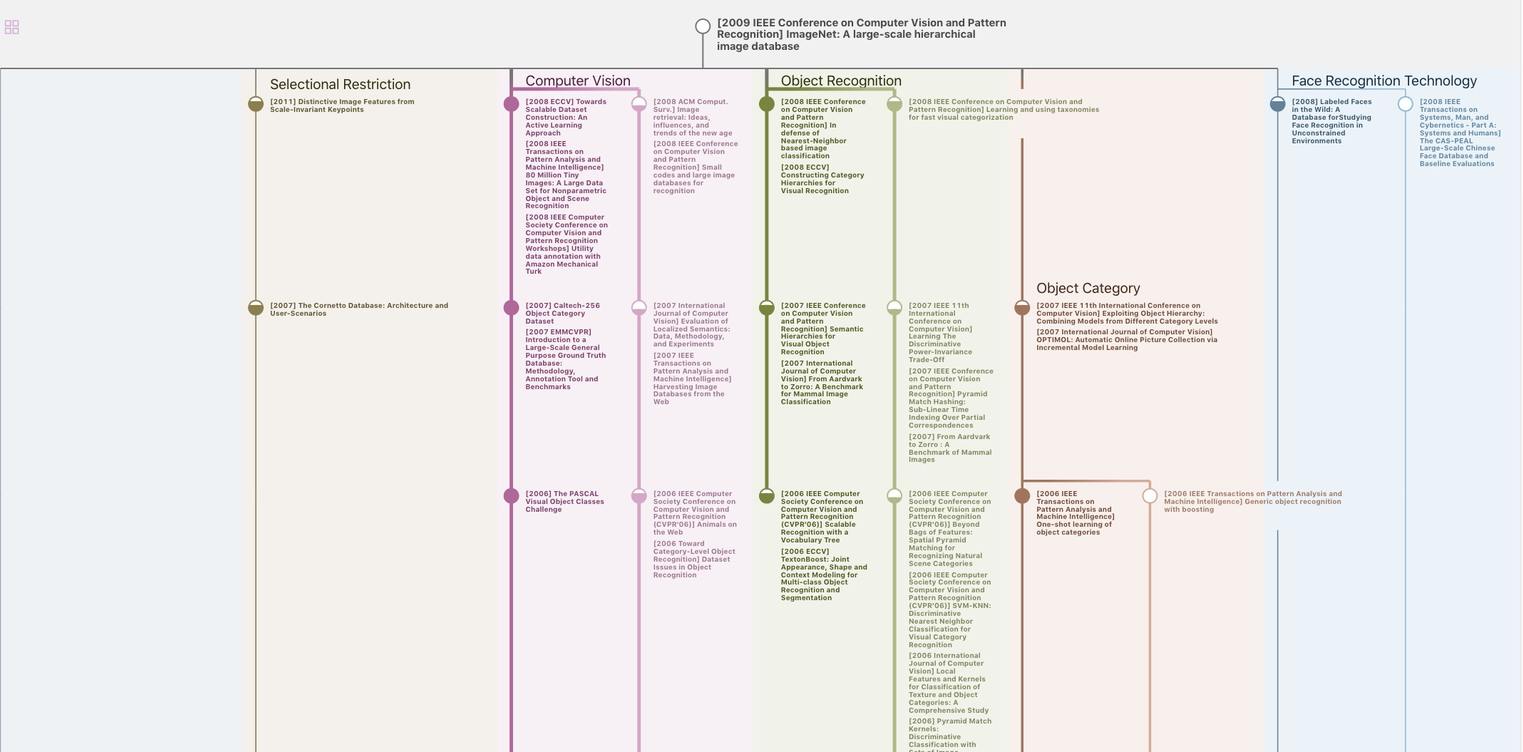
生成溯源树,研究论文发展脉络
Chat Paper
正在生成论文摘要