Low-Rank Multitask Learning based on Tensorized SVMs and LSSVMs
CoRR(2023)
摘要
Multitask learning (MTL) leverages task-relatedness to enhance performance. With the emergence of multimodal data, tasks can now be referenced by multiple indices. In this paper, we employ high-order tensors, with each mode corresponding to a task index, to naturally represent tasks referenced by multiple indices and preserve their structural relations. Based on this representation, we propose a general framework of low-rank MTL methods with tensorized support vector machines (SVMs) and least square support vector machines (LSSVMs), where the CP factorization is deployed over the coefficient tensor. Our approach allows to model the task relation through a linear combination of shared factors weighted by task-specific factors and is generalized to both classification and regression problems. Through the alternating optimization scheme and the Lagrangian function, each subproblem is transformed into a convex problem, formulated as a quadratic programming or linear system in the dual form. In contrast to previous MTL frameworks, our decision function in the dual induces a weighted kernel function with a task-coupling term characterized by the similarities of the task-specific factors, better revealing the explicit relations across tasks in MTL. Experimental results validate the effectiveness and superiority of our proposed methods compared to existing state-of-the-art approaches in MTL. The code of implementation will be available at https://github.com/liujiani0216/TSVM-MTL.
更多查看译文
关键词
tensorized svms,lssvms,learning,low-rank
AI 理解论文
溯源树
样例
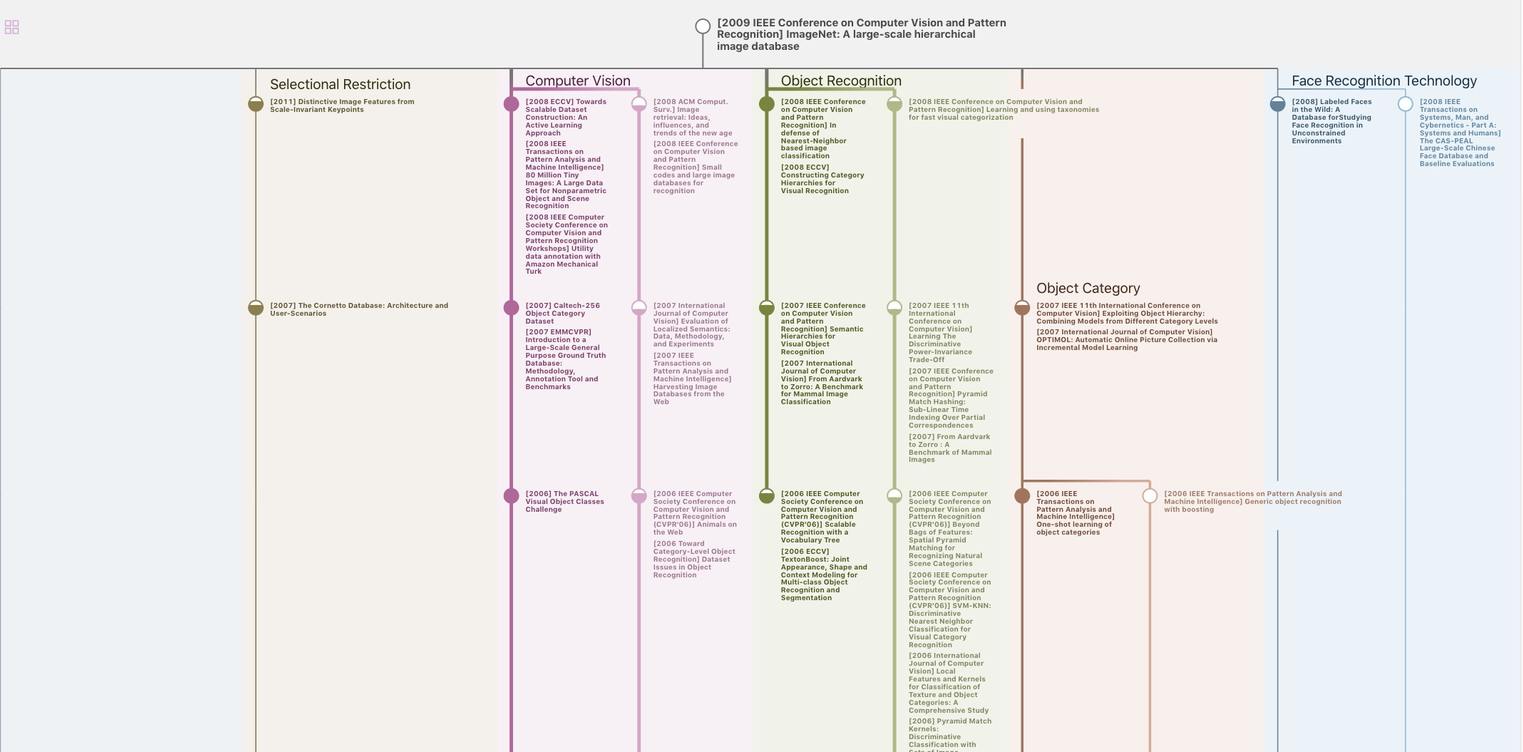
生成溯源树,研究论文发展脉络
Chat Paper
正在生成论文摘要