Providing Previously Unseen Users Fair Recommendations Using Variational Autoencoders
PROCEEDINGS OF THE 17TH ACM CONFERENCE ON RECOMMENDER SYSTEMS, RECSYS 2023(2023)
摘要
An emerging definition of fairness in machine learning requires that models are oblivious to demographic user information, e.g., a user's gender or age should not influence the model. Personalized recommender systems are particularly prone to violating this definition through their explicit user focus and user modelling. Explicit user modelling is also an aspect that makes many recommender systems incapable of providing hitherto unseen users with recommendations. We propose novel approaches for mitigating discrimination in Variational Autoencoder-based recommender systems by limiting the encoding of demographic information. The approaches are capable of, and evaluated on, providing users that are not represented in the training data with fair recommendations.
更多查看译文
关键词
fairness,variational autoencoder
AI 理解论文
溯源树
样例
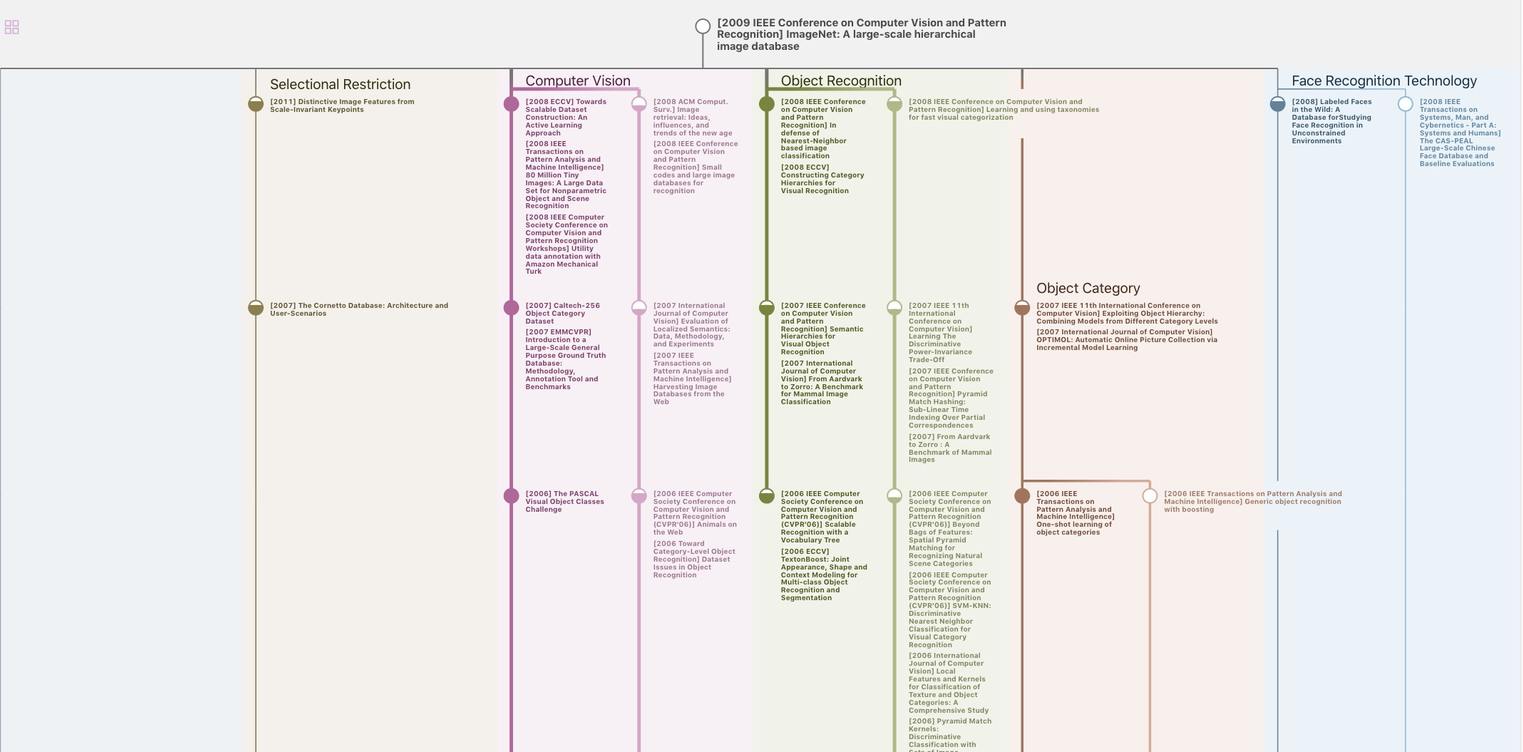
生成溯源树,研究论文发展脉络
Chat Paper
正在生成论文摘要