Speech Self-Supervised Representations Benchmarking: a Case for Larger Probing Heads
CoRR(2023)
摘要
Self-supervised learning (SSL) leverages large datasets of unlabeled speech
to reach impressive performance with reduced amounts of annotated data. The
high number of proposed approaches fostered the emergence of comprehensive
benchmarks that evaluate their performance on a set of downstream tasks
exploring various aspects of the speech signal. However, while the number of
considered tasks has been growing, most proposals rely upon a single downstream
architecture that maps the frozen SSL representations to the task labels. This
study examines how benchmarking results are affected by changes in the probing
head architecture. Interestingly, we found that altering the downstream
architecture structure leads to significant fluctuations in the performance
ranking of the evaluated models. Against common practices in speech SSL
benchmarking, we evaluate larger-capacity probing heads, showing their impact
on performance, inference costs, generalization and multi-level feature
exploitation.
更多查看译文
关键词
speech,heads,self-supervised
AI 理解论文
溯源树
样例
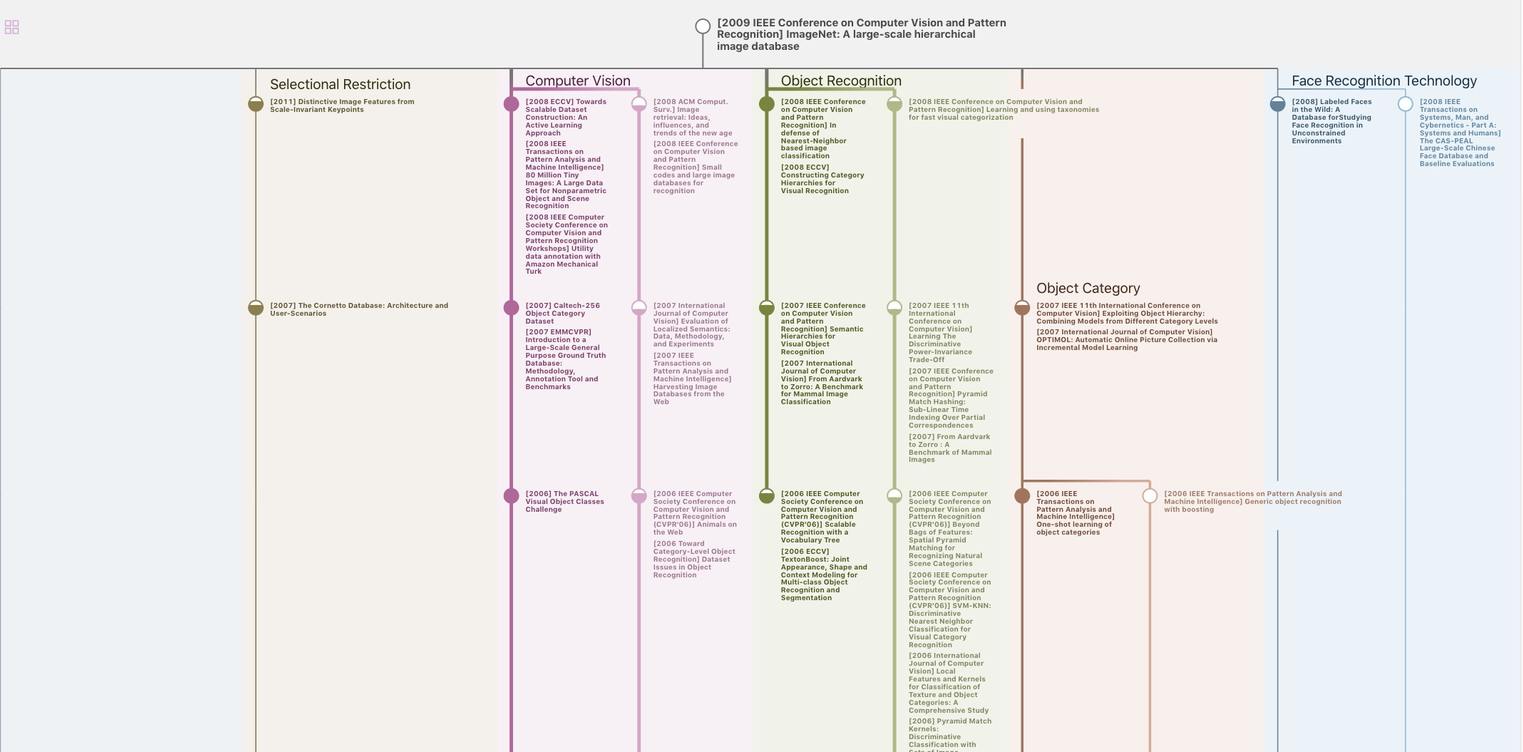
生成溯源树,研究论文发展脉络
Chat Paper
正在生成论文摘要