Towards Unified Token Learning for Vision-Language Tracking
CoRR(2023)
摘要
In this paper, we present a simple, flexible and effective vision-language (VL) tracking pipeline, termed \textbf{MMTrack}, which casts VL tracking as a token generation task. Traditional paradigms address VL tracking task indirectly with sophisticated prior designs, making them over-specialize on the features of specific architectures or mechanisms. In contrast, our proposed framework serializes language description and bounding box into a sequence of discrete tokens. In this new design paradigm, all token queries are required to perceive the desired target and directly predict spatial coordinates of the target in an auto-regressive manner. The design without other prior modules avoids multiple sub-tasks learning and hand-designed loss functions, significantly reducing the complexity of VL tracking modeling and allowing our tracker to use a simple cross-entropy loss as unified optimization objective for VL tracking task. Extensive experiments on TNL2K, LaSOT, LaSOT$_{\rm{ext}}$ and OTB99-Lang benchmarks show that our approach achieves promising results, compared to other state-of-the-arts.
更多查看译文
关键词
Vision-Language Tracking,Token Generation,Multi-Modal Modeling
AI 理解论文
溯源树
样例
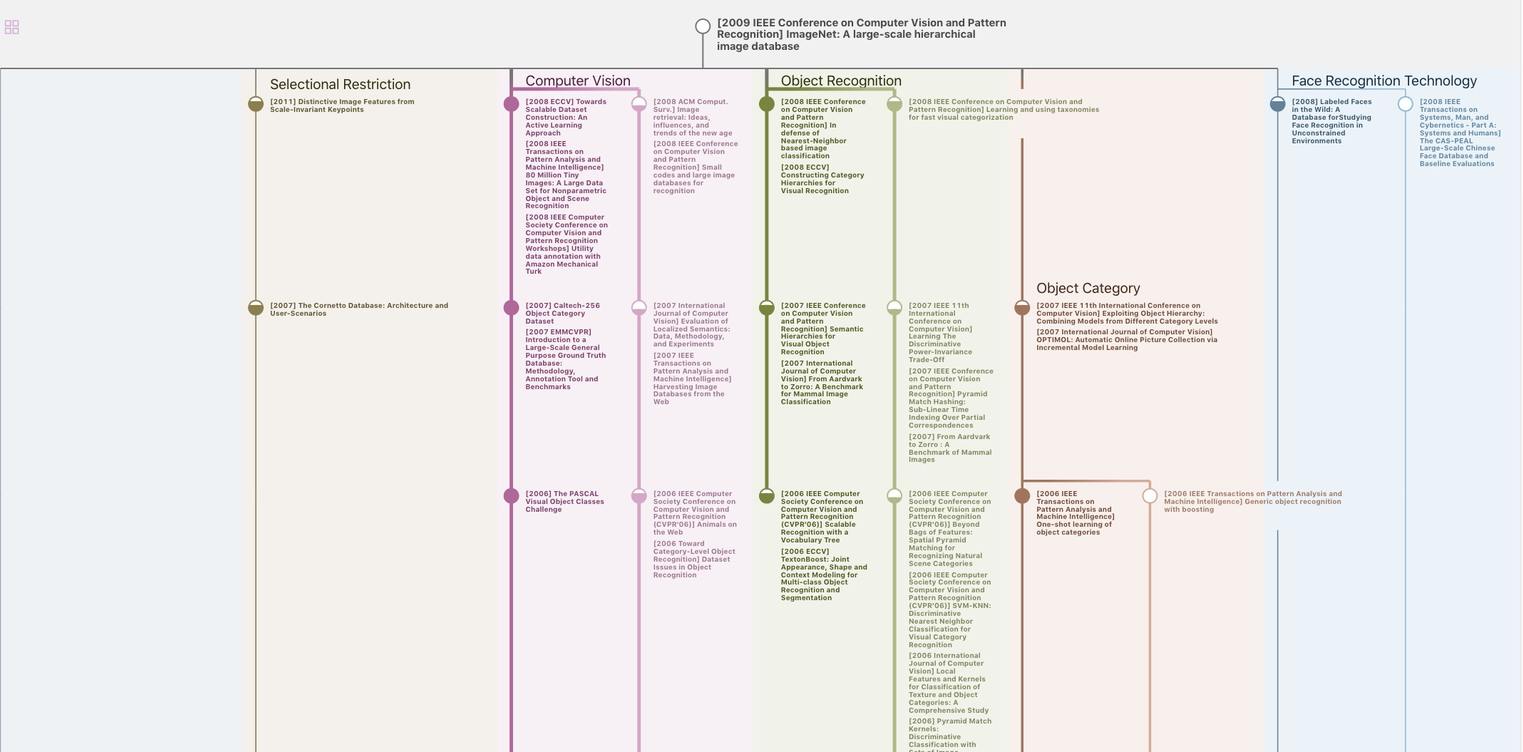
生成溯源树,研究论文发展脉络
Chat Paper
正在生成论文摘要