Deep Learning for Visual Localization and Mapping: A Survey
IEEE TRANSACTIONS ON NEURAL NETWORKS AND LEARNING SYSTEMS(2023)
摘要
Deep-learning-based localization and mapping approaches have recently emerged as a new research direction and receive significant attention from both industry and academia. Instead of creating hand-designed algorithms based on physical models or geometric theories, deep learning solutions provide an alternative to solve the problem in a data-driven way. Benefiting from the ever-increasing volumes of data and computational power on devices, these learning methods are fast evolving into a new area that shows potential to track self-motion and estimate environmental models accurately and robustly for mobile agents. In this work, we provide a comprehensive survey and propose a taxonomy for the localization and mapping methods using deep learning. This survey aims to discuss two basic questions: whether deep learning is promising for localization and mapping, and how deep learning should be applied to solve this problem. To this end, a series of localization and mapping topics are investigated, from the learning-based visual odometry and global relocalization to mapping, and simultaneous localization and mapping (SLAM). It is our hope that this survey organically weaves together the recent works in this vein from robotics, computer vision, and machine learning communities and serves as a guideline for future researchers to apply deep learning to tackle the problem of visual localization and mapping.
更多查看译文
关键词
Deep learning,global localization,visual odometry (VO),visual simultaneous localization and mapping (SLAM),visual-inertial odometry (VIO)
AI 理解论文
溯源树
样例
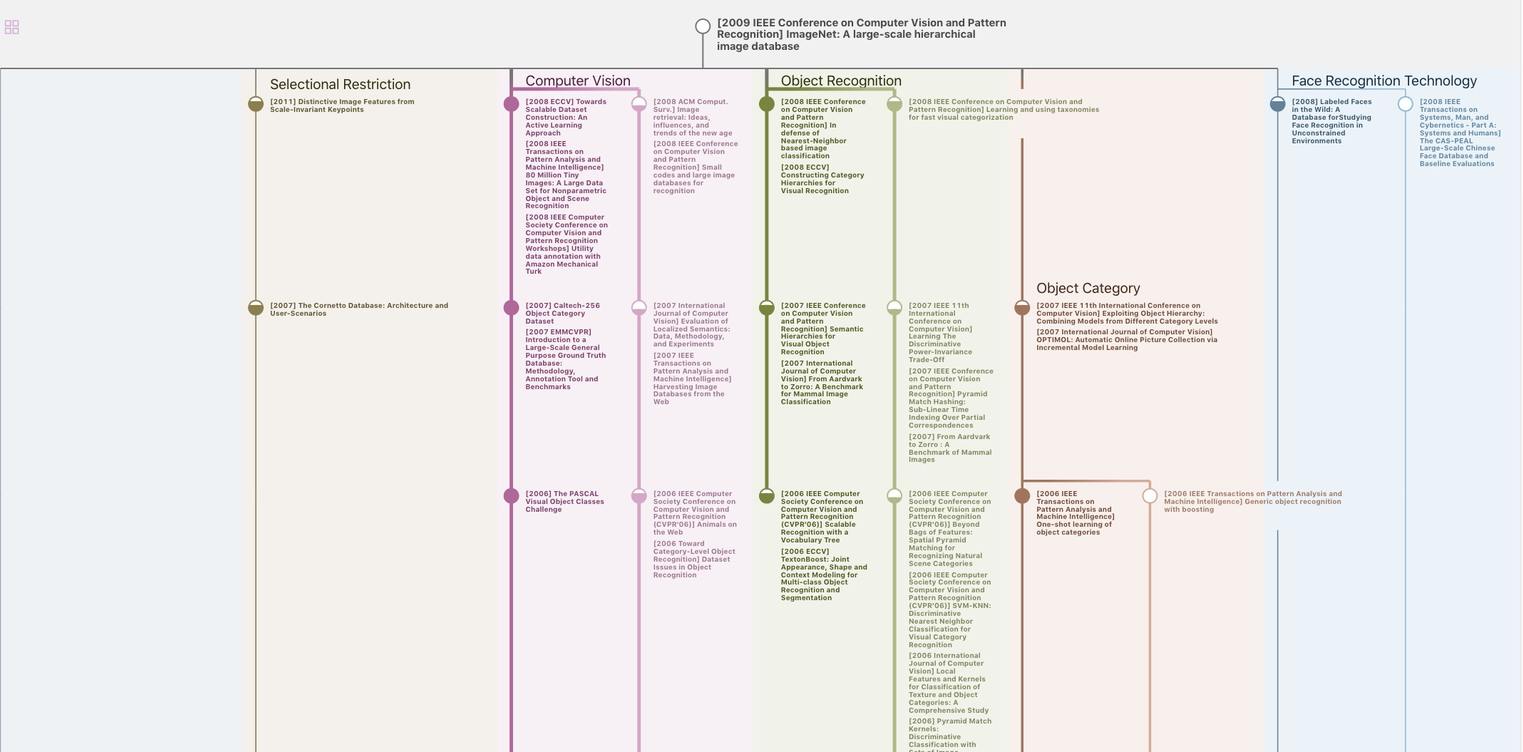
生成溯源树,研究论文发展脉络
Chat Paper
正在生成论文摘要