Time-to-Pattern: Information-Theoretic Unsupervised Learning for Scalable Time Series Summarization
CoRR(2023)
摘要
Data summarization is the process of generating interpretable and representative subsets from a dataset. Existing time series summarization approaches often search for recurring subsequences using a set of manually devised similarity functions to summarize the data. However, such approaches are fraught with limitations stemming from an exhaustive search coupled with a heuristic definition of series similarity. Such approaches affect the diversity and comprehensiveness of the generated data summaries. To mitigate these limitations, we introduce an approach to time series summarization, called Time-to-Pattern (T2P), which aims to find a set of diverse patterns that together encode the most salient information, following the notion of minimum description length. T2P is implemented as a deep generative model that learns informative embeddings of the discrete time series on a latent space specifically designed to be interpretable. Our synthetic and real-world experiments reveal that T2P discovers informative patterns, even in noisy and complex settings. Furthermore, our results also showcase the improved performance of T2P over previous work in pattern diversity and processing scalability, which conclusively demonstrate the algorithm's effectiveness for time series summarization.
更多查看译文
关键词
learning,series,time-to-pattern,information-theoretic
AI 理解论文
溯源树
样例
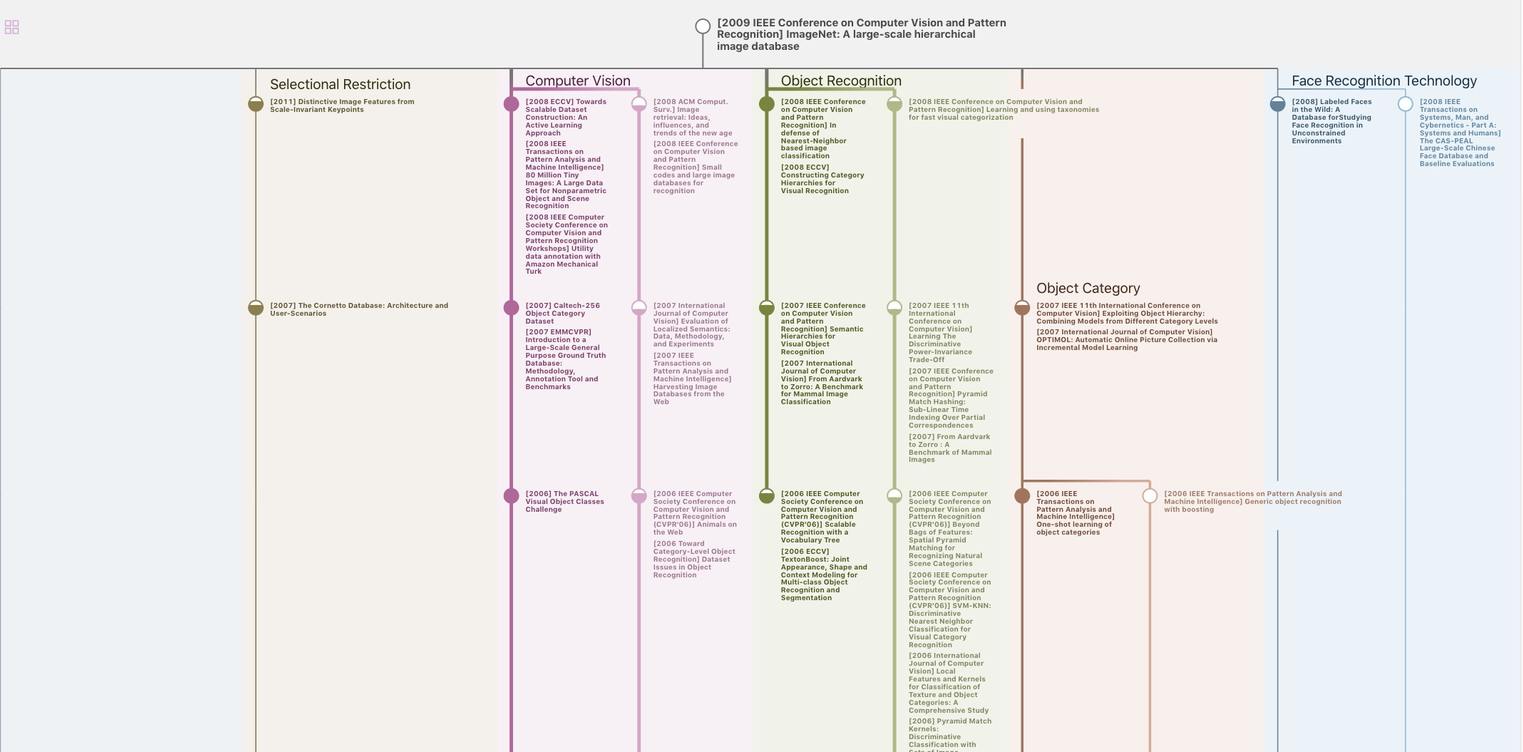
生成溯源树,研究论文发展脉络
Chat Paper
正在生成论文摘要