PAITS: Pretraining and Augmentation for Irregularly-Sampled Time Series
CoRR(2023)
摘要
Real-world time series data that commonly reflect sequential human behavior are often uniquely irregularly sampled and sparse, with highly nonuniform sampling over time and entities. Yet, commonly-used pretraining and augmentation methods for time series are not specifically designed for such scenarios. In this paper, we present PAITS (Pretraining and Augmentation for Irregularly-sampled Time Series), a framework for identifying suitable pretraining strategies for sparse and irregularly sampled time series datasets. PAITS leverages a novel combination of NLP-inspired pretraining tasks and augmentations, and a random search to identify an effective strategy for a given dataset. We demonstrate that different datasets benefit from different pretraining choices. Compared with prior methods, our approach is better able to consistently improve pretraining across multiple datasets and domains. Our code is available at \url{https://github.com/google-research/google-research/tree/master/irregular_timeseries_pretraining}.
更多查看译文
关键词
pretraining,augmentation,series,irregularly-sampled
AI 理解论文
溯源树
样例
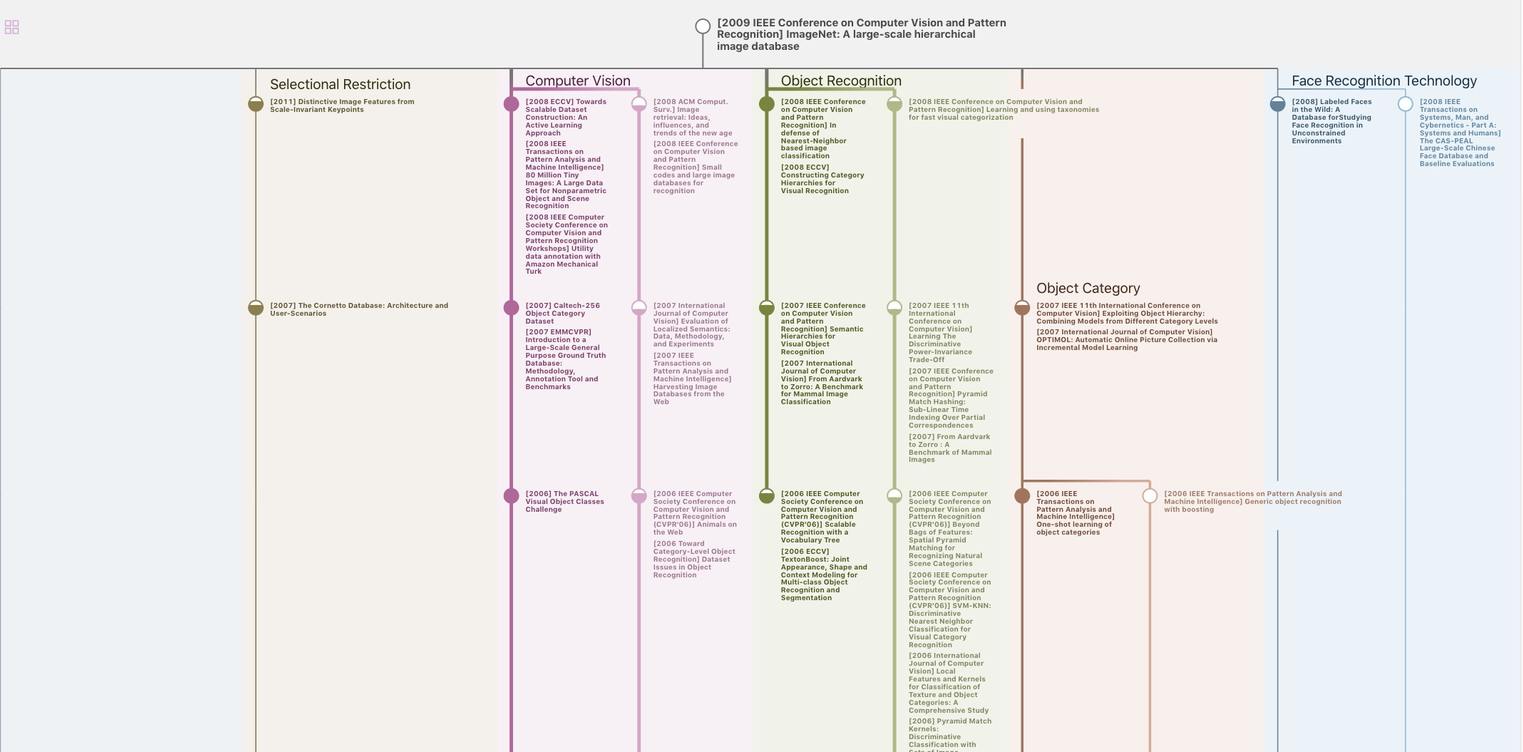
生成溯源树,研究论文发展脉络
Chat Paper
正在生成论文摘要