Learning Adversarially Robust Object Detector with Consistency Regularization in Remote Sensing Images
Remote Sensing(2023)
摘要
Object detection in remote sensing has developed rapidly and has been applied in many fields, but it is known to be vulnerable to adversarial attacks. Improving the robustness of models has become a key issue for reliable application deployment. This paper proposes a robust object detector for remote sensing images (RSIs) to mitigate the performance degradation caused by adversarial attacks. For remote sensing objects, multi-dimensional convolution is utilized to extract both specific features and consistency features from clean images and adversarial images dynamically and efficiently. This enhances the feature extraction ability and thus enriches the context information used for detection. Furthermore, regularization loss is proposed from the perspective of image distribution. This can separate consistent features from the mixed distributions for reconstruction to assure detection accuracy. Experimental results obtained using different datasets (HRSC, UCAS-AOD, and DIOR) demonstrate that the proposed method effectively improves the robustness of detectors against adversarial attacks.
更多查看译文
关键词
robust detector, adversarial example, object detection, adversarial training, remote sensing image
AI 理解论文
溯源树
样例
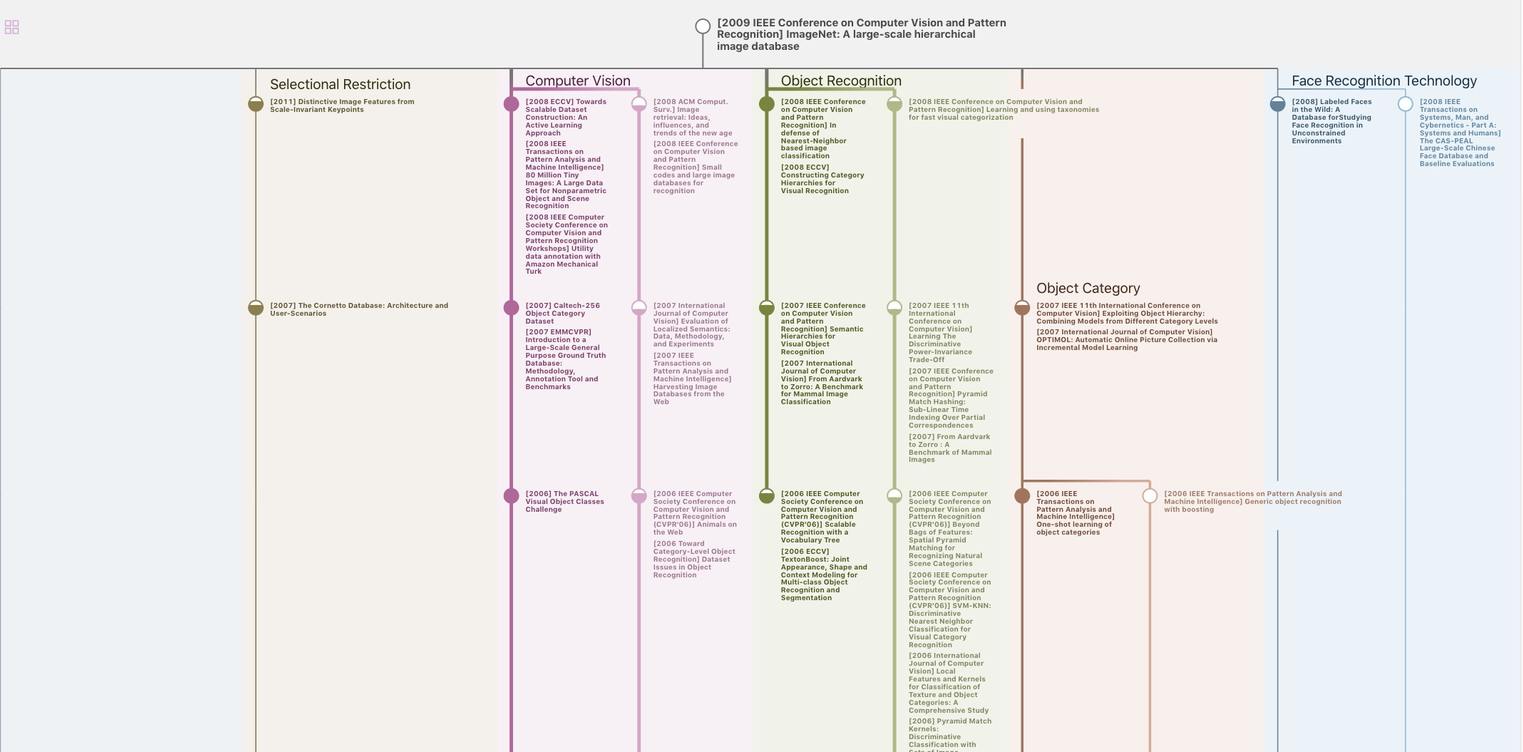
生成溯源树,研究论文发展脉络
Chat Paper
正在生成论文摘要