Dimensionality Reduction and Anomaly Detection Based on Kittler’s Taxonomy: Analyzing Water Bodies in Two Dimensional Spaces
Remote Sensing(2023)
摘要
Dimensionality reduction is one of the most used transformations of data and plays a critical role in maintaining meaningful properties while transforming data from high- to low-dimensional spaces. Previous studies, e.g., on image analysis, comparing data from these two spaces have found that, generally, any study related to anomaly detection can achieve the same or similar results when applied to both dimensional spaces. However, there have been no studies that compare differences in these spaces related to anomaly detection strategy based on Kittler’s Taxonomy (ADS-KT). This study aims to investigate the differences between both spaces when dimensionality reduction is associated with ADS-KT while analyzing a satellite image. Our methodology starts applying the pre-processing phase of the ADS-KT to create the high-dimensional space. Next, a dimensionality reduction technique generates the low-dimensional space. Then, we analyze extracted features from both spaces based on visualizations. Finally, machine-learning approaches, in accordance with the ADS-KT, produce results for both spaces. In the results section, metrics assessing transformed data present values close to zero contrasting with the high-dimensional space. Therefore, we conclude that dimensionality reduction directly impacts the application of the ADS-KT. Future work should investigate whether dimensionality reduction impacts the ADS-KT for any set of attributes.
更多查看译文
关键词
dimensionality reduction, remote sensing, Kittler's taxonomy, anomaly detection, machine learning, image analysis
AI 理解论文
溯源树
样例
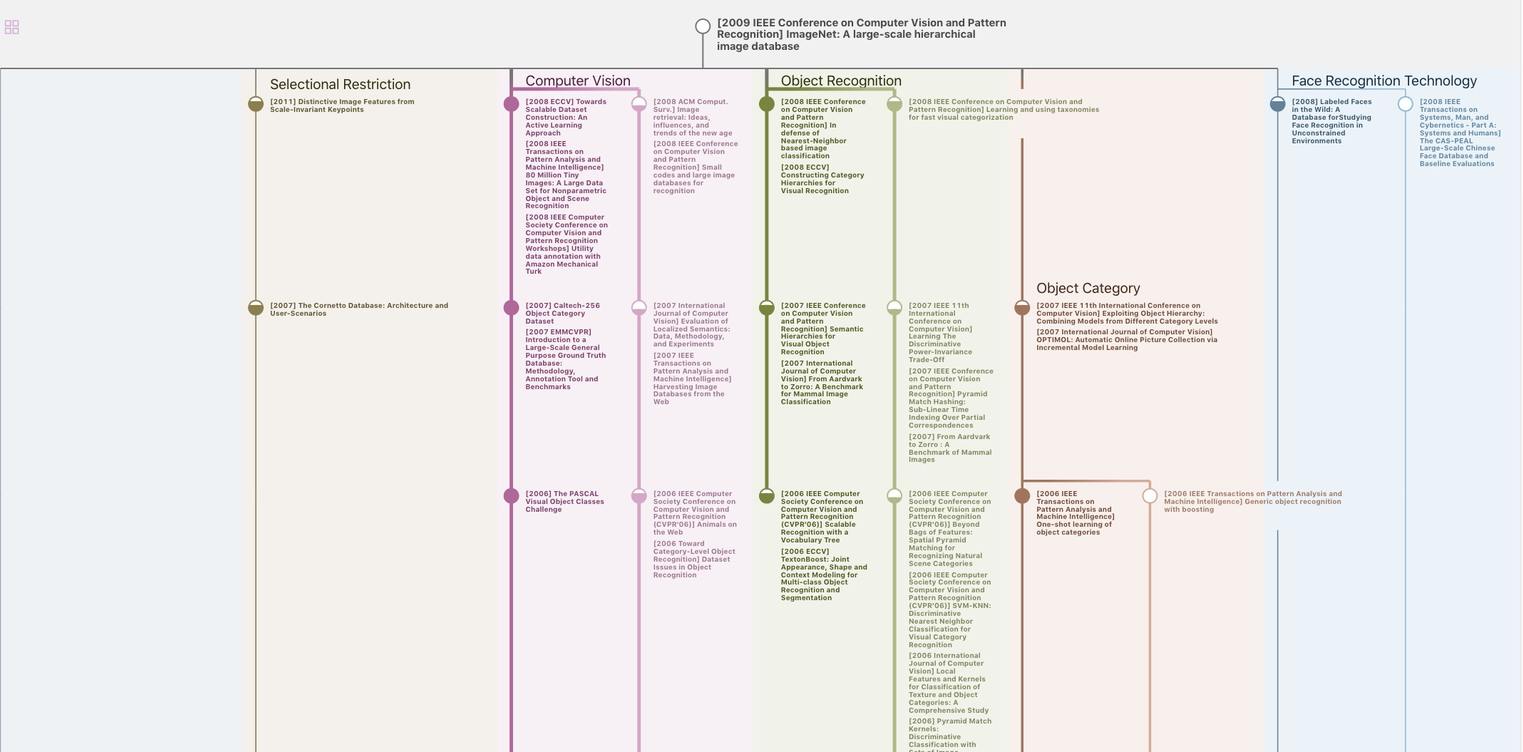
生成溯源树,研究论文发展脉络
Chat Paper
正在生成论文摘要