Prediction of multisite pain incidence in adolescence using a machine learning approach
medRxiv (Cold Spring Harbor Laboratory)(2023)
摘要
Importance Multisite pain is a major adverse health outcome in the adolescent population, affecting the daily lives of up to every third adolescent and their families.
Objective To 1) predict multisite pain incidence in the whole body and in the musculoskeletal locations in adolescents, and 2) explore the sex-specific predictors of multisite pain incidence with a novel machine learning approach.
Design A 2-year observational study (2013-2015). Three different baseline data sets were utilized to predict multisite pain incidence during the follow-up.
Setting Population-based sample of Finnish adolescents.
Participants Apparently healthy adolescents.
Exposures The first data set included 48 selected baseline variables relevant for adolescents’ health and wellbeing. Data included information on students self-reported, objectively measured, and device-based demographics, physical and psychosocial characteristics, and lifestyle factors. The second data set included nine physical fitness variables related to the Finnish national ‘Move!’ monitoring and surveillance system for health-related fitness. The third data set included all available baseline data (392 variables).
Main Outcome and Measures Onset of multisite pain (=weekly pain during the past three months manifesting in at least three sites and not related to any known disease or injury) during the 2-year follow-up in the whole body or musculoskeletal locations. Musculoskeletal pain sites included the neck/shoulder, upper extremities, chest, upper back, low back, buttocks, and lower extremities. Whole body pain sites also the head and abdominal areas. A machine learning algorithm random forest was utilized.
Results Among 410 participants (57% girls) aged on average 12.5-years (SD 1.2), 16 % of boys and 28 % of girls developed multisite pain in the whole body and 10 % and 15 % in the musculoskeletal area during follow-up.
The prediction ability of the machine learning approach with 48 predictive variables reached an AUC 0.65 at highest. With ML, a broad variety of predictors were identified, with up to 33 variables showing predictive power in girls and 13 in boys.
Conclusions and Relevance Findings highlight that rather than any isolated variable, a variety of factors pose a risk for future multisite pain. More emphasis on holistic and multidisciplinary approaches is recommended to prevent multisite pain in adolescence.
Question What is the ability of machine learning approach to predict multisite pain incidence during adolescence?
Findings With a random forest machine learning method, a broad variety of predictive physical, lifestyle and psychosocial factors were identified. Prediction ability reached AUC 0.65.
Meaning The findings highlight that predictors of multisite pain incidence in adolescence are multifaceted, although the prediction ability of machine learning remained under clinical relevance (AUC <0.7). These findings support the adoption of holistic and multidisciplinary prevention approaches for multisite pain in adolescence in the future.
### Competing Interest Statement
The authors have declared no competing interest.
### Funding Statement
This study was funded by Ella and Georg Ehrnrooth foundation. Data collection for this study was supported by the Juho Vainio Foundation (201410342) and the Finnish Ministry of Education and Culture (OKM/92/626/2013).
### Author Declarations
I confirm all relevant ethical guidelines have been followed, and any necessary IRB and/or ethics committee approvals have been obtained.
Yes
The details of the IRB/oversight body that provided approval or exemption for the research described are given below:
The study setting and measurements were approved by the Ethics Committee of the University of Jyvaskyla
I confirm that all necessary patient/participant consent has been obtained and the appropriate institutional forms have been archived, and that any patient/participant/sample identifiers included were not known to anyone (e.g., hospital staff, patients or participants themselves) outside the research group so cannot be used to identify individuals.
Yes
I understand that all clinical trials and any other prospective interventional studies must be registered with an ICMJE-approved registry, such as ClinicalTrials.gov. I confirm that any such study reported in the manuscript has been registered and the trial registration ID is provided (note: if posting a prospective study registered retrospectively, please provide a statement in the trial ID field explaining why the study was not registered in advance).
Yes
I have followed all appropriate research reporting guidelines, such as any relevant EQUATOR Network research reporting checklist(s) and other pertinent material, if applicable.
Yes
Data and utilized scripts are available upon reasonable request from IR (scripts) and THT (data).
更多查看译文
关键词
multisite pain incidence,adolescence,machine learning,prediction
AI 理解论文
溯源树
样例
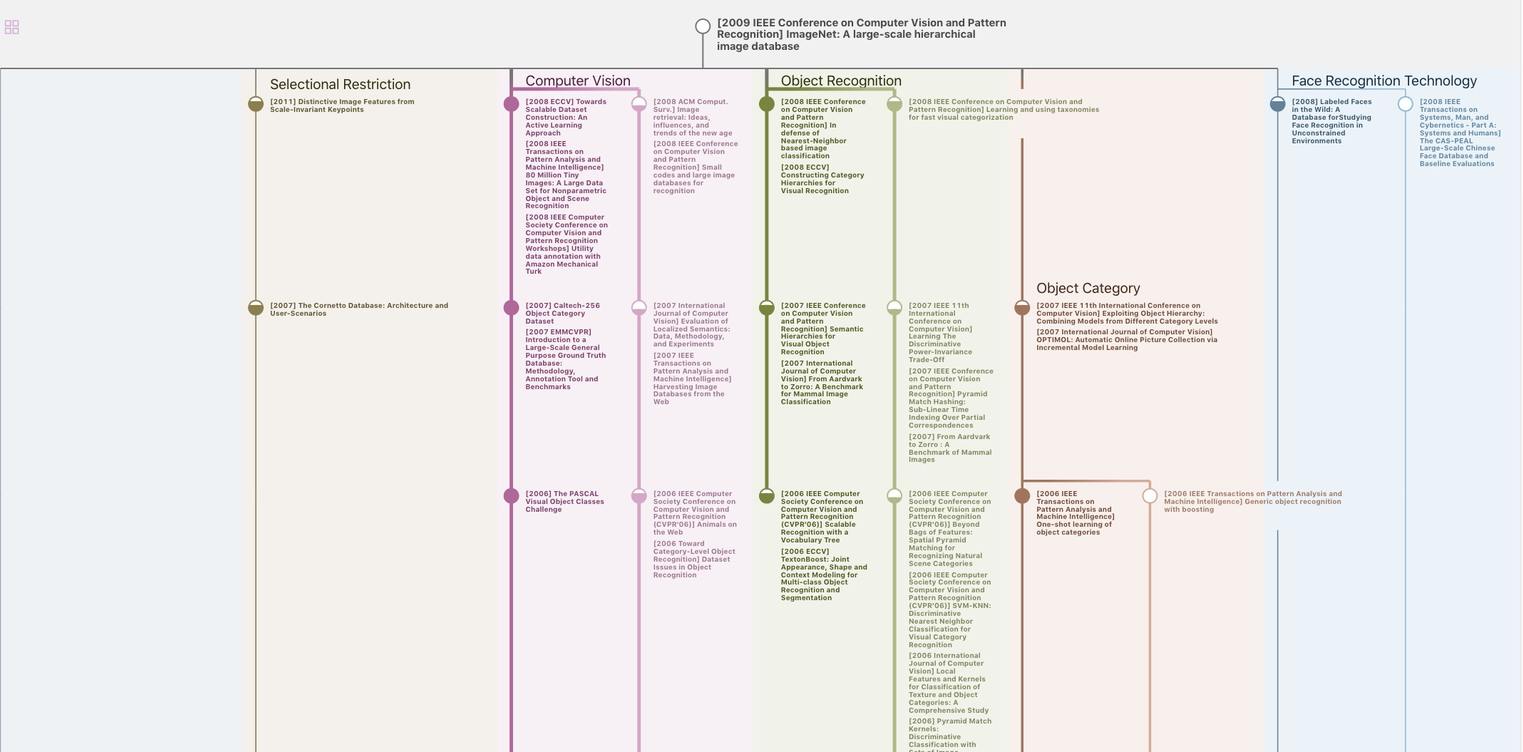
生成溯源树,研究论文发展脉络
Chat Paper
正在生成论文摘要