Simulating and reporting frequentist operating characteristics of clinical trials that borrow external information: Towards a fair comparison in case of one-arm and hybrid control two-arm trials
PHARMACEUTICAL STATISTICS(2024)
摘要
Borrowing information from historical or external data to inform inference in a current trial is an expanding field in the era of precision medicine, where trials are often performed in small patient cohorts for practical or ethical reasons. Even though methods proposed for borrowing from external data are mainly based on Bayesian approaches that incorporate external information into the prior for the current analysis, frequentist operating characteristics of the analysis strategy are often of interest. In particular, type I error rate and power at a prespecified point alternative are the focus. We propose a procedure to investigate and report the frequentist operating characteristics in this context. The approach evaluates type I error rate of the test with borrowing from external data and calibrates the test without borrowing to this type I error rate. On this basis, a fair comparison of power between the test with and without borrowing is achieved. We show that no power gains are possible in one-sided one-arm and two-arm hybrid control trials with normal endpoint, a finding proven in general before. We prove that in one-arm fixed-borrowing situations, unconditional power (i.e., when external data is random) is reduced. The Empirical Bayes power prior approach that dynamically borrows information according to the similarity of current and external data avoids the exorbitant type I error inflation occurring with fixed borrowing. In the hybrid control two-arm trial we observe power reductions as compared to the test calibrated to borrowing that increase when considering unconditional power.
更多查看译文
关键词
Bayesian dynamic borrowing of information,external information,frequentist operating characteristics,power gain,type I error inflation
AI 理解论文
溯源树
样例
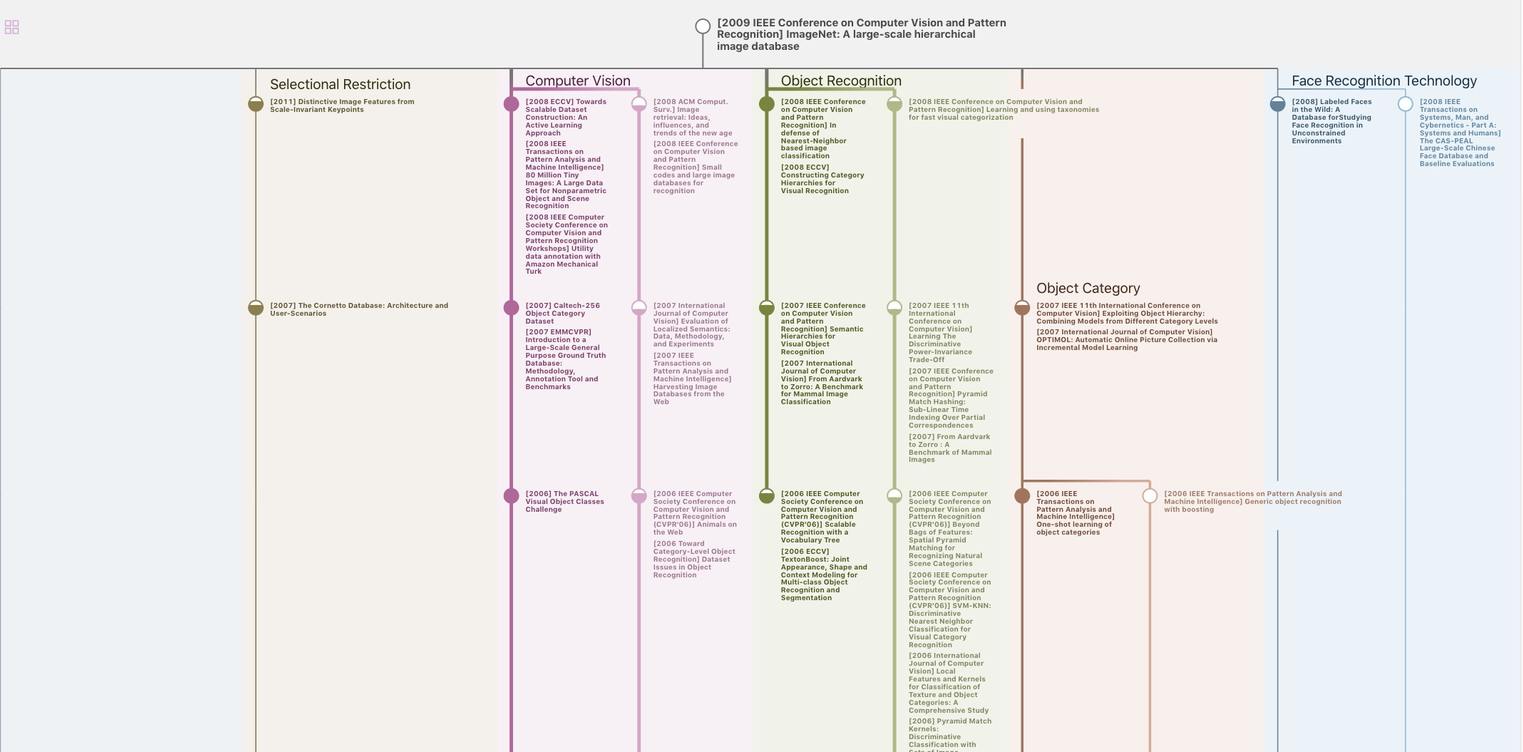
生成溯源树,研究论文发展脉络
Chat Paper
正在生成论文摘要