Fusion of Human Cognitive Knowledge and Machine Inference for Breast Cancer Detection
2023 International Conference on Advanced Robotics and Mechatronics (ICARM)(2023)
摘要
With the rapid development of artificial intelligence technology, more and more breast ultrasound CAD systems have emerged. In view of the problem that the existing breast ultrasound diagnosis system relies too much on manually design features and needs a lot of time to annotate and correct the data, the proposal of “fully automated breast ultrasound CAD” solves this problem. It reduces the workload of doctors, and the performance of the diagnosis system has been significantly improved in terms of interpretability and automation. However, the fully automated diagnosis system, which is completely dependent on the machine, also faces some challenges: on the one hand, some BI-RADS features, such as “blood vessels”, cannot be observed in the ultrasound image, resulting in the machine being unable to extract such important information from the existing image for subsequent reasoning. On the other hand, some features that are not obvious enough are difficult to be recognized by machines in ultrasonic images. However, this kind of information may play a decisive role in the final judgment of the diagnosis results. The loss of this kind of information caused by factors such as image quality in the process of machine feature extraction will affect the final diagnosis performance. Therefore, this paper proposes a new diagnostic paradigm: a semi-automatic breast ultrasound diagnosis system based on human-machine fusion. First, some features automatically extracted by machine, while the other features that are difficult to be recognized by machine are marked and scored by experienced ultrasonic doctors. Further, the features of the two parts are fused into more informative features. These features are used as medical knowledge for diagnosis to build a human-machine fusion breast ultrasound knowledge graph, then diagnose through the KG reasoning algorithm, and output the final diagnosis results. The experimental results show that the proposed method can effectively improve the performance of the fully automated diagnosis system.
更多查看译文
AI 理解论文
溯源树
样例
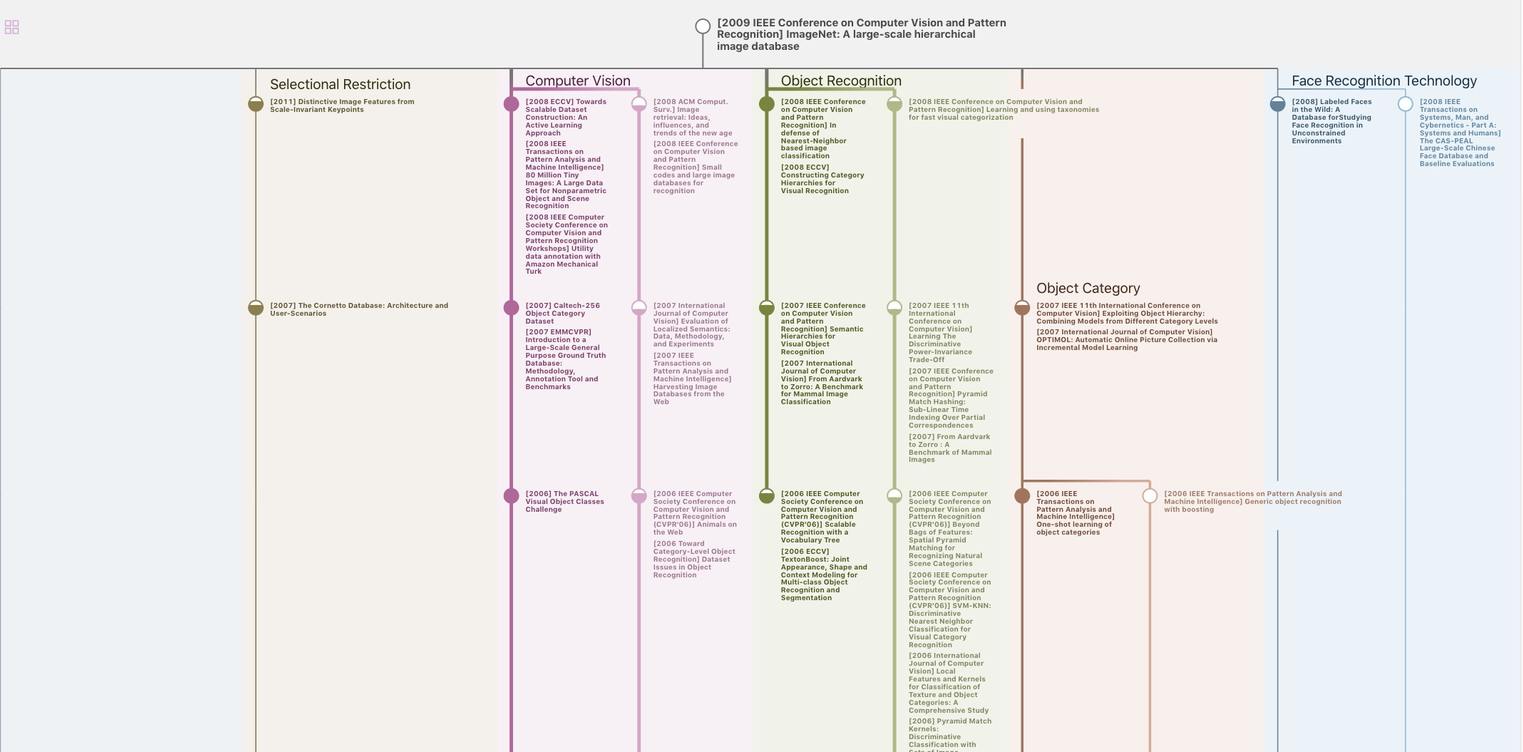
生成溯源树,研究论文发展脉络
Chat Paper
正在生成论文摘要