D2IFLN: Disentangled Domain-Invariant Feature Learning Networks for Domain Generalization
IEEE TRANSACTIONS ON COGNITIVE AND DEVELOPMENTAL SYSTEMS(2023)
摘要
Domain generalization (DG) aims to learn a model that generalizes well to an unseen test distribution. Mainstream methods follow the domain-invariant representational learning philosophy to achieve this goal. However, due to the lack of priori knowledge to determine which features are domain specific and task-independent, and which features are domain invariant and task relevant, existing methods typically learn entangled representations, limiting their capacity to generalize to the distribution-shifted target domain. To address this issue, in this article, we propose novel disentangled domain-invariant feature learning networks ((DIFLN)-I-2) to realize feature disentanglement and facilitate domain-invariant feature learning. Specifically, we introduce a semantic disentanglement network and a domain disentanglement network, disentangling the learned domain-invariant features from both domain-specific class-irrelevant features and domain-discriminative features. To avoid the semantic confusion in adversarial learning for domain-invariant feature learning, we further introduce a graph neural network to aggregate different domain semantic features during model training. Extensive experiments on three DG benchmarks show that the proposed (DIFLN)-I-2 performs better than the state of the art.
更多查看译文
关键词
Domain generalization (DG),domain-invariant feature learning,representation disentanglement
AI 理解论文
溯源树
样例
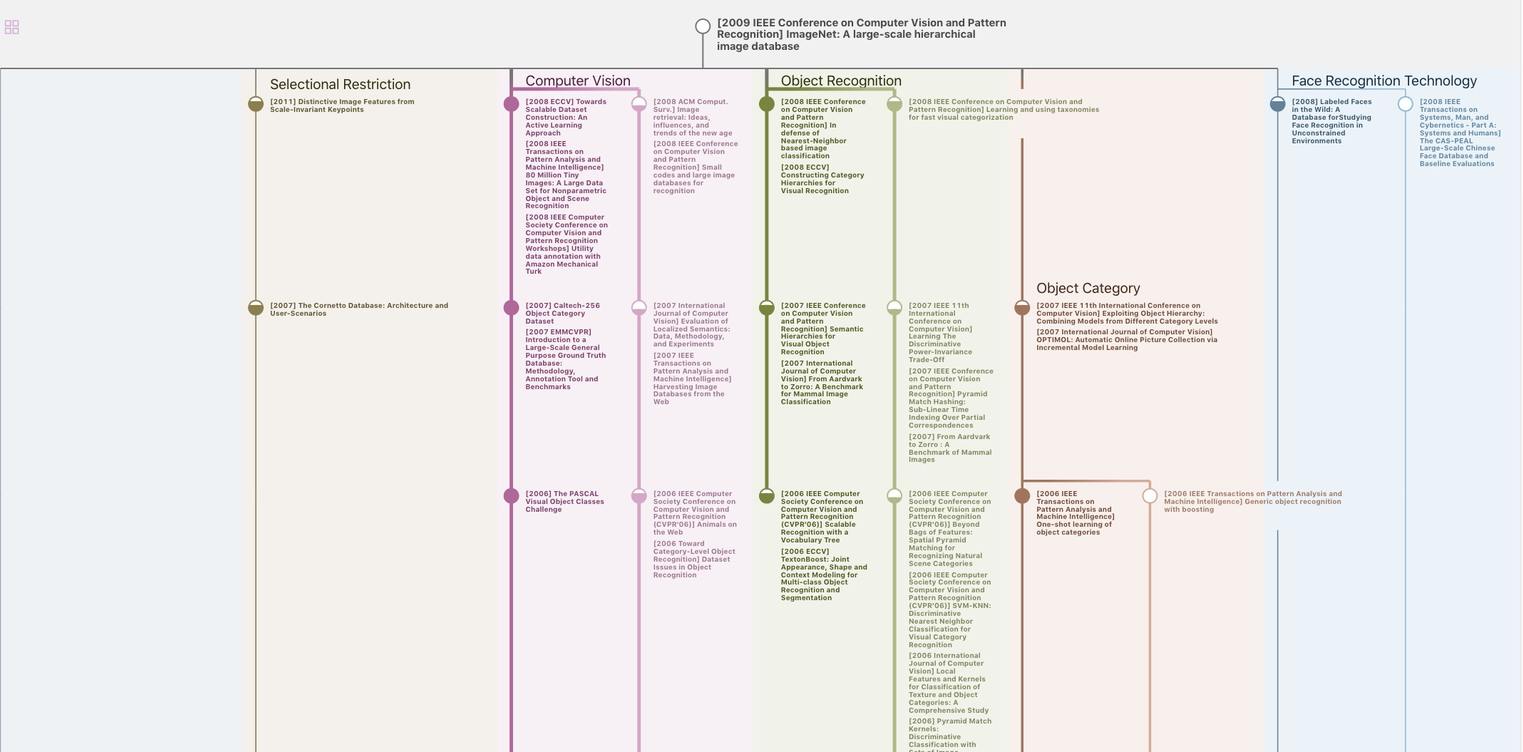
生成溯源树,研究论文发展脉络
Chat Paper
正在生成论文摘要