GatorTron: A Large Clinical Language Model to Unlock Patient Information from Unstructured Electronic Health Records
medRxiv (Cold Spring Harbor Laboratory)(2022)
摘要
ABSTRACT Objective To develop a large pretrained clinical language model from scratch using transformer architecture; systematically examine how transformer models of different sizes could help 5 clinical natural language processing (NLP) tasks at different linguistic levels. Methods We created a large corpus with >90 billion words from clinical narratives (>82 billion words), scientific literature (6 billion words), and general English text (2.5 billion words). We developed GatorTron models from scratch using the BERT architecture of different sizes including 345 million, 3.9 billion, and 8.9 billion parameters, compared GatorTron with three existing transformer models in the clinical and biomedical domain on 5 different clinical NLP tasks including clinical concept extraction, relation extraction, semantic textual similarity, natural language inference, and medical question answering, to examine how large transformer models could help clinical NLP at different linguistic levels. Results and Conclusion GatorTron scaled up transformer-based clinical language models to a size of 8.9 billion parameters and achieved state-of-the-art performance on 5 clinical NLP tasks of different linguistic levels targeting various healthcare information documented in unstructured electronic health records (EHRs). The proposed GatorTron models performed remarkably better in much complex clinical NLP tasks such as natural language inference (9.6% and 7.5% improvements) and question answering (9.5% and 7.77% improvements) compared with existing smaller clinical transformer models (i.e., BioBERT and ClinicalBERT), demonstrating the potential of large transformer-based clinical models for advanced medical artificial intelligent (AI) applications such as question answering.
更多查看译文
关键词
unlock patient information,large clinical language model
AI 理解论文
溯源树
样例
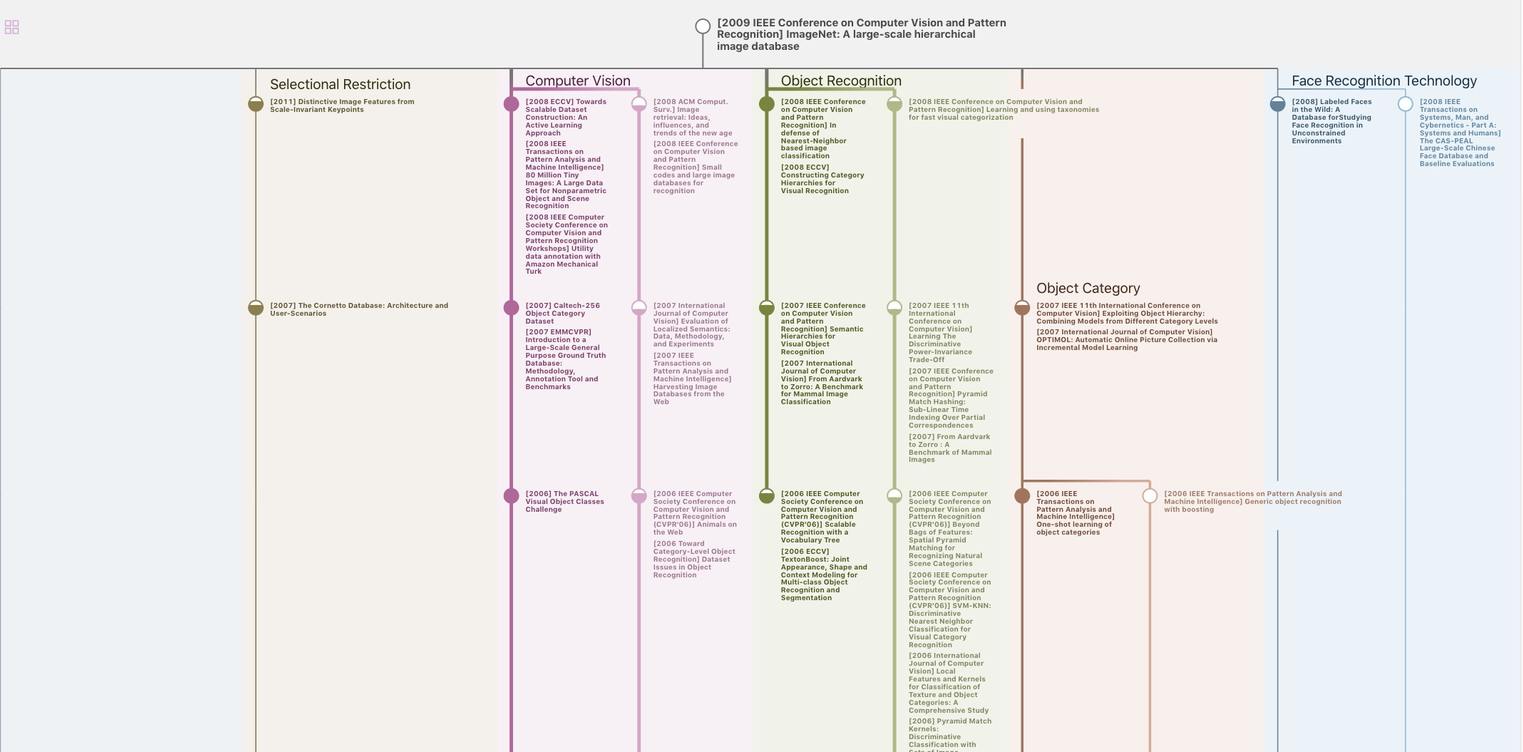
生成溯源树,研究论文发展脉络
Chat Paper
正在生成论文摘要